Residual Fractal Network For Single Image Super Resolution By Widening And Deepening
2020 25TH INTERNATIONAL CONFERENCE ON PATTERN RECOGNITION (ICPR)(2020)
摘要
The architecture of the convolutional neural network (CNN) plays an important role in single image super-resolution (SISR). However, most models proposed in recent years usually transplant methods or architectures that perform well in other vision fields. Thence they do not combine the characteristics of super-resolution (SR) and ignore the key information brought by the recurring texture feature in the image. To utilize patch-recurrence in SR and the high correlation of texture, we propose a residual fractal convolutional block (RFCB) and expand its depth and width to obtain residual fractal network (RFN), which contains two variations, deep residual fractal network (DRFN) and wide residual fractal network (WRFN). RFCB is recursive with multiple branches of magnified receptive field. Through the phased feature fusion module, the network focuses on extracting high-frequency texture feature that repeatedly appear in the i mage. We also introduce residual in residual (RIR) structure to RFCB that enables abundant low-frequency feature feed into deeper layers and reduce the difficulties of network training. RFN is the first supervised learning method to combine the patch-recurrence characteristic in SISR into network design. Extensive experiments demonstrate that RFN outperforms state-of-the-art SISR methods in terms of both quantitative metrics and visual quality, while the amount of parameters has been greatly optimized. The source code and pre-trained models are released in :ps://github.comaiahangGu/RF]..
更多查看译文
关键词
supervised learning,SISR,network design,patch-recurrence characteristic,phased feature fusion module,wide residual fractal network,deep residual fractal network,RFN,RFCB,residual fractal convolutional block,texture feature,convolutional neural network,single image super resolution
AI 理解论文
溯源树
样例
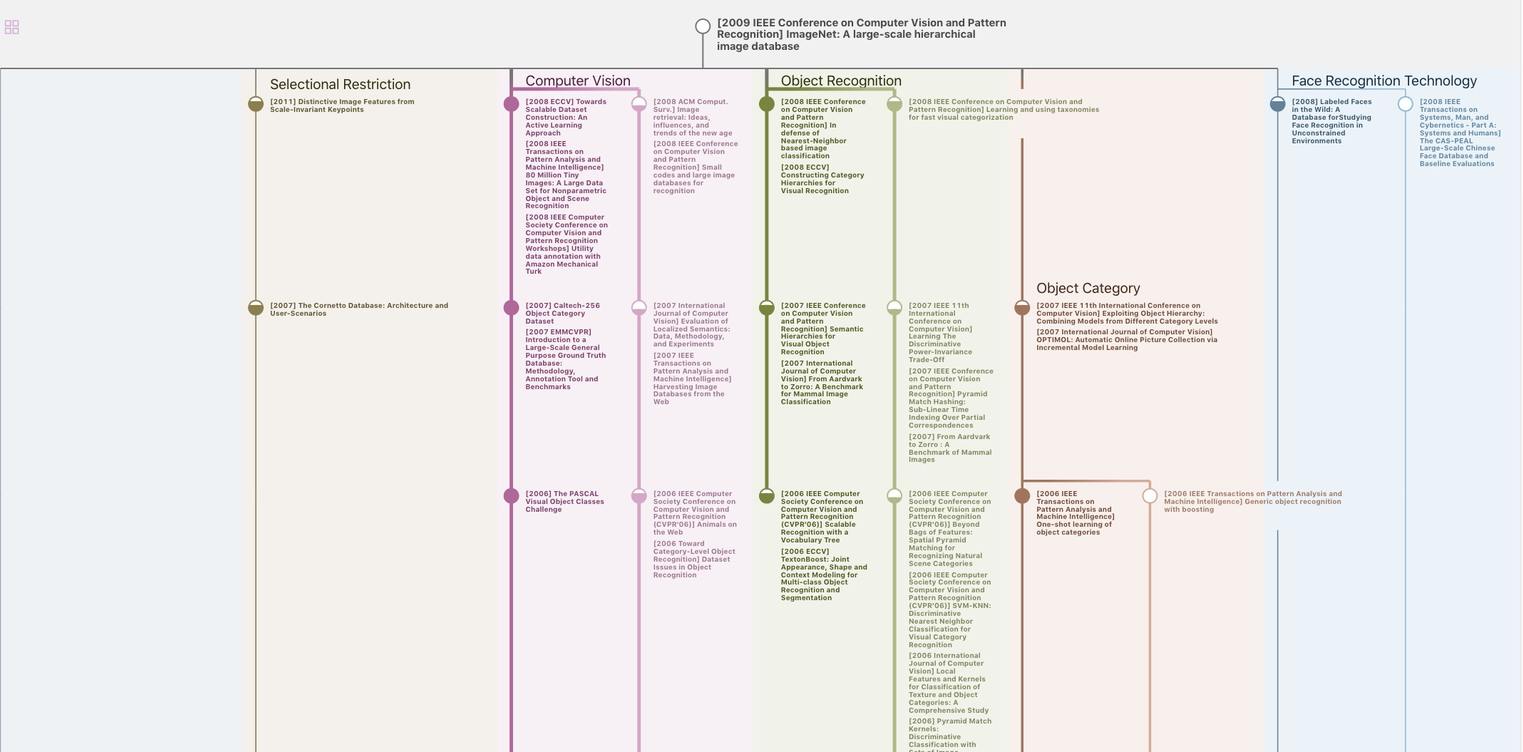
生成溯源树,研究论文发展脉络
Chat Paper
正在生成论文摘要