Group-Wise Feature Orthogonalization And Suppression For Gan Based Facial Attribute Translation
2020 25TH INTERNATIONAL CONFERENCE ON PATTERN RECOGNITION (ICPR)(2020)
摘要
Generative Adversarial Network (GAN) has been widely used for object attribute editing. However, the semantic correlation, resulted from the feature map interaction in the generative network of GAN, may impair the generalization ability of the generative network. In this work, semantic disentanglement is introduced in GAN to reduce the attribute correlation. The feature maps of the generative network are first grouped with an efficient clustering algorithm based on hash encoding, which are used to excavate hidden semantic attributes and calculate the group-wise orthogonality loss for the reduction of attribute entanglement. Meanwhile, the feature maps falling in the intersection regions of different groups are further suppressed to reduce the attribute-wise interaction. Extensive experiments reveal that the proposed GAN generated more genuine objects than the state of the arts. Quantitative results of classification accuracy, inception score and FID score further justify the effectiveness of the proposed GAN.
更多查看译文
关键词
semantic correlation,feature map interaction,generative network,semantic disentanglement,attribute correlation,feature maps,hidden semantic attributes,group-wise orthogonality loss,attribute entanglement,attribute-wise interaction,group-wise feature orthogonalization,GAN based facial attribute translation,Generative Adversarial Network,object attribute editing
AI 理解论文
溯源树
样例
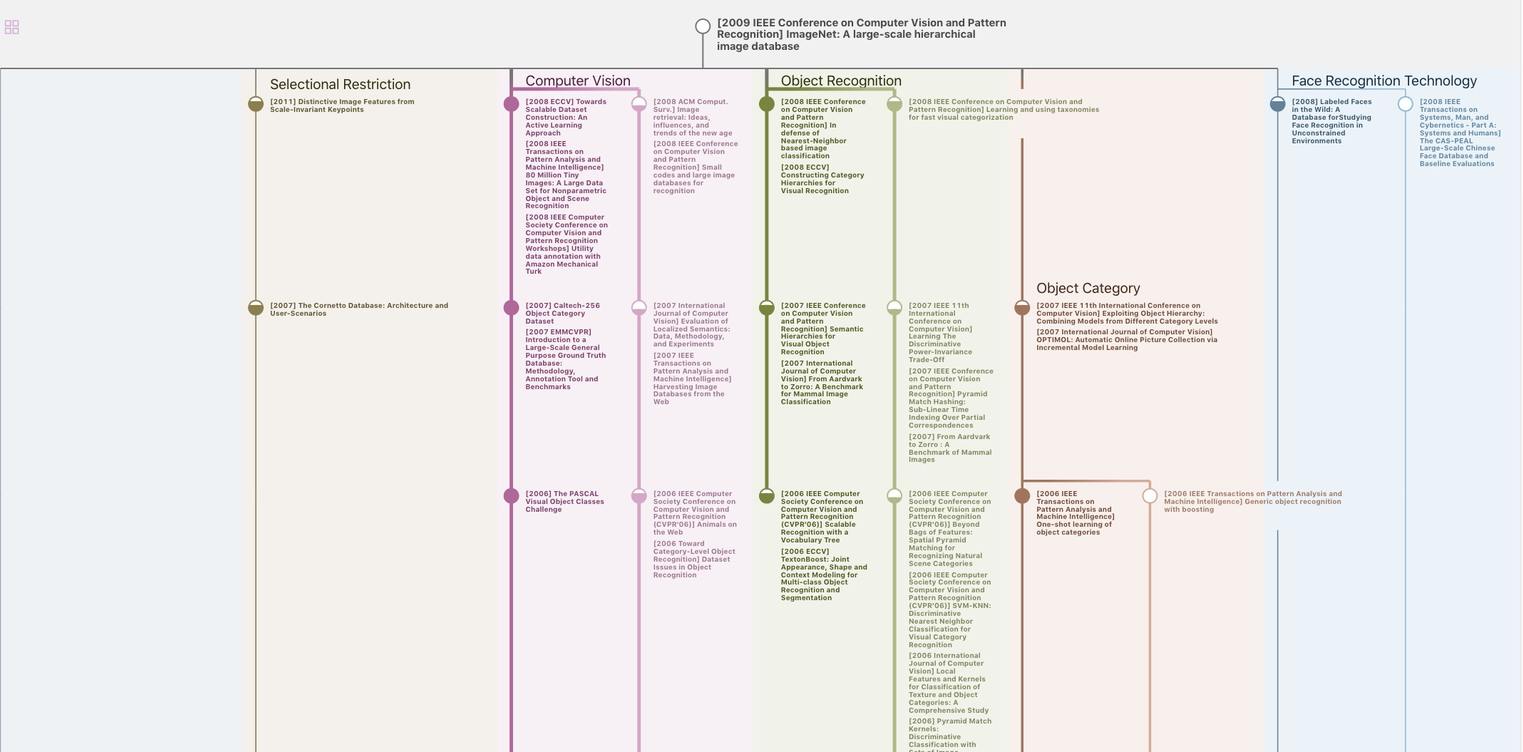
生成溯源树,研究论文发展脉络
Chat Paper
正在生成论文摘要