Feature range analysis
INTERNATIONAL JOURNAL OF DATA SCIENCE AND ANALYTICS(2021)
Abstract
We propose a feature range analysis algorithm whose aim is to derive features that explain the response variable better than the original features. Moreover, for binary classification problems, and for regression problems where positive and negative samples can be defined (e.g., using a threshold value of the numeric response variable), our aim is to derive features that explain, characterize and isolate the positive samples or subsets of positive samples that have the same root cause. Each derived feature represents a single or multi-dimensional subspace of the feature space, where each dimension is specified as a feature range pair for numeric features, and as a feature-level pair for categorical features. We call these derived features range features . Unlike most rule learning and subgroup discovery algorithms, the response variable can be numeric, and our algorithm does not require a discretization of the response. The algorithm has been applied successfully to real-life root-causing tasks in chip design, manufacturing, and validation, at Intel. Furthermore, we propose and experimentally evaluate a number of heuristics for usage of range features in building predictive models, demonstrating that prediction accuracy can be improved for the majority of real-life proprietary and open-source datasets used in the evaluation.
MoreTranslated text
Key words
Range analysis, Feature selection, Feature synthesis, Rule learning, Rule induction, Subgroup discovery, Predictive modeling
AI Read Science
Must-Reading Tree
Example
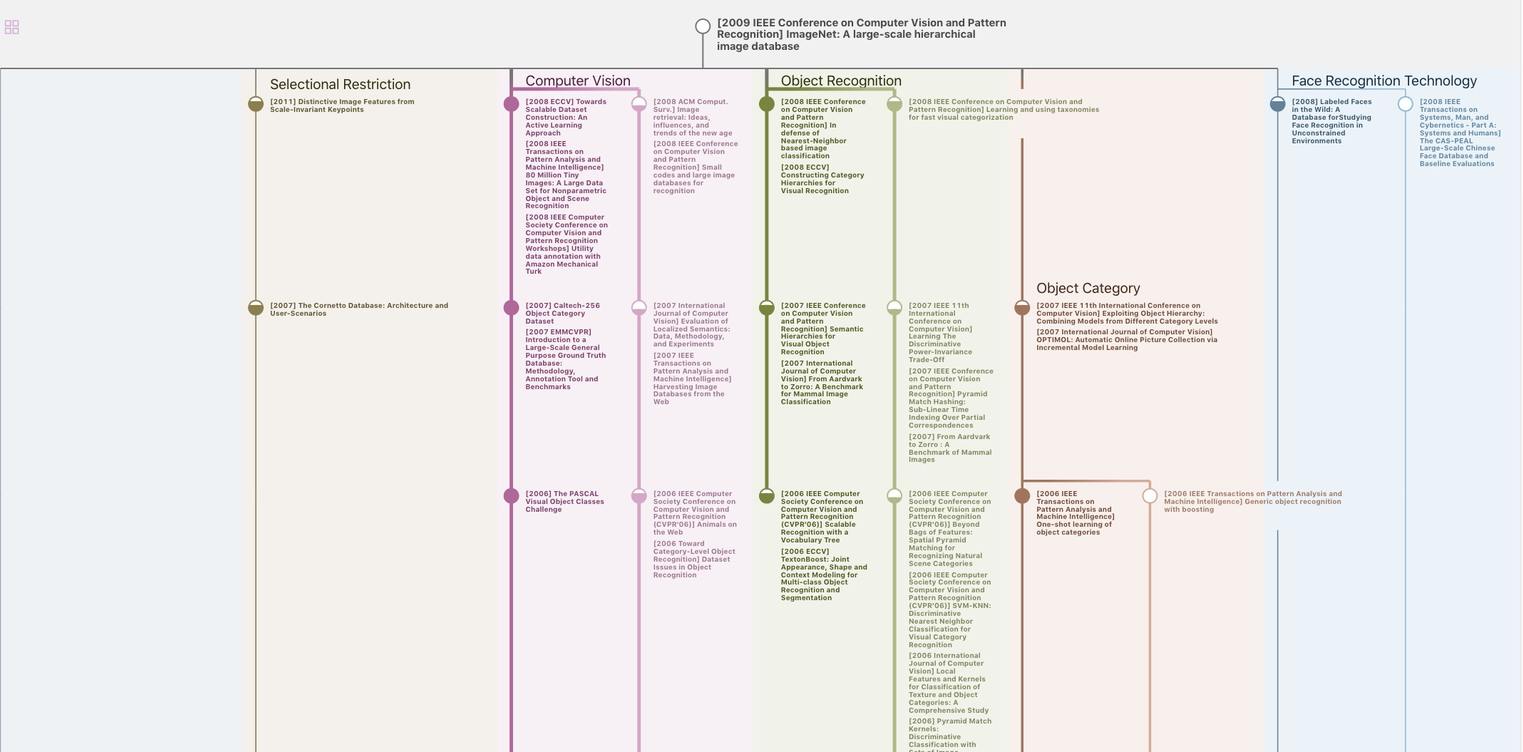
Generate MRT to find the research sequence of this paper
Chat Paper
Summary is being generated by the instructions you defined