Derivation Of Geometrically And Semantically Annotated Uav Datasets At Large Scales From 3d City Models
2020 25TH INTERNATIONAL CONFERENCE ON PATTERN RECOGNITION (ICPR)(2020)
摘要
While in high demand for the development of deep learning approaches, extensive datasets of annotated unmanned aerial vehicle (UAV) imagery are still scarce today. Manual annotation, however, is time-consuming and, thus, has limited the potential for creating large-scale datasets. We tackle this challenge by presenting a procedure for the automatic creation of simulated UAV image sequences in urban areas and pixel-level annotations from publicly available data sources. We synthesize photo-realistic UAV imagery from GOGGLE EARTH STUDIO and derive annotations from an open CityGML model that not only provides geometric but also semantic information.The first dataset we exemplarily created using our approach contains 144 000 images of Berlin, Germany, with four types of annotations, namely semantic labels as well as depth, surface normals, and edge maps. Based on this specific case, we demonstrate the entire pipeline and give a comprehensive overview of technical obstacles and solutions. In experiments, we evaluated the quality of our dataset and its potential application in monocular depth estimation and semantic segmentation using deep learning methods. To facilitate the creation of further datasets, we provide our source code along with the produced dataset at https://github.com/sian1995/large.scaleuavdataset.
更多查看译文
关键词
Unmanned Aerial Vehicles (UAVs), Geometry, Semantics, Automatic Annotation, 3D City Models
AI 理解论文
溯源树
样例
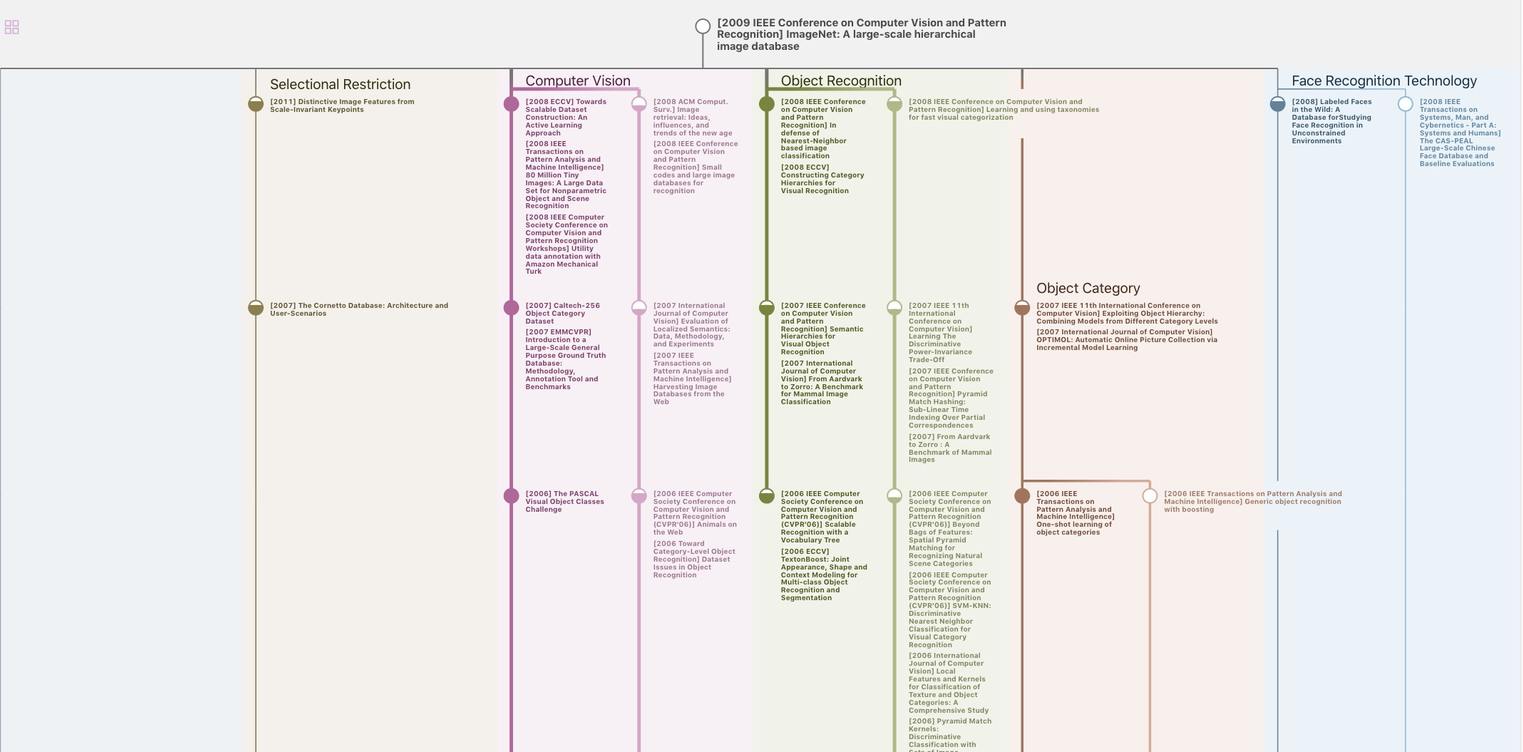
生成溯源树,研究论文发展脉络
Chat Paper
正在生成论文摘要