Machine learning model predict stress-strain plot for Marlow hyperelastic material design
Materials Today Communications(2021)
摘要
Machine Learning (ML), a subset of Artificial Intelligence (AI), is a study of computer algorithms that detect useful patterns in a multitude of experimental data sets. ML uses patterns for the prediction of values, classification of entities into specific categories, etc. Herein, we have presented a general workflow that exhibits the step-by-step process required to predict the properties of the ionic thermoplastic elastomer (Ionic-TPEs) sample. The workflow consists of five distinct steps; (a) acquisition of experimental data, (b) preprocessing of data and formation of structured data set, (c) selection and training of right ML model, (d) evaluation of the performance of the trained model, and (e) communication of the results. For this purpose, three different regression ML models have been selected and compared among them to find out the best performing ML model. A virtual stress-strain plot, created by the trained regression model for the ionic TPE sample, has been compared with the actual experimental data. Statistical parameters such as coefficient of determination (R2 score), mean absolute error (MAE), and mean squared error (MSE) has been evaluated, which suggests how well the regression models have been able to predict the values with reference to the actual experimental data. Physical properties such as tensile strength, elongation at break, hardness (Shore A), and tear strength has been predicted and compared against the actual experimental data for the particular ionic TPE sample. Furthermore, we demonstrate the Marlow hyperelastic material modeling using the virtual stress-strain plot constructed by the RF regression model.
更多查看译文
关键词
Machine learning,Thermoplastic elastomer,Regression analysis,Hyperelasticity
AI 理解论文
溯源树
样例
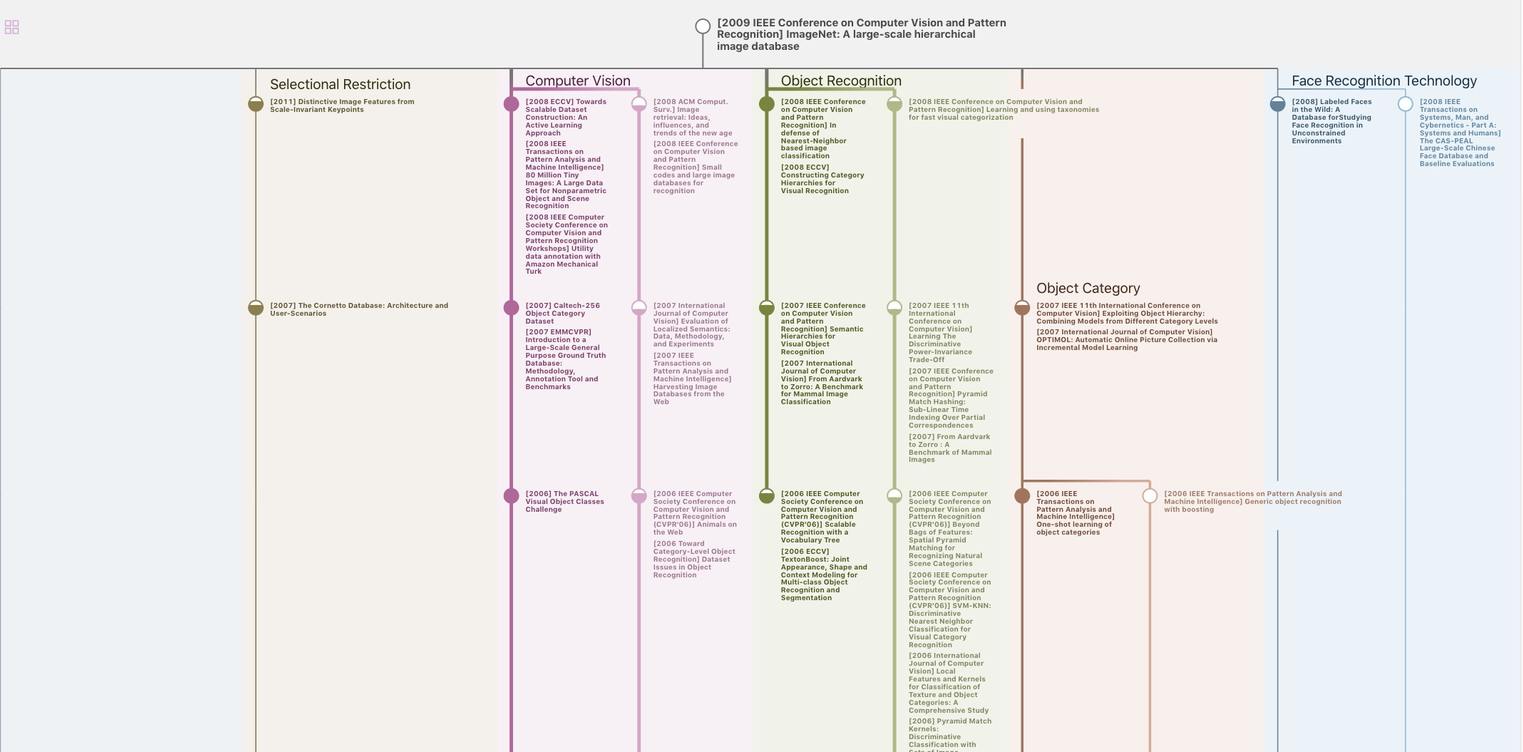
生成溯源树,研究论文发展脉络
Chat Paper
正在生成论文摘要