Deep learning of topological phase transitions from entanglement aspects: An unsupervised way
PHYSICAL REVIEW B(2021)
摘要
Machine learning techniques have been shown to be effective to recognize different phases of matter and produce phase diagrams in the parameter space of interest, while they usually require prior labeled data to perform well. Here, we propose a machine learning procedure, mainly in an unsupervised manner, which can first identify topological/nontopological phases and then refine the locations of phase boundaries. By following this proposed procedure, we expand our previous work on the one-dimensional p-wave superconductor [Tsai et al., Phys. Rev. B 102, 054512 (2020)] and further on the Su-Schrieffer-Heeger model, with an emphasis on using the quantum-entanglement-based quantities as the input features. We find that our method not only reproduces similar results to the previous work with sharp phase boundaries but importantly it also does not rely on prior knowledge of the phase space, e.g., the number of phases present. We conclude with a few remarks about its potential, limitations, and explanatory power.
更多查看译文
关键词
topological phase transitions,entanglement aspects,phase transitions,deep learning
AI 理解论文
溯源树
样例
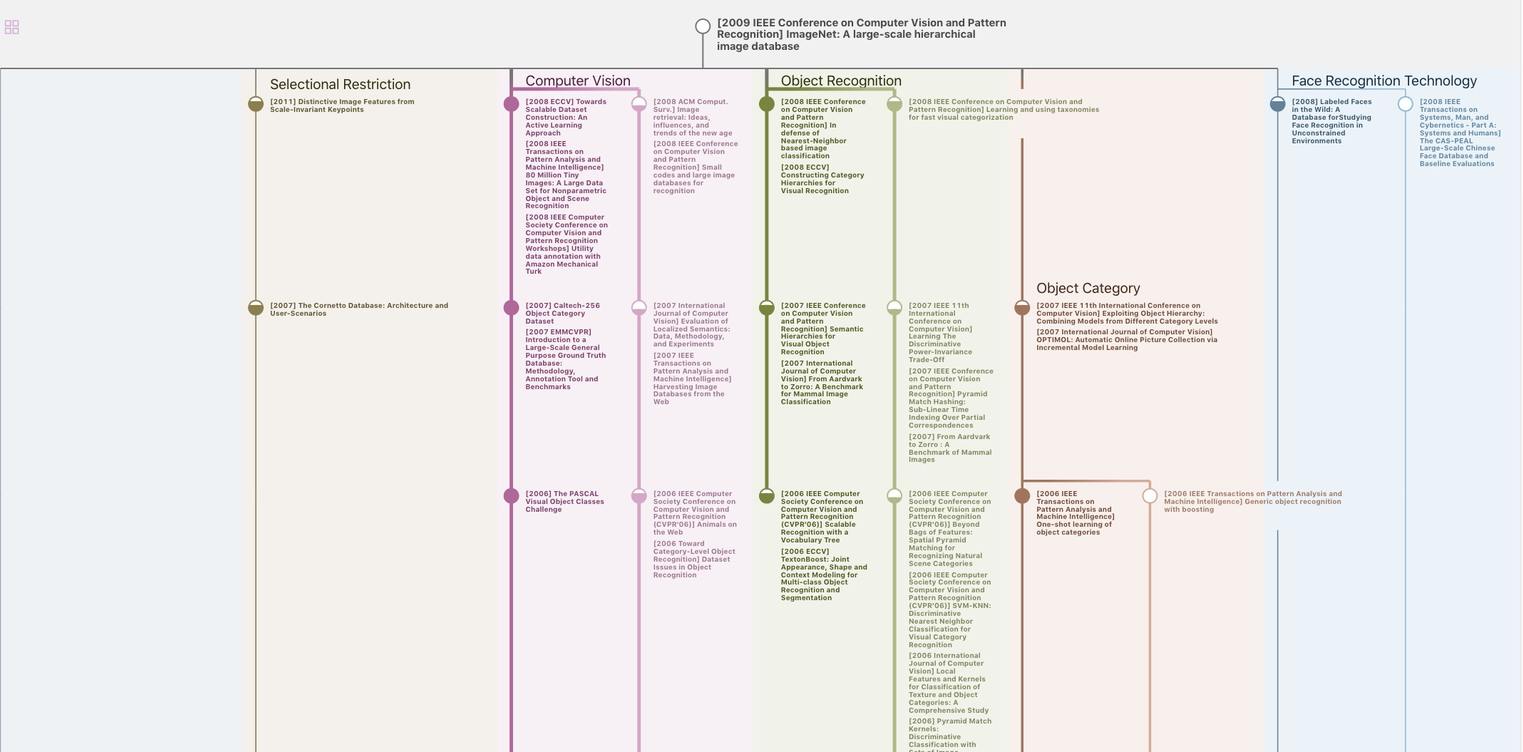
生成溯源树,研究论文发展脉络
Chat Paper
正在生成论文摘要