Temporal Attention with Domain-Specific Graph Regularization for PM 2.5 Forecasting
international conference on artificial intelligence(2020)
摘要
PM 2.5 is an essential indicator of the air quality, and accurate forecasting of PM 2.5 can help improve people’s daily life. However, the PM 2.5 concentration among different cities correlates with each other in terms of both space and time, and it is a non-trivial task for traditional physical/statistical methods to model such complex spatial-temporal correlations. Besides, various factors from environments can have an impact on PM 2.5 forecasting, thus it is necessary to consider complex information and utilize its correlations with PM 2.5 concentration. In this paper, we propose a temporal attention network with domain-specific graph regularization to improve the prediction of PM 2.5 concentration. Firstly, we consider both vertical and horizontal transportation of PM 2.5 among different cities to enhance the representation ability of graph neural networks. Secondly, we use temporal attention mechanism to capture correlations with past information. We compare our proposed methods with other state-of-the-art methods such as MLP, LSTM, GRU, GC-LSTM and PM 2.5 -GNN, and experimental results verify the effectiveness and efficiency of the proposed method.
更多查看译文
关键词
PM2.5,Graph Neural Network,Self-Attention,Recurrent Neural Network
AI 理解论文
溯源树
样例
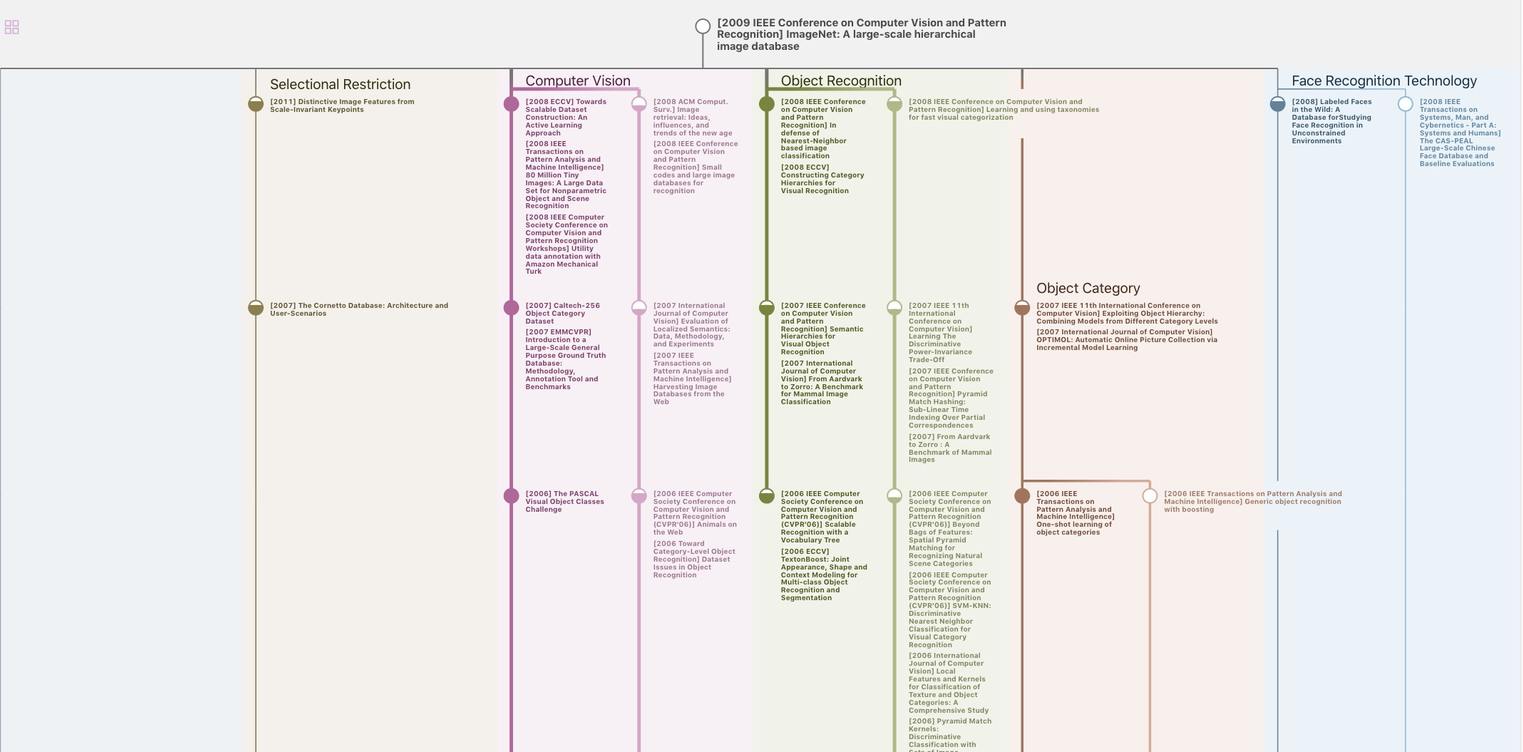
生成溯源树,研究论文发展脉络
Chat Paper
正在生成论文摘要