LatentSLAM: unsupervised multi-sensor representation learning for localization and mapping
2021 IEEE INTERNATIONAL CONFERENCE ON ROBOTICS AND AUTOMATION (ICRA 2021)(2021)
摘要
Biologically inspired algorithms for simultaneous localization and mapping (SLAM) such as RatSLAM have been shown to yield effective and robust robot navigation in both indoor and outdoor environments. One drawback however is the sensitivity to perceptual aliasing due to the template matching of low-dimensional sensory templates. In this paper, we propose an unsupervised representation learning method that yields low-dimensional latent state descriptors that can be used for RatSLAM. Our method is sensor agnostic and can be applied to any sensor modality, as we illustrate for camera images, radar range-doppler maps and lidar scans. We also show how combining multiple sensors can increase the robustness, by reducing the number of false matches. We evaluate on a dataset captured with a mobile robot navigating in a warehouse-like environment, moving through different aisles with similar appearance, making it hard for the SLAM algorithms to disambiguate locations.
更多查看译文
关键词
false matches,mobile robot navigating,warehouse-like environment,SLAM algorithms,unsupervised multisensor representation,biologically inspired algorithms,RatSLAM,effective robot navigation,robust robot navigation,indoor environments,outdoor environments,perceptual aliasing,template matching,low-dimensional sensory templates,unsupervised representation learning method,yields low-dimensional latent state descriptors,sensor modality,camera images,radar range-doppler maps,lidar scans,multiple sensors
AI 理解论文
溯源树
样例
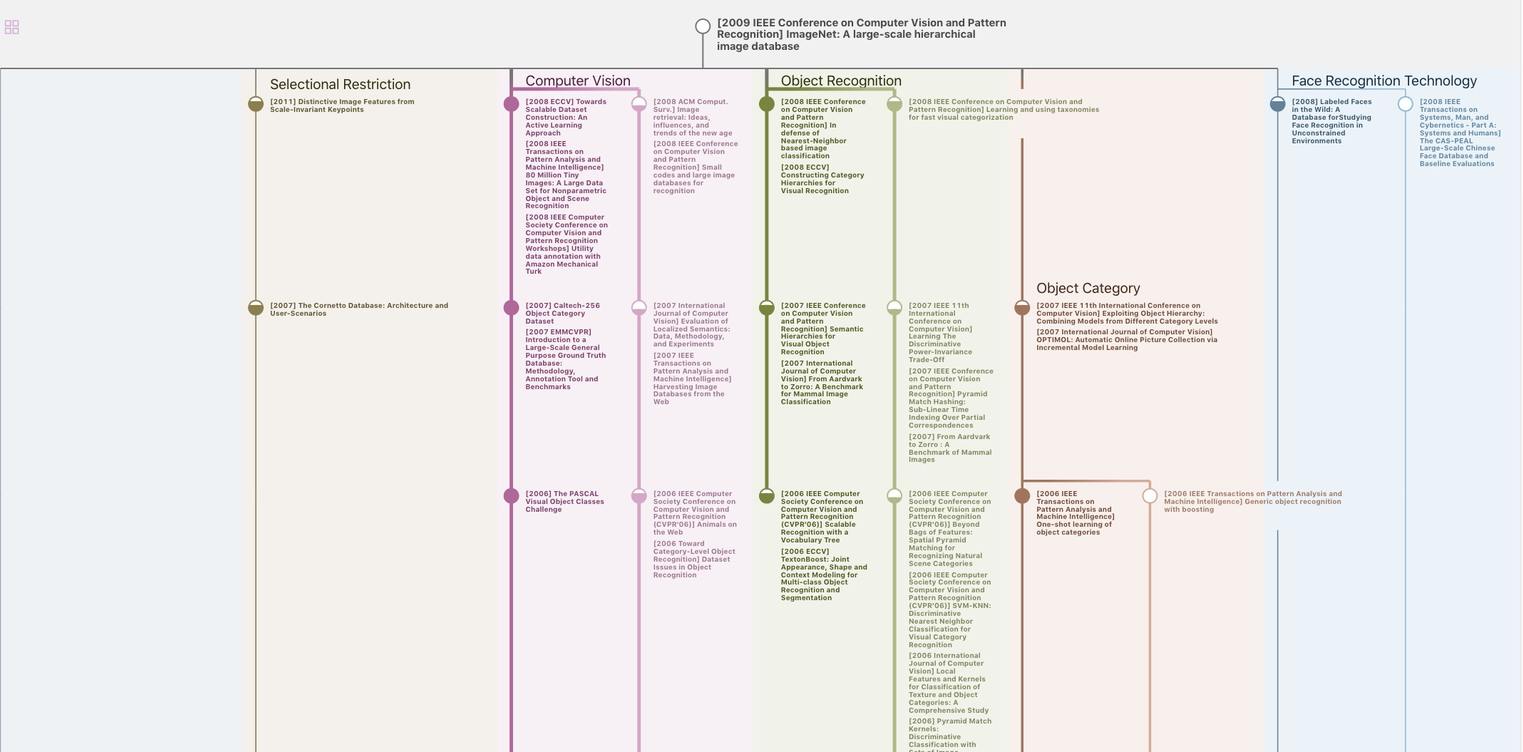
生成溯源树,研究论文发展脉络
Chat Paper
正在生成论文摘要