Generalized Local Attention Pooling For Deep Metric Learning
2020 25TH INTERNATIONAL CONFERENCE ON PATTERN RECOGNITION (ICPR)(2020)
摘要
Deep metric learning has been key to recent advances in face verification and image retrieval amongst others. These systems consist on a feature extraction block (extracts feature maps from images) followed by a spatial dimensionality reduction block (generates compact image representations from the feature maps) and an embedding generation module (projects the image representation to the embedding space). While research on deep metric learning has focused on improving the losses for the embedding generation module, the dimensionality reduction block has been overlooked.In this work, we propose a novel method to generate compact image representations which uses local spatial information through an attention mechanism, named Generalized Local Attention Pooling (GLAP). This method, instead of being placed at the end layer of the backbone, is connected at an intermediate level, resulting in lower memory requirements. We assess the performance of the aforementioned method by comparing it with multiple dimensionally reduction techniques, demonstrating the importance of using attention weights to generate robust compact image representations. Moreover, we compare the performance of multiple state-of-the-art losses using the standard deep metric learning system against the same experiment with our GLAP.Experiments showcase that the proposed Generalized Local Attention Pooling mechanism outperforms other pooling methods when compared with current state-of-the-art losses for deep metric learning.
更多查看译文
关键词
image representation,embedding generation module,local spatial information,named Generalized Local Attention Pooling,multiple dimensionality reduction techniques,robust compact image representations,standard deep metric learning system,Generalized Local Attention Pooling mechanism,face verification,image retrieval,feature extraction block,spatial dimensionality reduction block,feature maps
AI 理解论文
溯源树
样例
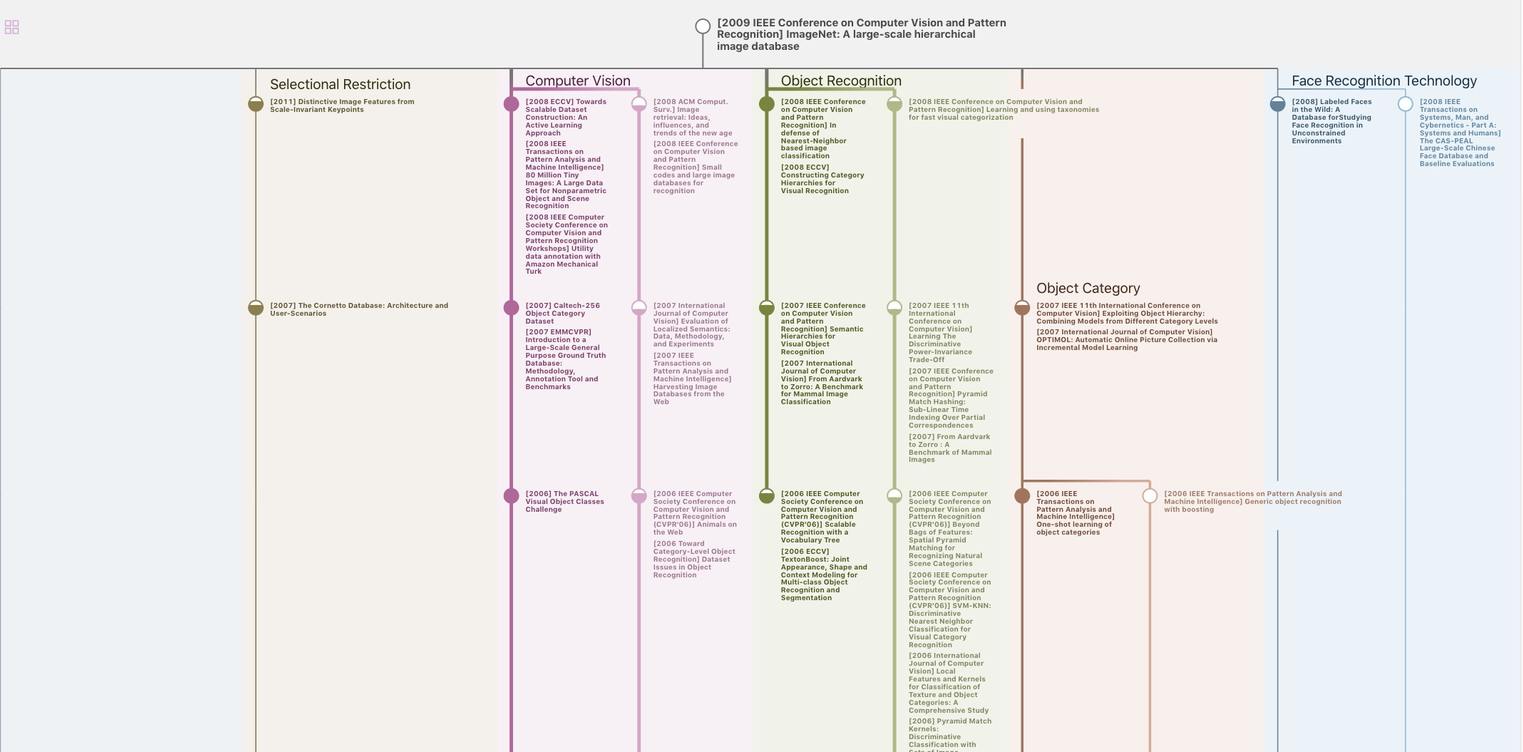
生成溯源树,研究论文发展脉络
Chat Paper
正在生成论文摘要