An application of multi-objective reinforcement learning for efficient model-free control of canals deployed with IoT networks.
J. Netw. Comput. Appl.(2021)
摘要
Canals have been widely constructed to deliver water from rich areas to poor areas to ease water shortages. Efficient controlling of canals is essential for high-performance water diversion. Many efforts have been made and lots of model-based approaches have been proposed to achieve efficient control of canals. However, when reliable physical models are unavailable or the performances of predictive models are unpromising for model-based approaches, model-free approaches (e.g., reinforcement learning, genetic algorithm) could be adopted to obtain desirable control policies. Most canal-control approaches based on reinforcement learning are developed by means of manually designed reward functions, which could face difficulties especially when multiple control objectives of canals need to be satisfied. This paper proposes a novel control approach where a Reward Network (R-Network) is developed to collaborate with the deep Q learning network. The weights of sub-rewards corresponding to multiple objectives are learned via interacting with the environment (canal simulator), and the R-Network approximates the reward function and outputs desired rewards for the reinforcement learning agent to obtain desired control policies. Extensive experiments are conducted via numerical simulation on the case study of the Chinese South to North Water Diversion Project, which is deployed with numerous sensors connected as Internet of Things. Experimental results show that our approach can achieve desirable performance improvements over the state-of-the-art model-free approaches under different test scenarios.
更多查看译文
关键词
Canal control,Model-free,Multi-objective,Reinforcement learning,South to North Water Transfer Project
AI 理解论文
溯源树
样例
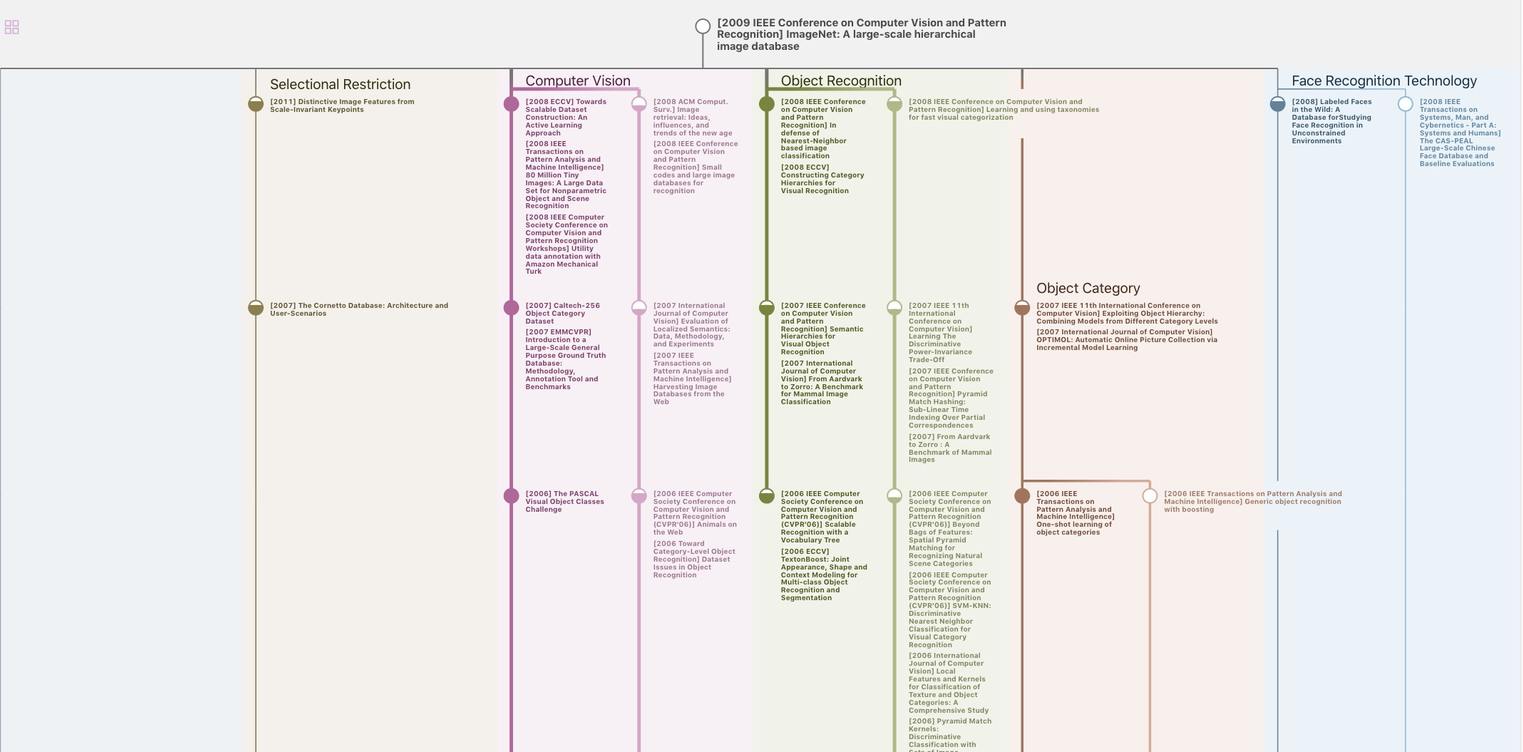
生成溯源树,研究论文发展脉络
Chat Paper
正在生成论文摘要