Uncertainty-Aware Data Augmentation For Food Recognition
2020 25TH INTERNATIONAL CONFERENCE ON PATTERN RECOGNITION (ICPR)(2020)
Abstract
Food recognition has recently attracted attention of many researchers. However, high food ambiguity, inter-class variability and intra-class similarity define a real challenge for the Deep learning and Computer Vision algorithms. In order to improve their performance, it is necessary to better understand what the model learns and, from this, to determine the type of data that should be additionally included for being the most beneficial to the training procedure. In this paper, we propose a new data augmentation strategy that estimates and uses the epistemic uncertainty to guide the model training. The method follows an active learning framework, where the new synthetic images are generated from the hard to classify real ones present in the training data based on the epistemic uncertainty. Hence, it allows the food recognition algorithm to focus on difficult images in order to learn their discriminatives features. On the other hand, avoiding data generation from images that do not contribute to the recognition makes it faster and more efficient. We show that the proposed method allows to improve food recognition and provides a better trade-off between micro- and macro-recall measures.
MoreTranslated text
Key words
uncertainty-aware data augmentation,high food ambiguity,inter-class variability,intra-class similarity,Deep learning,Computer Vision algorithms,model learns,training procedure,data augmentation strategy,epistemic uncertainty,model training,active learning framework,training data,food recognition algorithm,data generation
AI Read Science
Must-Reading Tree
Example
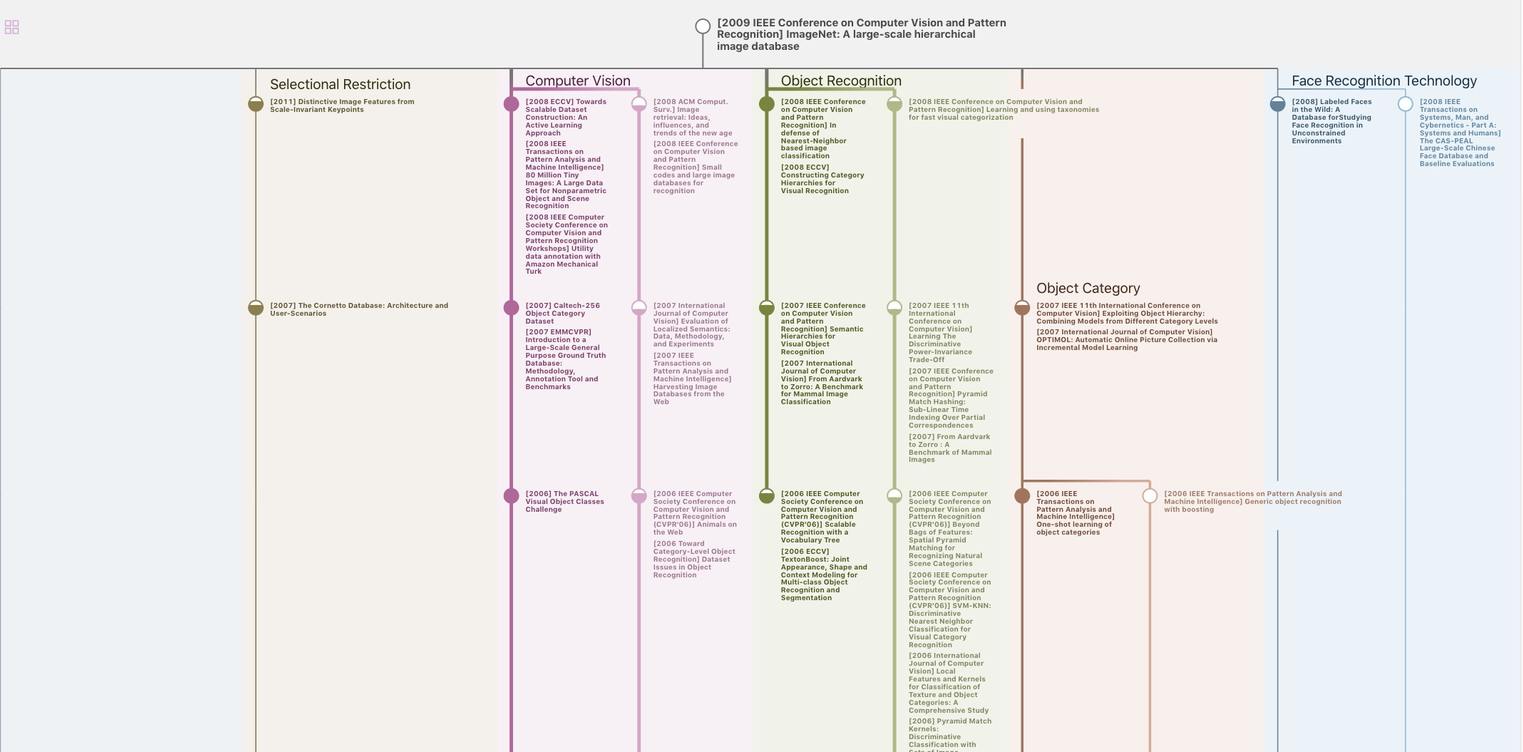
Generate MRT to find the research sequence of this paper
Chat Paper
Summary is being generated by the instructions you defined