Hierarchical Topic Model for Tensor Data and Extraction of Weekly and Daily Patterns from Activity Monitor Records
TRENDS AND APPLICATIONS IN KNOWLEDGE DISCOVERY AND DATA MINING, PAKDD 2021(2021)
摘要
Latent Dirichlet allocation (LDA) is a popular topic model for extracting common patterns from discrete datasets. It is extended to the pachinko allocation model (PAM) with a hierarchical topic structure. This paper presents a combination meal allocation (CMA) model, which is a further enhanced topic model from the PAM that has both hierarchical categories and hierarchical topics. We consider count datasets in multiway arrays, i.e., tensors, and introduce a set of topics to each mode of the tensors. The topics in each mode are interpreted as patterns in the topics and categories in the next mode. Despite there being a vast number of combinations in multilevel categories, our model provides simple and interpretable patterns by sharing the topics in each mode. Latent topics and their membership are estimated using Markov chain Monte Carlo (MCMC) methods. We apply the proposed model to step-count data recorded by activity monitors to extract some common activity patterns exhibited by the users. Our model identifies four daily patterns of ambulatory activities (commuting, daytime, nighttime, and early-bird activities) as sub-topics, and six weekly activity patterns as super-topics. We also investigate how the amount of activity in each pattern dynamically affects body weight changes.
更多查看译文
关键词
Topic model, Tensor data, Activity monitor records
AI 理解论文
溯源树
样例
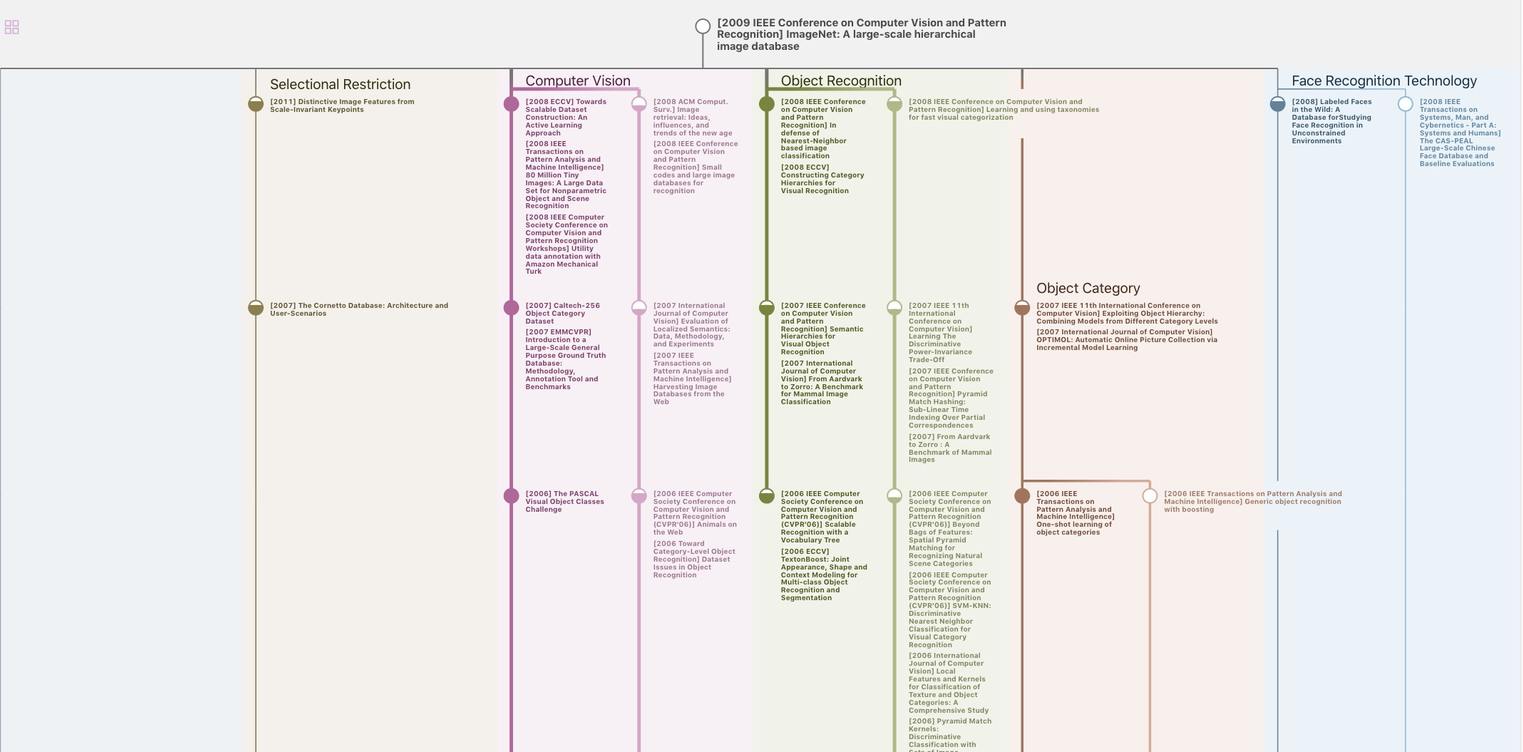
生成溯源树,研究论文发展脉络
Chat Paper
正在生成论文摘要