Efficient Super Resolution By Recursive Aggregation
2020 25TH INTERNATIONAL CONFERENCE ON PATTERN RECOGNITION (ICPR)(2020)
摘要
Deep neural networks have achieved remarkable results on image super resolution (SR), but the efficiency problem of deep SR networks is rarely studied. We experimentally find that many sequentially stacked convolutional blocks in nowadays SR networks are far from being fully optimized, which largely damages their overall efficiency. It indicates that comparable or even better results could be achieved with less but sufficiently optimized blocks. In this paper, we try to construct more efficient SR model via the proposed recursive aggregation network (RAN). It recursively aggregates convolutional blocks in different orders, and avoids too many sequentially stacked blocks. In this way, multiple shortcuts are introduced in RAN, and help gradients easier flow to all inner layers, even for very deep SR networks. As a result, all blocks in RAN can be better optimized, thus RAN can achieve better performance with smaller model size than existing methods.
更多查看译文
关键词
deep neural networks,image super resolution,deep SR networks,sequentially stacked convolutional blocks,recursive aggregation network,RAN
AI 理解论文
溯源树
样例
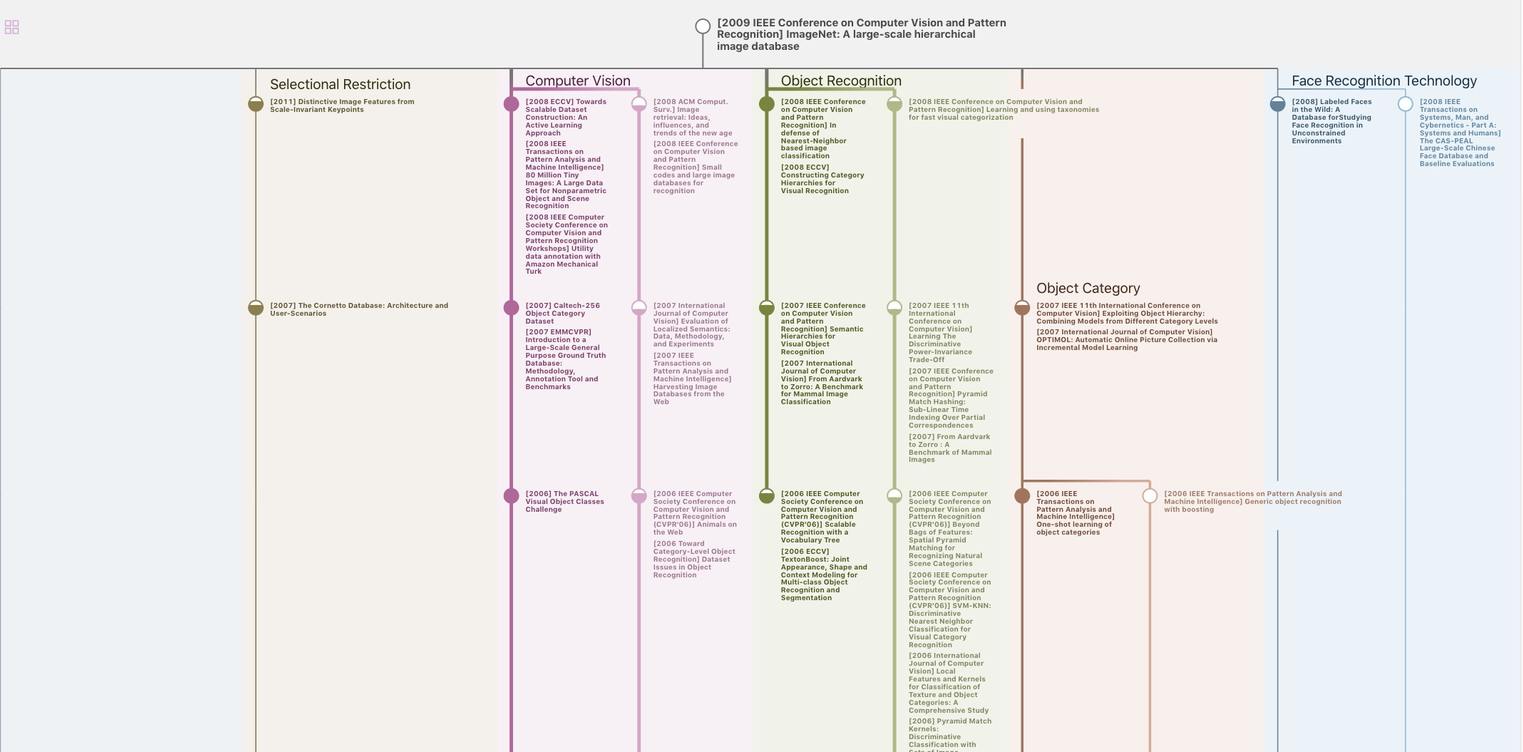
生成溯源树,研究论文发展脉络
Chat Paper
正在生成论文摘要