Tumor volume improves preoperative differentiation of prolactinomas and nonfunctioning pituitary adenomas
ENDOCRINE(2021)
摘要
Purpose Both prolactinomas and nonfunctioning adenomas (NFAs) can present with hyperprolactinemia. Distinguishing them is critical because prolactinomas are effectively managed with dopamine agonists, whereas compressive NFAs are treated surgically. Current guidelines rely only on serum prolactin (PRL) levels, which are neither sensitive nor specific enough. Recent studies suggest that accounting for tumor volume may improve diagnosis. The objective of this study is to investigate the diagnostic utility of PRL, tumor volume, and imaging features in differentiating prolactinoma and NFA. Methods Adult patients with pathologically confirmed prolactinoma ( n = 21) or NFA with hyperprolactinemia ( n = 58) between 2013 and 2020 were retrospectively identified. Diagnostic performance of clinical and imaging variables was analyzed using receiver-operating characteristic curves to calculate area under the curve (AUC). Results Tumor volume and PRL positively correlated for prolactinoma ( r = 0.4839, p = 0.0263) but not for NFA ( r = 0.0421, p = 0.7536). PRL distinguished prolactinomas from NFAs with an AUC of 0.8892 ( p < 0.0001) and optimal cut-off value of 62.45 ng/ml, yielding a sensitivity of 85.71% and specificity of 94.83%. The ratio of PRL to tumor volume had an AUC of 0.9647 ( p < 0.0001) and optimal cut-off value of 21.62 (ng/ml)/cm 3 with sensitivity of 100% and specificity of 82.76%. Binary logistic regression found that PRL was a significant positive predictor of prolactinoma diagnosis, whereas tumor volume, presence of cavernous sinus invasion, and T2 hyperintensity were significant negative predictors. The regression model had an AUC of 0.9915 ( p < 0.0001). Conclusions Consideration of tumor volume improves differentiation between prolactinomas and NFAs, which in turn leads to effective management.
更多查看译文
关键词
Prolactinoma,Nonfunctioning adenoma,Hyperprolactinemia,Stalk effect,Pituitary
AI 理解论文
溯源树
样例
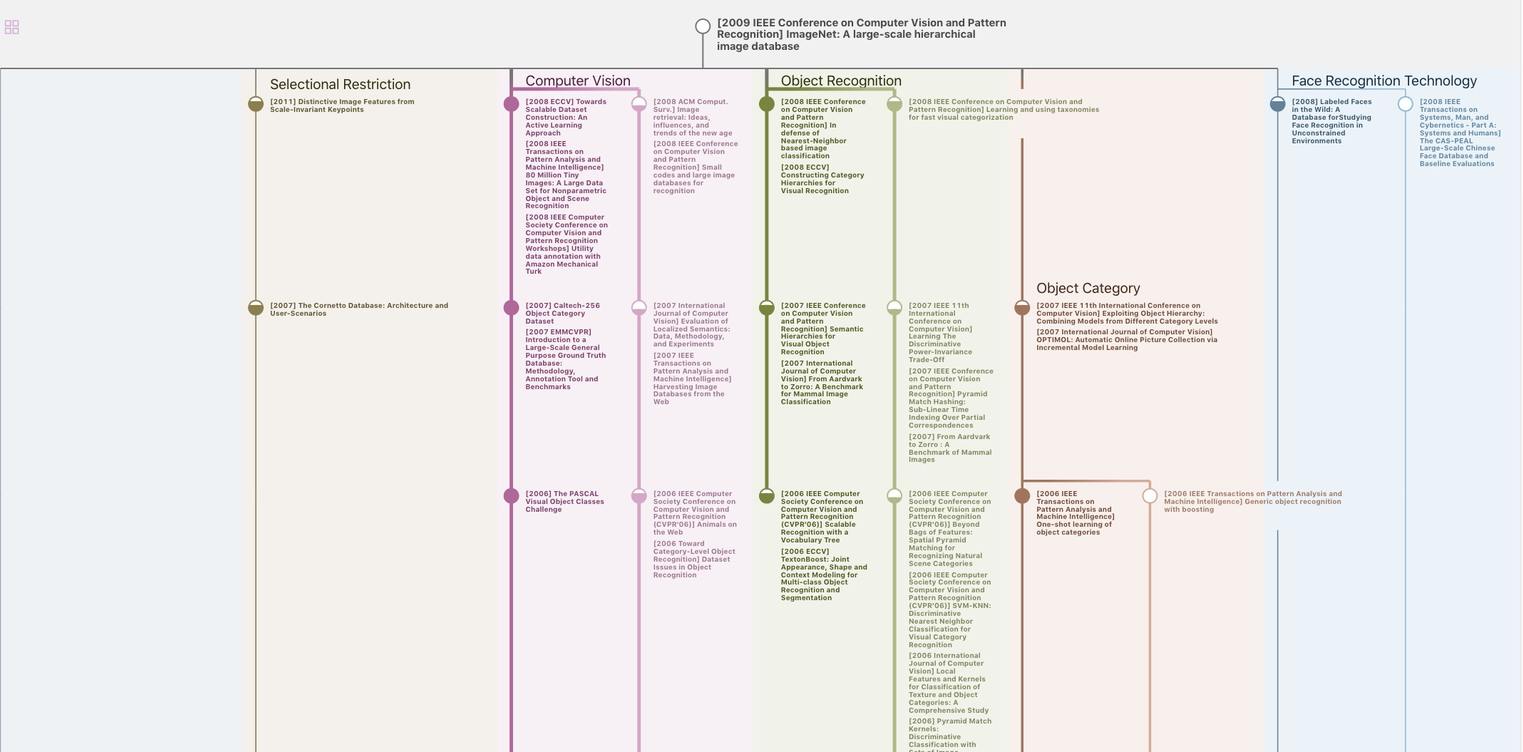
生成溯源树,研究论文发展脉络
Chat Paper
正在生成论文摘要