Adversarially Constrained Interpolation for Unsupervised Domain Adaptation
2020 25TH INTERNATIONAL CONFERENCE ON PATTERN RECOGNITION (ICPR)(2021)
摘要
We address the problem of unsupervised domain adaptation (UDA) which aims at adapting models trained on a labeled domain to a completely unlabeled domain. One way to achieve this goal is to learn a domain-invariant representation. However, this approach is subject to two challenges: samples from two domains are insufficient to guarantee domain-invariance at most part of the latent space, and neighboring samples from the target domain may not belong to the same class on the low-dimensional manifold. To mitigate these shortcomings, we propose two strategies. First, we incorporate a domain mixup strategy in domain adversarial learning model by linearly interpolating between the source and target domain samples. This allows the latent space to be continuous and yields an improvement of the domain matching. Second, the domain discriminator is regularized via judging the relative difference between both domains for the input mixup features, which speeds up the domain matching. Experiment results show that our proposed model achieves a superior performance on different tasks under various domain shifts and data complexity.
更多查看译文
关键词
adversarially constrained interpolation,domain-invariant representation,completely unlabeled domain,labeled domain,adapting models,unsupervised domain adaptation,data complexity,domain shifts,domain discriminator,domain matching,domain adversarial,domain mixup strategy,target domain,latent space
AI 理解论文
溯源树
样例
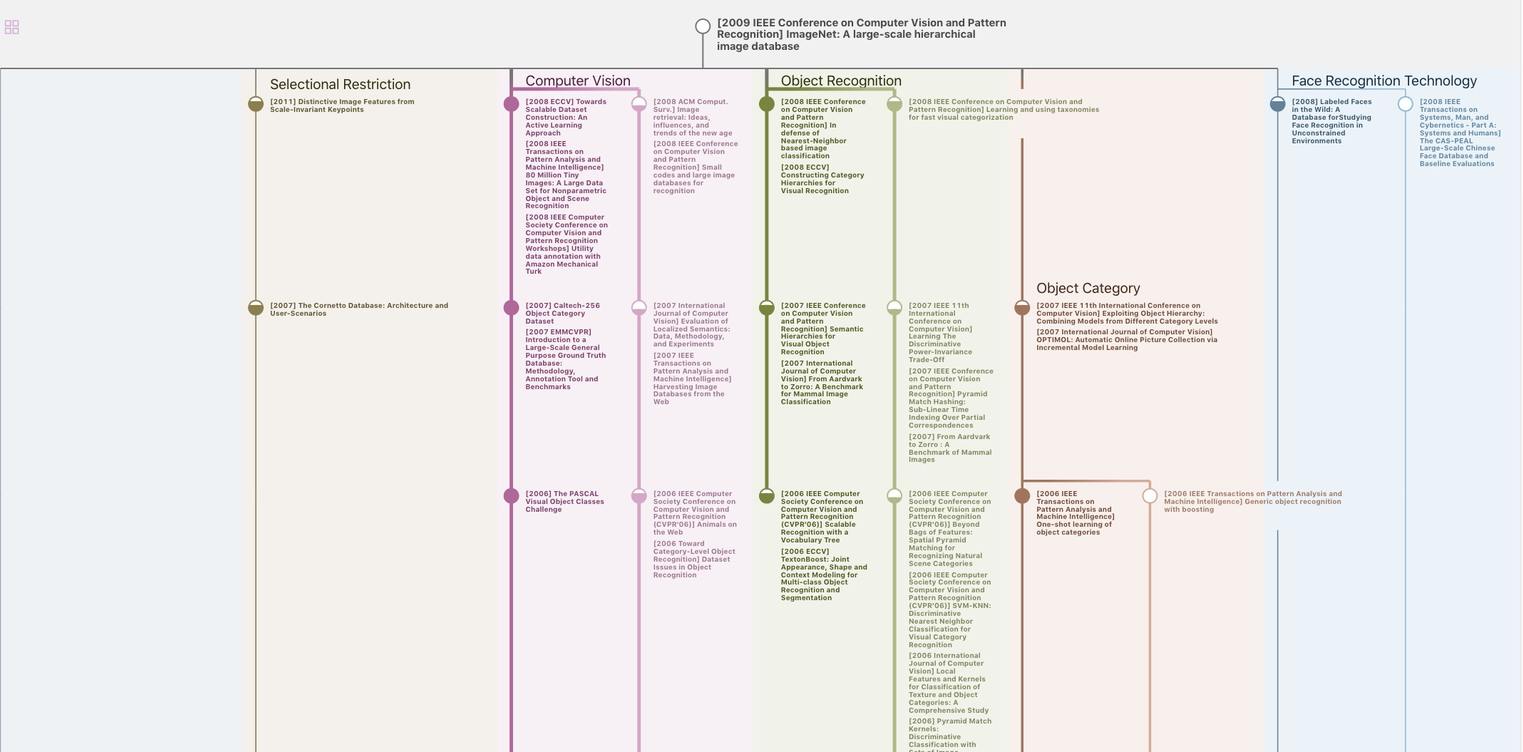
生成溯源树,研究论文发展脉络
Chat Paper
正在生成论文摘要