NON-RECURSIVE GRAPH CONVOLUTIONAL NETWORKS
2021 IEEE INTERNATIONAL CONFERENCE ON ACOUSTICS, SPEECH AND SIGNAL PROCESSING (ICASSP 2021)(2021)
摘要
Graph Convolutional Networks (GCNs) are powerful models for node representation learning tasks. However, the node representation in existing GCN models is usually generated by performing recursive neighborhood aggregation across multiple graph convolutional layers with certain sampling methods, which may lead to redundant feature mixing, needless information loss, and extensive computations. Therefore, in this paper, we propose a novel architecture named Non-Recursive Graph Convolutional Network (NRGCN) to improve both the training efficiency and the learning performance of GCNs in the context of node classification. Specifically, NRGCN proposes to represent different hops of neighbors for each node based on inner-layer aggregation and layer-independent sampling. In this way, each node can be directly represented by concatenating the information extracted independently from each hop of its neighbors thereby avoiding the recursive neighborhood expansion across layers. Moreover, the layer-independent sampling and aggregation can be precomputed before the model training, thus the training process can be accelerated considerably. Extensive experiments on benchmark datasets verify that our NRGCN outperforms the state-of-the-art GCN models, in terms of the node classification performance and reliability.
更多查看译文
关键词
graph convolutional networks, neural networks, deep learning
AI 理解论文
溯源树
样例
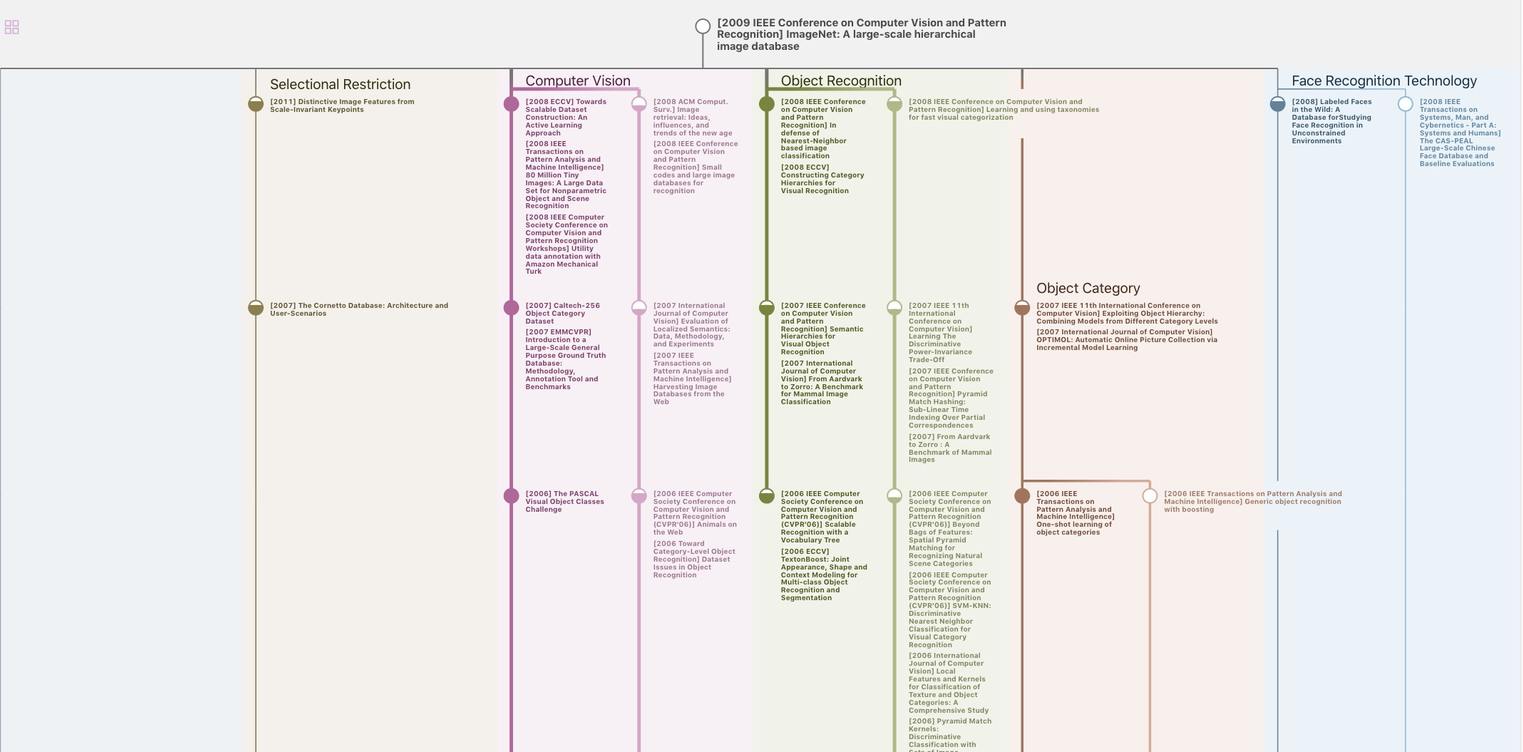
生成溯源树,研究论文发展脉络
Chat Paper
正在生成论文摘要