Seci-Gan: Semantic And Edge Completion For Dynamic Objects Removal
2020 25TH INTERNATIONAL CONFERENCE ON PATTERN RECOGNITION (ICPR)(2020)
摘要
Image inpainting aims at synthesizing the missing content of damaged or corrupted images to produce visually realistic restorations; typical applications are in image restoration, automatic scene editing, super-resolution, and dynamic object removal. In this paper, we propose Semantic and Edge Conditioned Inpainting Generative Adversarial Network (SECI-GAN), an architecture that jointly exploits the high-level cues extracted by semantic segmentation and the fine-grained details captured by edge extraction to condition the image inpainting process. SECI-GAN is designed with a particular focus on recovering big regions belonging to the same object (e.g. cars or pedestrians) in the context of dynamic object removal from complex street views. To demonstrate the effectiveness of SECI-GAN, we evaluate our results on the Cityscapes dataset, showing that SECI-GAN is better than competing state-of-the-art models at recovering the structure and the content of the missing parts while producing consistent predictions.
更多查看译文
关键词
SECI-GAN,dynamic objects removal,corrupted images,visually realistic restorations,image restoration,semantic segmentation,edge completion,image inpainting,damaged images,automatic scene editing,semanti edge conditioned inpainting generative adversarial network,big regions recovery,complex street views,Cityscapes dataset
AI 理解论文
溯源树
样例
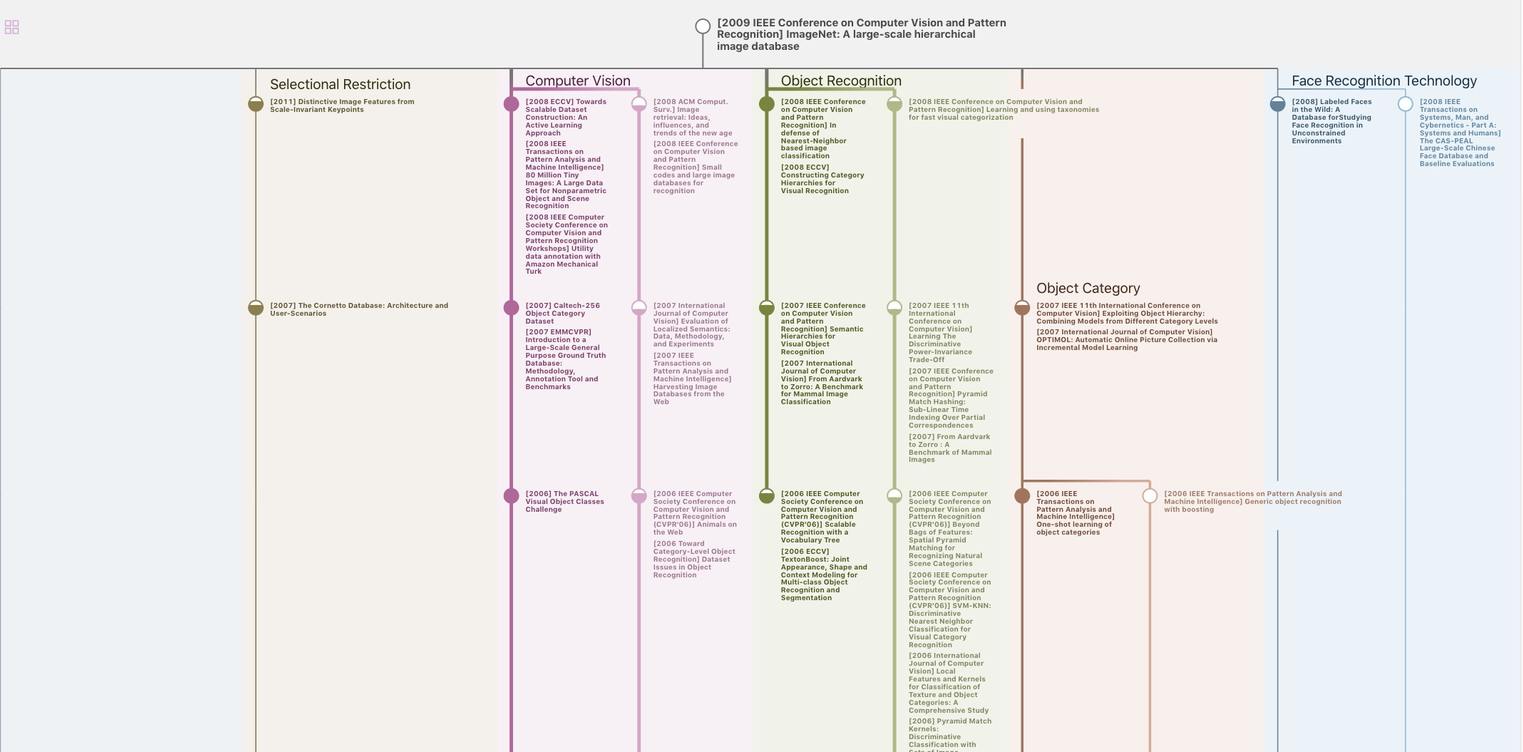
生成溯源树,研究论文发展脉络
Chat Paper
正在生成论文摘要