Prediction of Second Primary Lung Cancer Patient's Survivability Based on Improved Eigenvector Centrality-Based Feature Selection
IEEE ACCESS(2021)
摘要
Modeling of second primary lung cancer (SPLC) patients' survival prediction has important theoretical significance and practical needs. Cancer survivability prediction may provide advice for better clinical decisions and personalized medicine. The Surveillance, Epidemiology, and End Results (SEER) program provides large data sets for analysis with machine learning methods. SPLC cases are identified and labeled from the SEER database; the data set is then preprocessed with improved eigenvector centrality-based feature selection (IECFS). The IECFS method utilizes interclass and intraclass dispersions and the ranking criteria. By adjusting the value of the alpha parameter and the number of features selected, the method achieves the best performance. The experiment is divided into five folds. This method yields a prediction accuracy of 90.998% for the five-year survivability that is higher than the original classification accuracy (89.16%) and the other state-of-the-art feature selection methods. For the three-year survivability, the proposed methods yields a prediction accuracy of 83.16%, slightly outperforming all of the compared methods. The method is effective and generalizable.
更多查看译文
关键词
Cancer,Lung cancer,Feature extraction,Machine learning,Databases,Medical diagnostic imaging,Predictive models,Second primary lung cancer,cancer survival prediction,improved eigenvector centrality-based feature selection
AI 理解论文
溯源树
样例
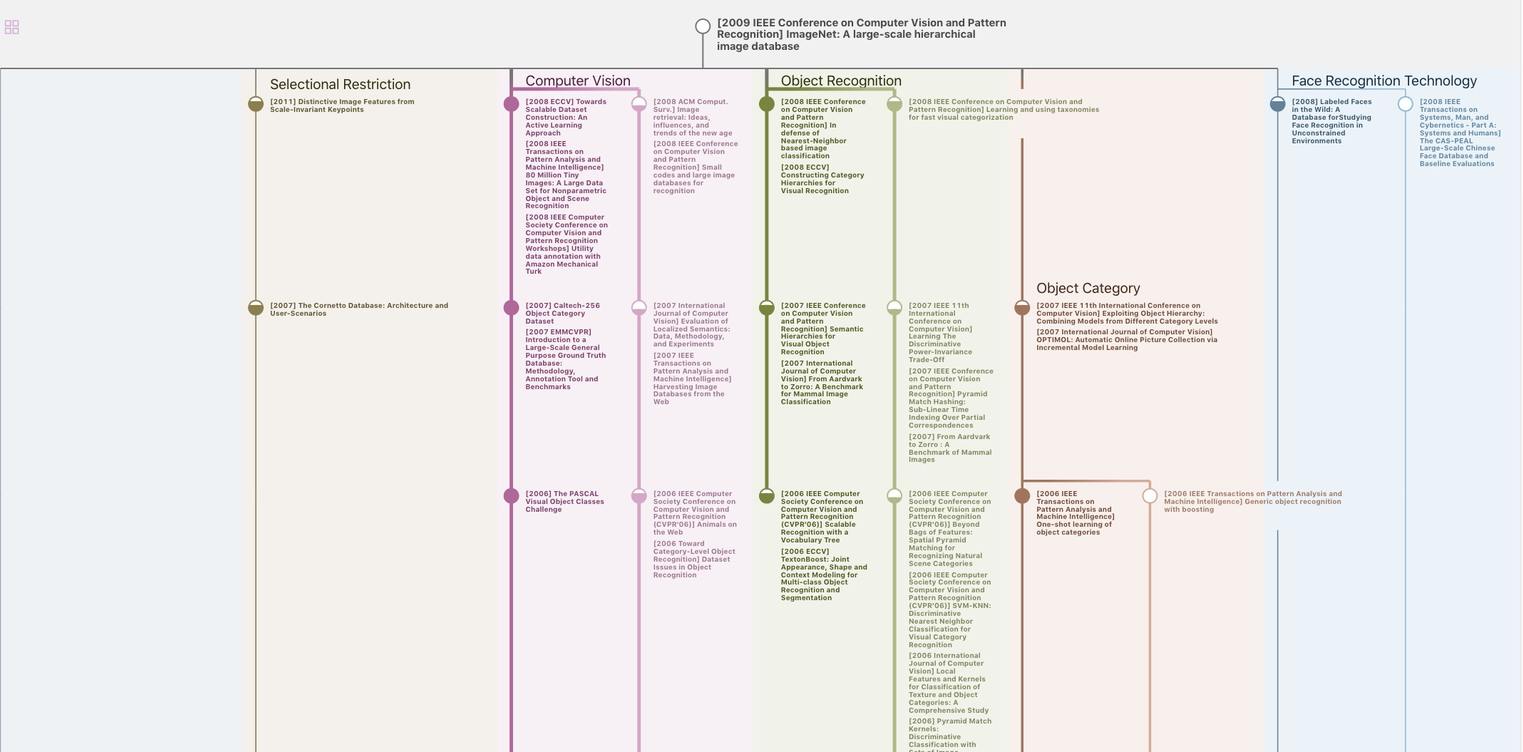
生成溯源树,研究论文发展脉络
Chat Paper
正在生成论文摘要