Robust Heart Rate Estimation with Spatial–Temporal Attention Network from Facial Videos
IEEE transactions on cognitive and developmental systems(2022)
Abstract
In order to solve the problems of highly redundant spatial information and motion noise in the heart rate (HR) estimation from facial videos based on remote photoplethysmography (rPPG), this article proposes a novel HR estimation method based on spatial–temporal attention model. First, to reduce the redundant information and strengthen the association relationships of long-range videos, the spatial–temporal facial features are extracted by the 2-D convolutional neural network (2DCNN) and 3-D convolutional neural network (3DCNN), respectively. The aggregation function is adopted to incorporate feature maps into short segment spatial–temporal feature maps. Second, the spatial–temporal strip pooling is designed in the spatial–temporal attention module to reduce head movement noises. Then, via the two-part loss function, the model can focus more on the rPPG signal rather than the interference. We conduct extensive experiments on two public data sets to verify the effectiveness of our model. The experimental results show that the proposed method achieves significantly better performances than the state-of-the-art baselines: The mean absolute error could be reduced by 11% on the PURE data set, and by 25% on the COHFACE data set.
MoreTranslated text
Key words
Feature extraction,Videos,Heart rate,Facial features,Estimation,Data mining,Signal processing,Aggregation function,remote heart rate (HR) estimation,remote photoplethysmography (rPPG),spatial-temporal attention,spatial-temporal strip pooling
AI Read Science
Must-Reading Tree
Example
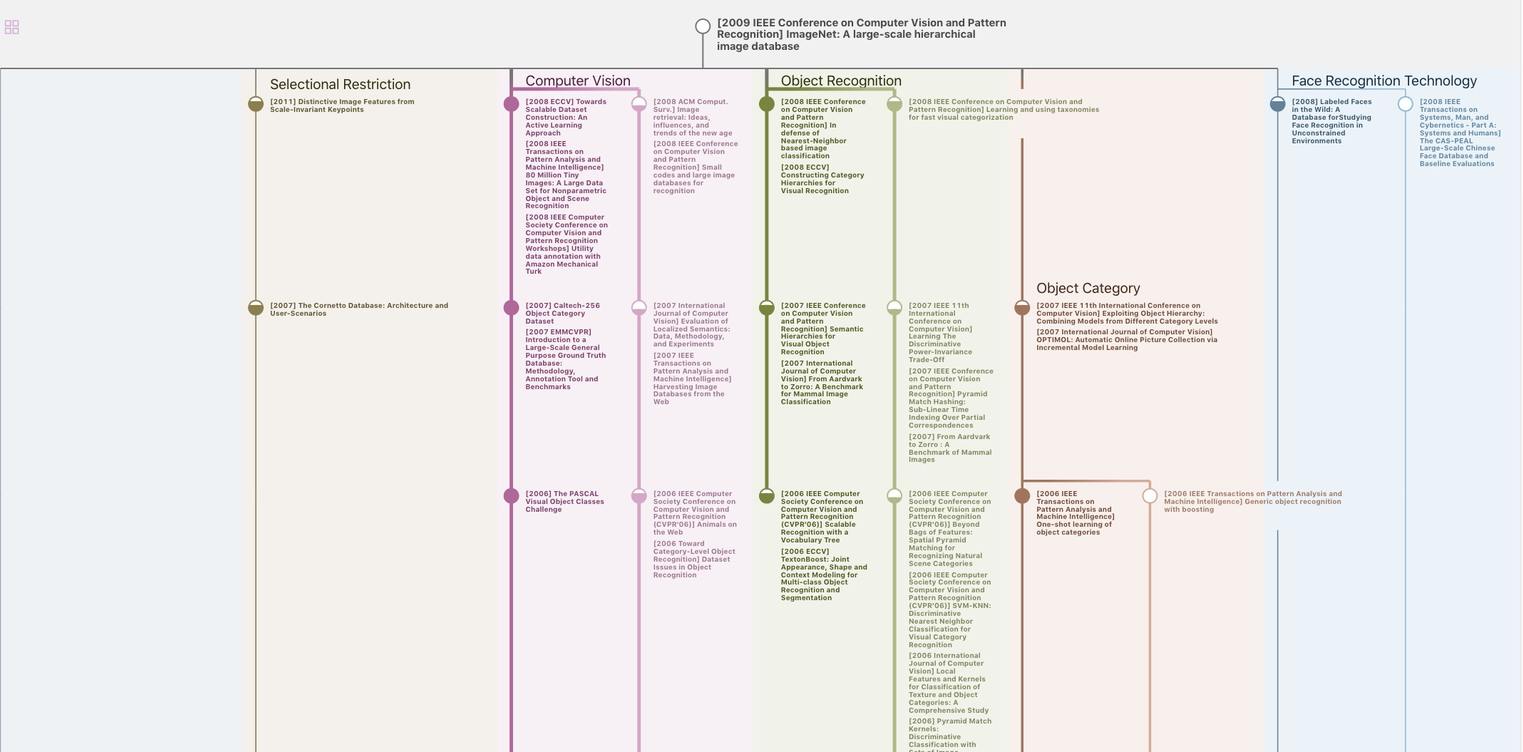
Generate MRT to find the research sequence of this paper
Chat Paper
Summary is being generated by the instructions you defined