Selective Probabilistic Classifier Based on Hypothesis Testing
2021 9th European Workshop on Visual Information Processing (EUVIP)(2021)
摘要
In this paper, we propose a simple yet effective method to deal with the violation of the Closed-World Assumption for a classifier. Previous works tend to apply a threshold either on the classification scores or the loss function to reject the inputs that violate the assumption. However, these methods cannot achieve the low False Positive Ratio (FPR) required in safety applications. The proposed method is a rejection option based on hypothesis testing with probabilistic networks. With probabilistic networks, it is possible to estimate the distribution of outcomes instead of a single output. By utilizing Z-test over the mean and standard deviation for each class, the proposed method can estimate the statistical significance of the network certainty and reject uncertain outputs. The proposed method was experimented on with different configurations of the COCO and CIFAR datasets. The performance of the proposed method is compared with the Softmax Response, which is a known top-performing method. It is shown that the proposed method can achieve a broader range of operation and cover a lower FPR than the alternative.
更多查看译文
关键词
Selective Classifier,Probabilistic Neural Network,Statistical Analysis,Uncertainty Estimation
AI 理解论文
溯源树
样例
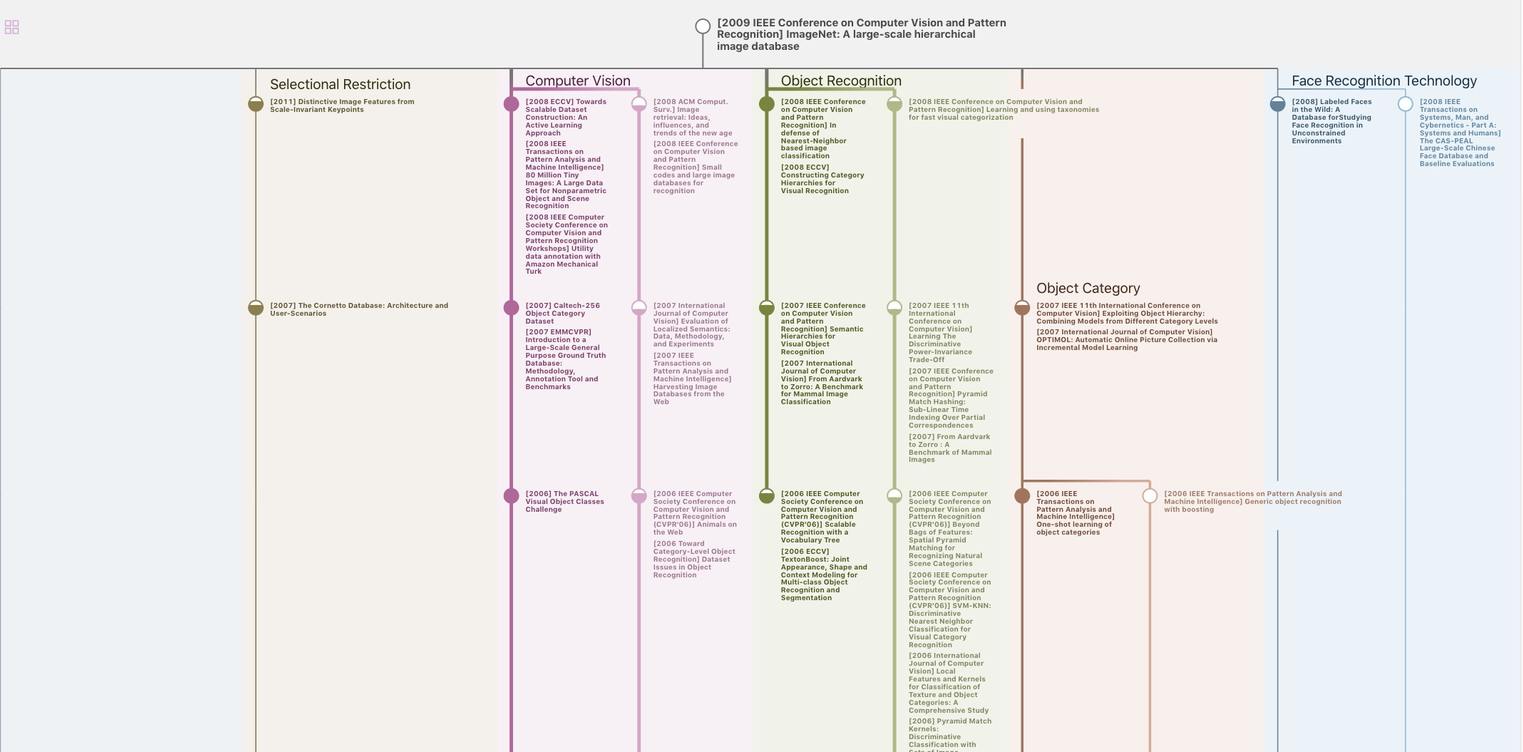
生成溯源树,研究论文发展脉络
Chat Paper
正在生成论文摘要