Progressive Gradient Pruning for Classification, Detection and Domain Adaptation
2020 25TH INTERNATIONAL CONFERENCE ON PATTERN RECOGNITION (ICPR)(2021)
摘要
Although deep neural networks (NNs) have achieved state-of-the-art accuracy in many visual recognition tasks, the growing computational complexity and energy consumption of networks remains an issue, especially for applications on platforms with limited resources and requiring real-time processing. Filter pruning techniques have recently shown promising results for the compression and acceleration of convolutional NNs (CNNs). However, these techniques involve numerous steps and complex optimisations because some only prune after training CNNs, while others prune from scratch during training, by integrating sparsity constraints or by modifying the loss function. In this paper, we introduce a new Progressive Gradient Pruning (PGP) technique for iterative filter pruning during training. In contrast to previous progressive pruning techniques, it relies on a novel filter selection criterion that measures the change in filter weights, uses a new hard and soft pruning strategy, and effectively adapts momentum tensors during the backward propagation pass. Experimental results obtained after training various CNNs on benchmark datasets for image classification, object detection and domain adaptation indicate that our PGP technique can achieve a better trade-off between classification accuracy and network (time and memory) complexity than PSFP and other state-of-the-art filter pruning techniques. Code is available on GitHub link: https://github.com/Anon6627/Pruning-PGP.
更多查看译文
关键词
progressive pruning techniques,filter selection criterion,filter weights,soft pruning strategy,image classification,object detection,domain adaptation,visual recognition tasks,computational complexity,energy consumption,filter pruning techniques,convolutional neural nets,CNN,progressive gradient pruning technique,momentum tensors,backward propagation pass
AI 理解论文
溯源树
样例
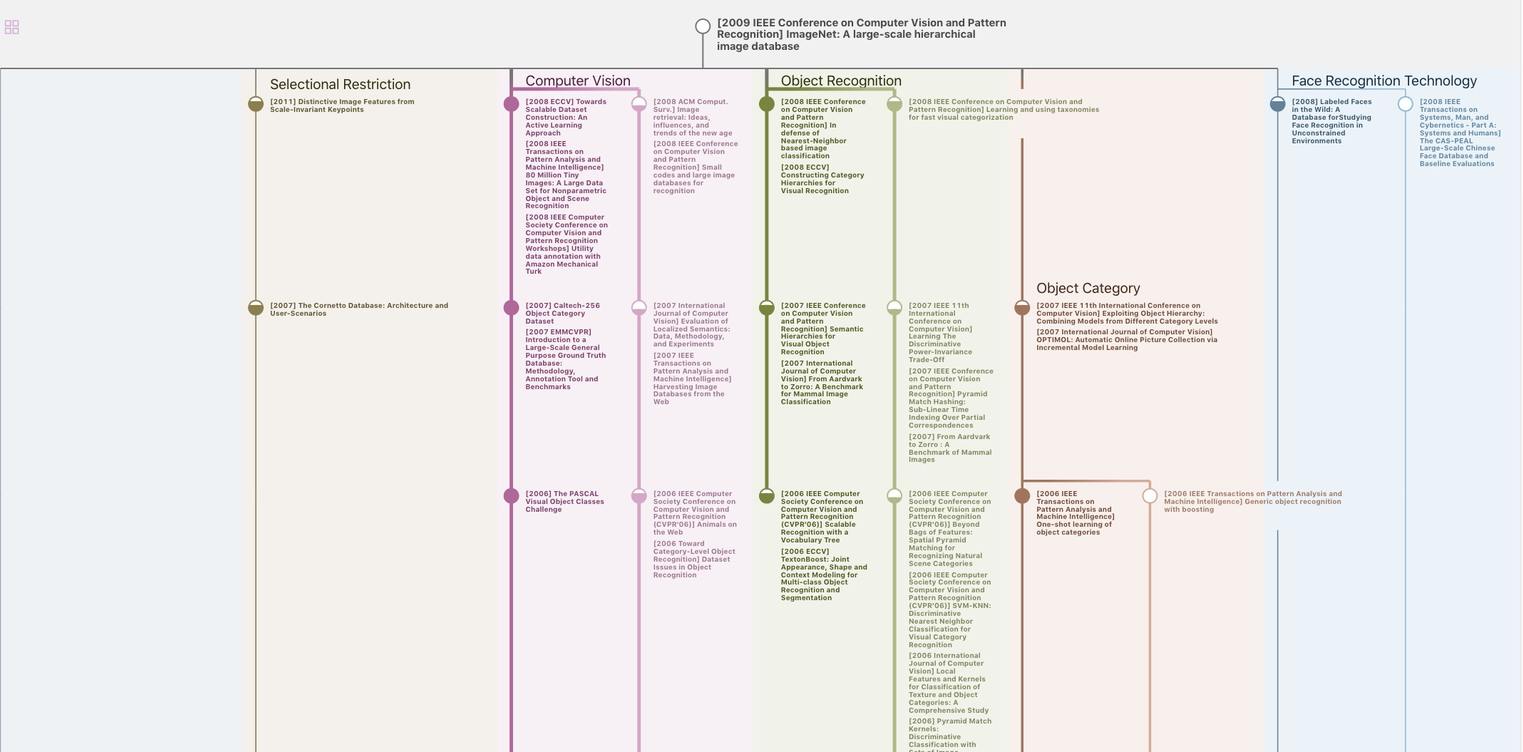
生成溯源树,研究论文发展脉络
Chat Paper
正在生成论文摘要