Enhancing Fault Detection Using Multi-layer Perceptron Neural Network and Unconventional Seismic Attributes
Journal of Magnetic Resonance(2021)
摘要
Revealing the faults, one of the essential steps in seismic interpretation, provides valuable information for modeling static and dynamic characteristics of hydrocarbon reservoirs. Several methods developed for automatic fault and fracture detection, which seismic attributes integrated with artificial neural networks, and fuzzy systems are the most common ones. In this study, a 3D seismic data set of the F3 Block, offshore Netherland, was utilized for enhanced fault detection using an artificial neural network and unconventional seismic attributes integration. A steering cube was computed using a phase-based dip calculation technique to enhance seismic attributes’ accuracy and target detection capability. The fault enhancement filter, as a combination of the diffusion and median filters, and conventional attributes are modified and redefined along with the dip and azimuth information. A supervised, fully connected multi-layer perceptron neural network was constructed to integrate the previous traditional seismic attributes with optimum parameters to generate a fault probability cube. For an improved interpretation, the fault probability cube is then treated through the unconventional seismic attributes. Finally, the end product is subjected to the voxel connectivity filter to visualize the detected faults’ three-dimensional nature. Our proposed workflow results were superposed with the ones derived from the color-based inversion cube as an accurate inversion method. The proposed automatic fault extraction workflow can yield considerable savings in time and result in a highly detailed mapping of discontinuities.
更多查看译文
AI 理解论文
溯源树
样例
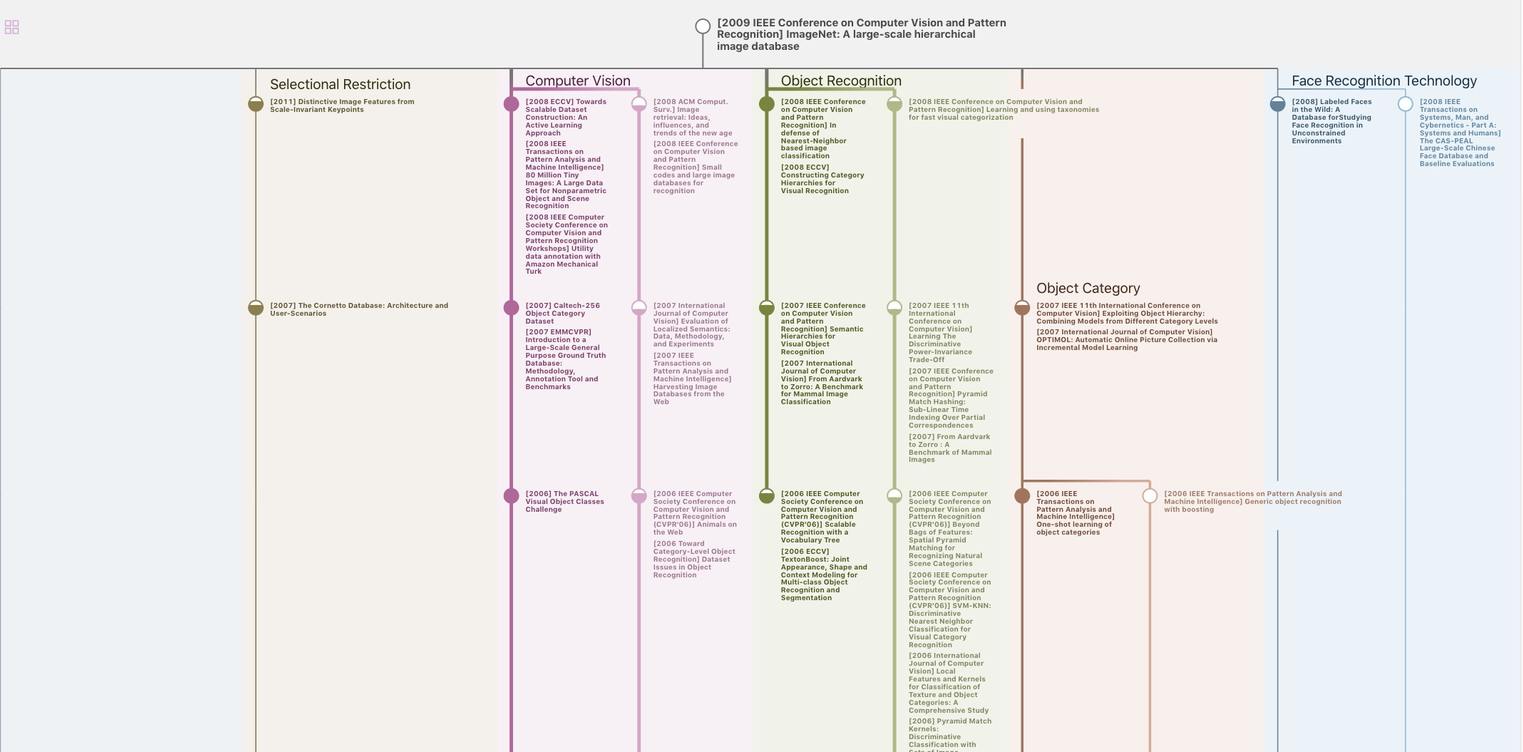
生成溯源树,研究论文发展脉络
Chat Paper
正在生成论文摘要