A Detection-Based Approach To Multiview Action Classification In Infants
2020 25TH INTERNATIONAL CONFERENCE ON PATTERN RECOGNITION (ICPR)(2020)
摘要
Activity recognition in children and infants is important in applications such as safety monitoring, behavior assessment, and child-robot interaction, among others. However, it differs from activity recognition in adults not only because body poses and proportions are different, but also because of the way in which actions are performed. This paper addresses the problem of infant action classification in challenging conditions. The actions are performed in a pediatric rehabilitation environment in which not only infants but also robots and adults are present, with the infant being one of the smallest actors in the scene. We propose a multiview action classification system based on Faster R-CNN and LSTM networks, which fuses information from different views by using learnable fusion coefficients derived from detection confidence scores. The proposed system is view-independent, learns features that are close to view-invariant, and can handle new or missing views at test time. Our approach outperforms the state-of-the-art baseline model for a small dataset (2 subjects, 10-24 months old) by 11.4% in terms of average classification accuracy in four classes (crawl, sit, stand and walk). Moreover, experiments in an extended dataset (6 subjects, 8-24 months old) show that the proposed fusion strategy outperforms all the alternative fusion methods studied.
更多查看译文
关键词
detection-based approach,activity recognition,safety monitoring,behavior assessment,child-robot interaction,body poses,proportions,infant action classification,pediatric rehabilitation environment,multiview action classification system,detection confidence scores,view-independent,view-invariant,missing views,average classification accuracy,time 10.0 month to 24.0 month,time 8.0 month to 24.0 month
AI 理解论文
溯源树
样例
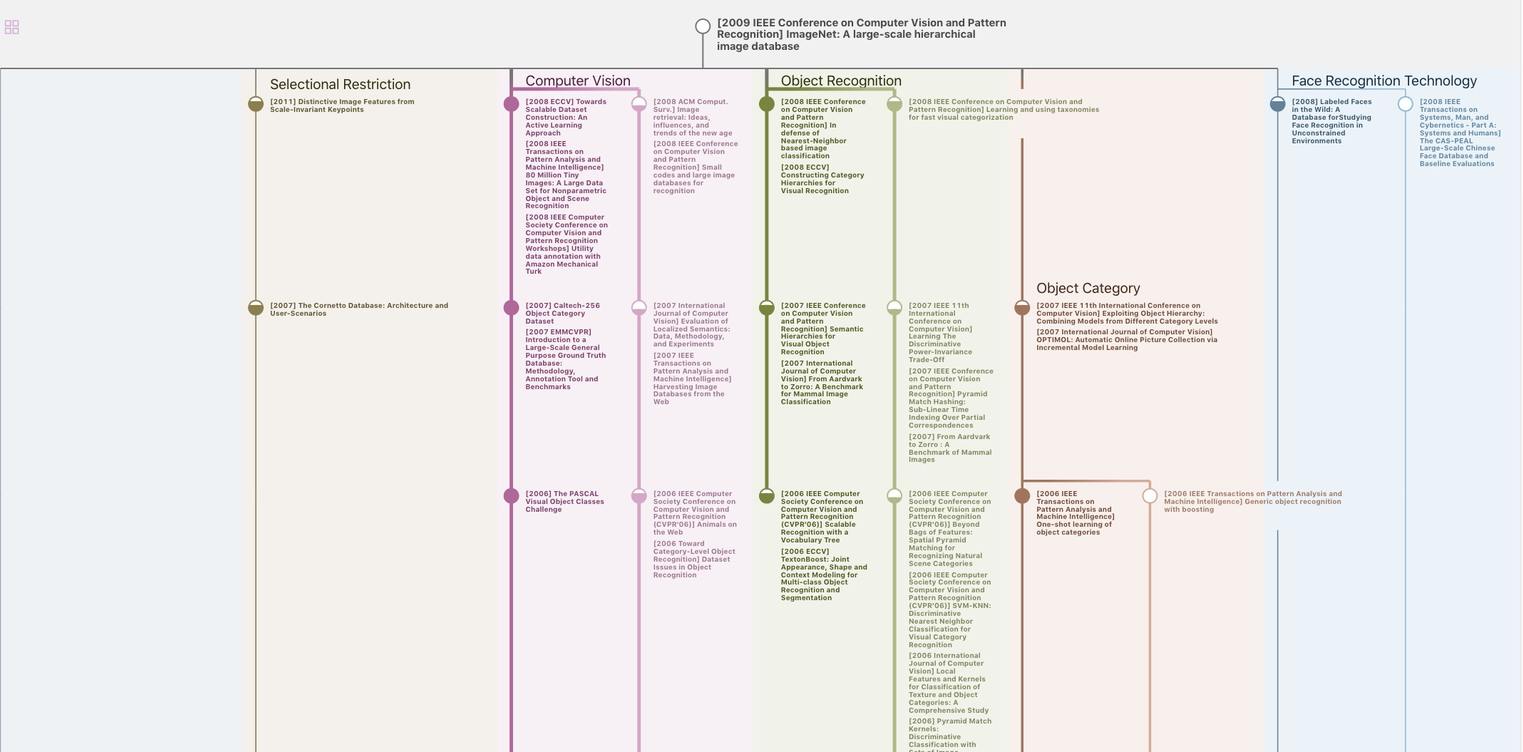
生成溯源树,研究论文发展脉络
Chat Paper
正在生成论文摘要