Mapping Multiple LSTM Models on FPGAs
2020 INTERNATIONAL CONFERENCE ON FIELD-PROGRAMMABLE TECHNOLOGY (ICFPT 2020)(2020)
摘要
Recurrent Neural Networks (RNNs) and their more recent variant Long Short-Term Memory (LSTM) are utilised in a number of modern applications like Natural Language Processing and human action recognition, where capturing longterm dependencies on sequential and temporal data is required. However, their computational structure imposes a challenge when it comes to their efficient mapping on a computing device due to its memory-bounded nature. As recent approaches aim to capture longer dependencies through the utilisation of Hierarchical and Stacked RNN/LSTM models, i.e. models that utilise multiple LSTM models for prediction, meeting the desired application latency becomes even more challenging. This paper addresses the problem of mapping multiple LSTM models to a device by introducing a framework that alters their computational structure opening opportunities for co-optimising the memory requirements to the target architecture. Targeting an FPGA device, the proposed framework achieves 3× to 5× improved performance over state-of-the-art approaches for the same accuracy loss, opening the path for the deployment of high-performance systems for Hierarchical and Stacked LSTM models.
更多查看译文
关键词
Performance evaluation,Recurrent neural networks,Processor scheduling,Computational modeling,Memory management,Predictive models,Natural language processing
AI 理解论文
溯源树
样例
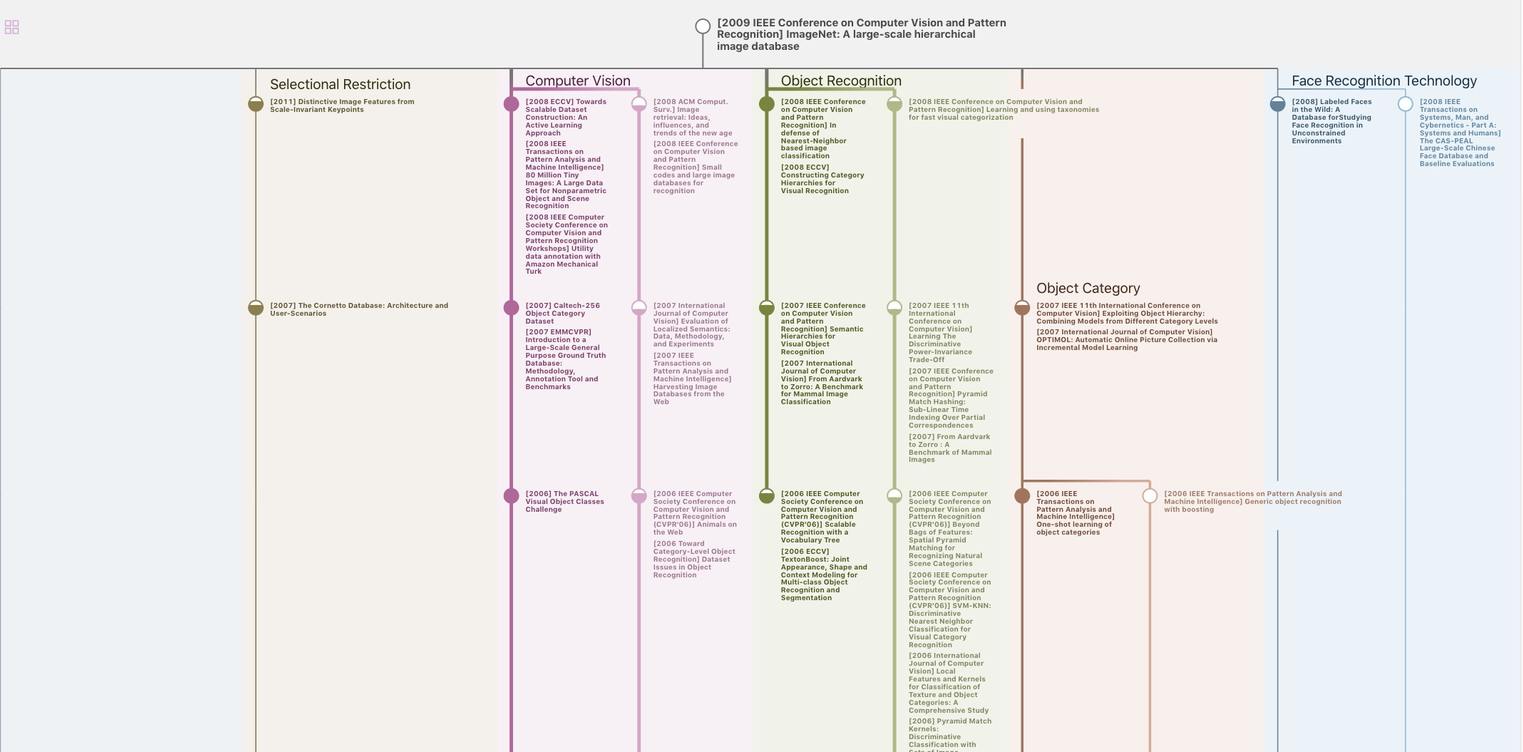
生成溯源树,研究论文发展脉络
Chat Paper
正在生成论文摘要