Learning Natural Thresholds For Image Ranking
2020 25TH INTERNATIONAL CONFERENCE ON PATTERN RECOGNITION (ICPR)(2020)
摘要
For image ranking tasks with naturally continuous output, such as age and scenicness estimation, it is common to discretize the label range and apply methods from (ordered) classification analysis. In this paper, we propose a data-driven approach for simultaneous representation learning and label discretization. Compared to arbitrarily selecting thresholds, we seek to learn thresholds and image representations by minimizing a novel loss function in an end-to-end model. We demonstrate our combined approach on a variety of image ranking tasks and demonstrate that it outperforms task-specific methods. Additionally, our learned partitioning scheme can be transferred to improve methods that rely on discretization.
更多查看译文
关键词
natural thresholds,image ranking tasks,scenicness estimation,classification analysis,data-driven approach,representation learning,label discretization,image representations,end-to-end model,task-specific methods,learned partitioning scheme,loss function
AI 理解论文
溯源树
样例
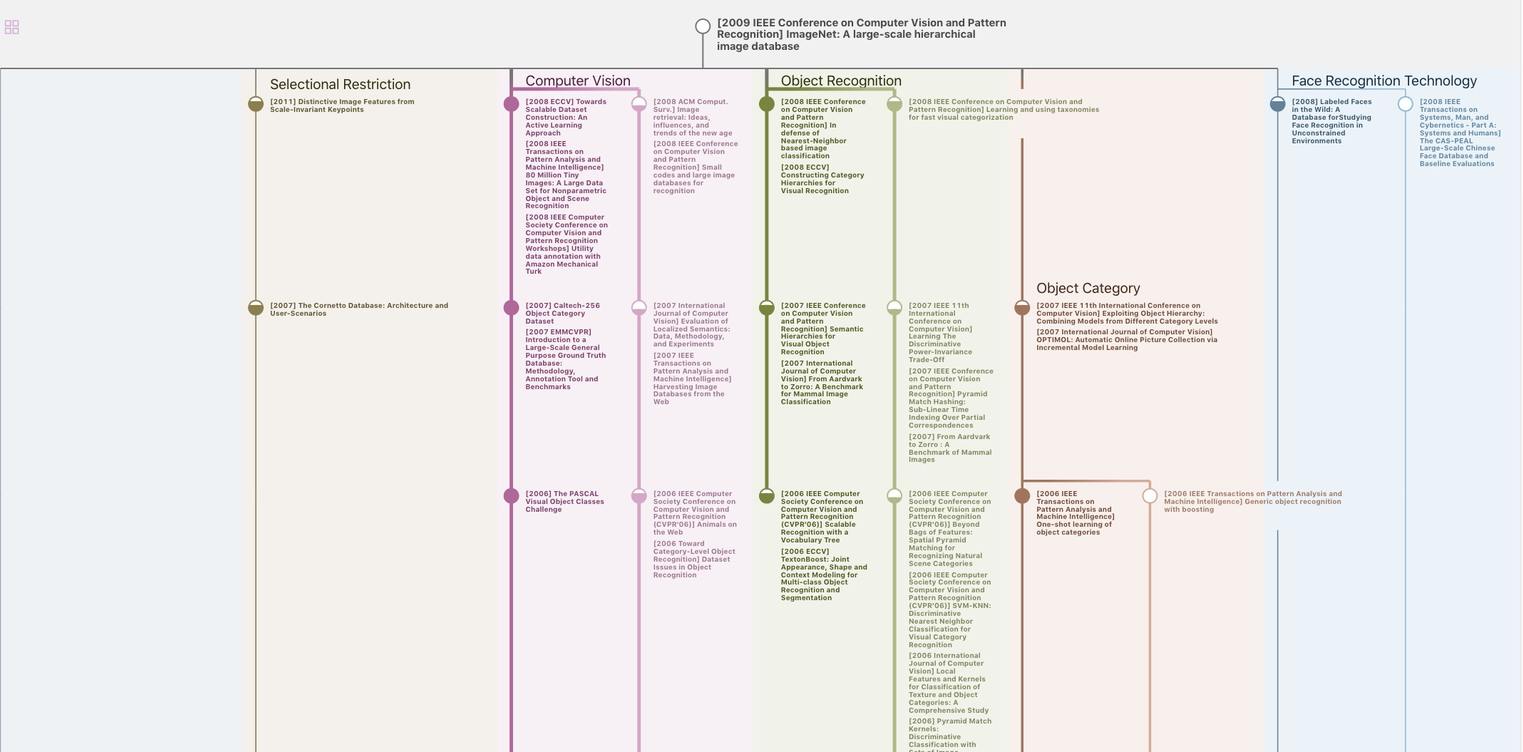
生成溯源树,研究论文发展脉络
Chat Paper
正在生成论文摘要