Large-Scale Evolutionary Multiobjective Optimization Assisted by Directed Sampling
IEEE TRANSACTIONS ON EVOLUTIONARY COMPUTATION(2021)
关键词
Optimization,Statistics,Sociology,Search problems,Convergence,Sorting,Computer science,Directed sampling (DS),evolutionary multiobjective optimization,large-scale multiobjective problems (LSMOPs),nondominated sorting,reference vectors
AI 理解论文
溯源树
样例
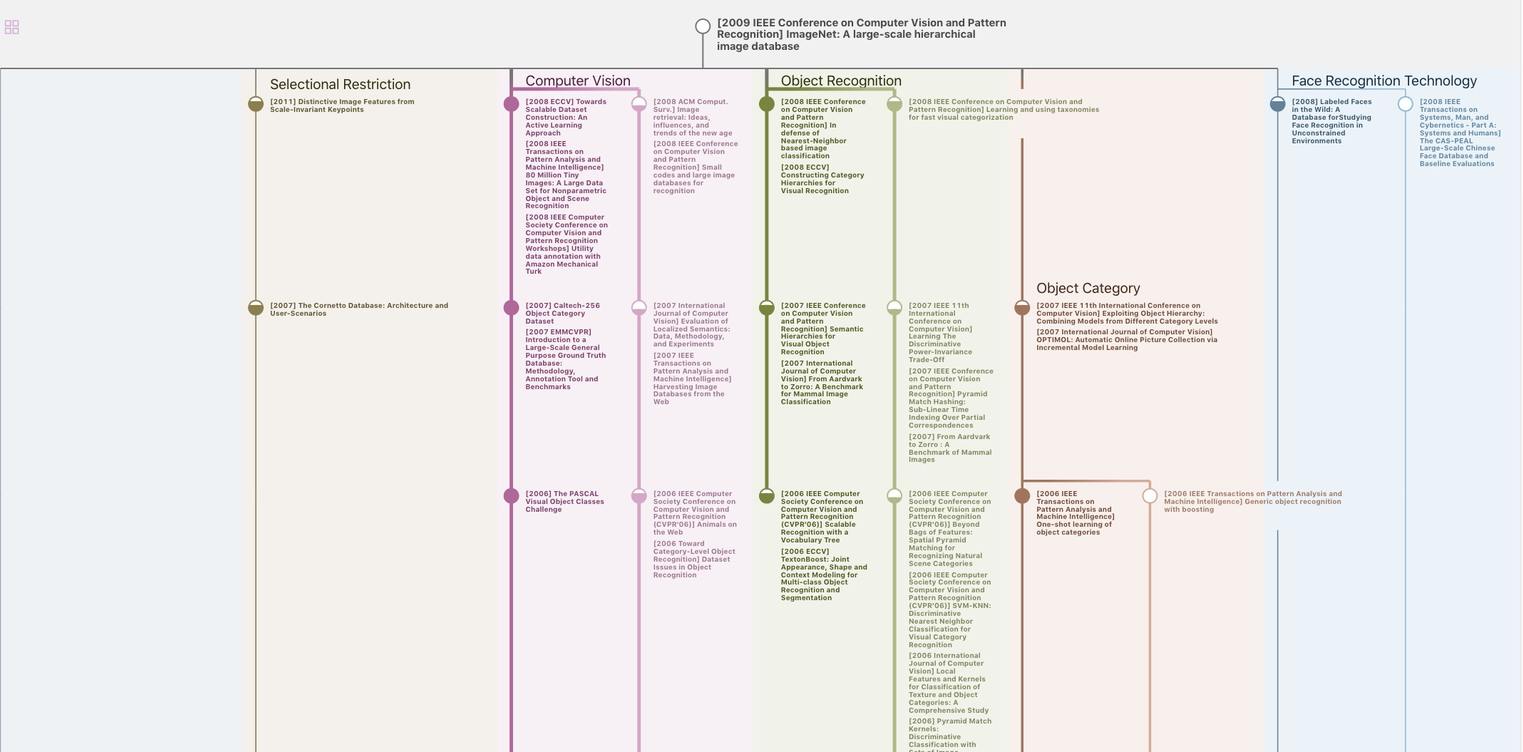
生成溯源树,研究论文发展脉络
Chat Paper
正在生成论文摘要