Deep Space Probing For Point Cloud Analysis
2020 25TH INTERNATIONAL CONFERENCE ON PATTERN RECOGNITION (ICPR)(2020)
摘要
3D points distribute in a continuous 3D space irregularly, thus directly adapting 2D image convolution to 3D points is not an easy job. Previous works often artificially divide the space into regular grids, yet it could be suboptimal to learn geometry. In this paper, we propose SPCNN, namely, Space Probing Convolutional Neural Network, which naturally generalizes image CNN to deal with point clouds. The key idea of SPCNN is learning to probe the 3D space in an adaptive manner. Specifically, we define a pool of learnable convolutional weights, and let each point in the local region learn to choose a suitable convolutional weight from the pool. This is achieved by constructing a geometry guided index-mapping function that implicitly establishes a correspondence between convolutional weights and some local regions in the neighborhood (Fig. 1). In this way, the index-mapping function learns to adaptively partition nearby space for local geometry pattern recognition. With this convolution as a basic operator, SPCNN, a hierarchical architecture can be developed for effective point cloud analysis. Extensive experiments on challenging benchmarks across three tasks demonstrate that SPCNN achieves the state-of-the-art or has competitive performance.
更多查看译文
关键词
deep space probing,SPCNN,learnable convolutional weights,geometry guided index-mapping function,local geometry pattern recognition,effective point cloud analysis,point cloud analysis,continuous 3D space irregularly,2D image convolution,space probing convolutional neural network,hierarchical architecture
AI 理解论文
溯源树
样例
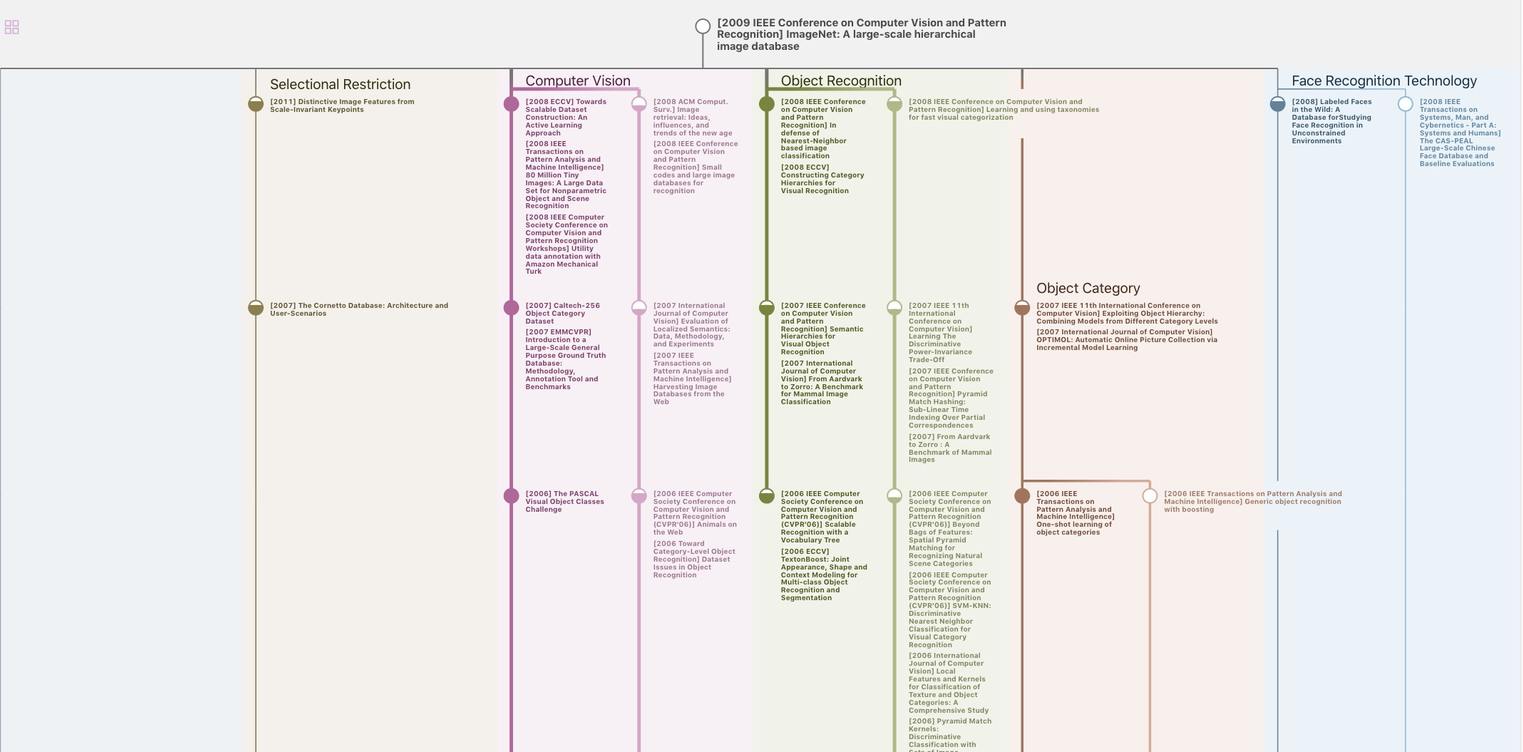
生成溯源树,研究论文发展脉络
Chat Paper
正在生成论文摘要