Handwritten Mathematical Expression Recognition with Bidirectionally Trained Transformer
DOCUMENT ANALYSIS AND RECOGNITION - ICDAR 2021, PT II(2021)
摘要
Encoder-decoder models have made great progress on handwritten mathematical expression recognition recently. However, it is still a challenge for existing methods to assign attention to image features accurately. Moreover, those encoder-decoder models usually adopt RNN-based models in their decoder part, which makes them inefficient in processing long LATEX sequences. In this paper, a transformer-based decoder is employed to replace RNN-based ones, which makes the whole model architecture very concise. Furthermore, a novel training strategy is introduced to fully exploit the potential of the transformer in bidirectional language modeling. Compared to several methods that do not use data augmentation, experiments demonstrate that our model improves the ExpRate of current state-of-the-art methods on CROHME 2014 by 2.23%. Similarly, on CROHME 2016 and CROHME 2019, we improve the ExpRate by 1.92% and 2.28% respectively.
更多查看译文
关键词
Handwritten mathematical expression recognition, Transformer, Bidirection, Encoder-decoder model
AI 理解论文
溯源树
样例
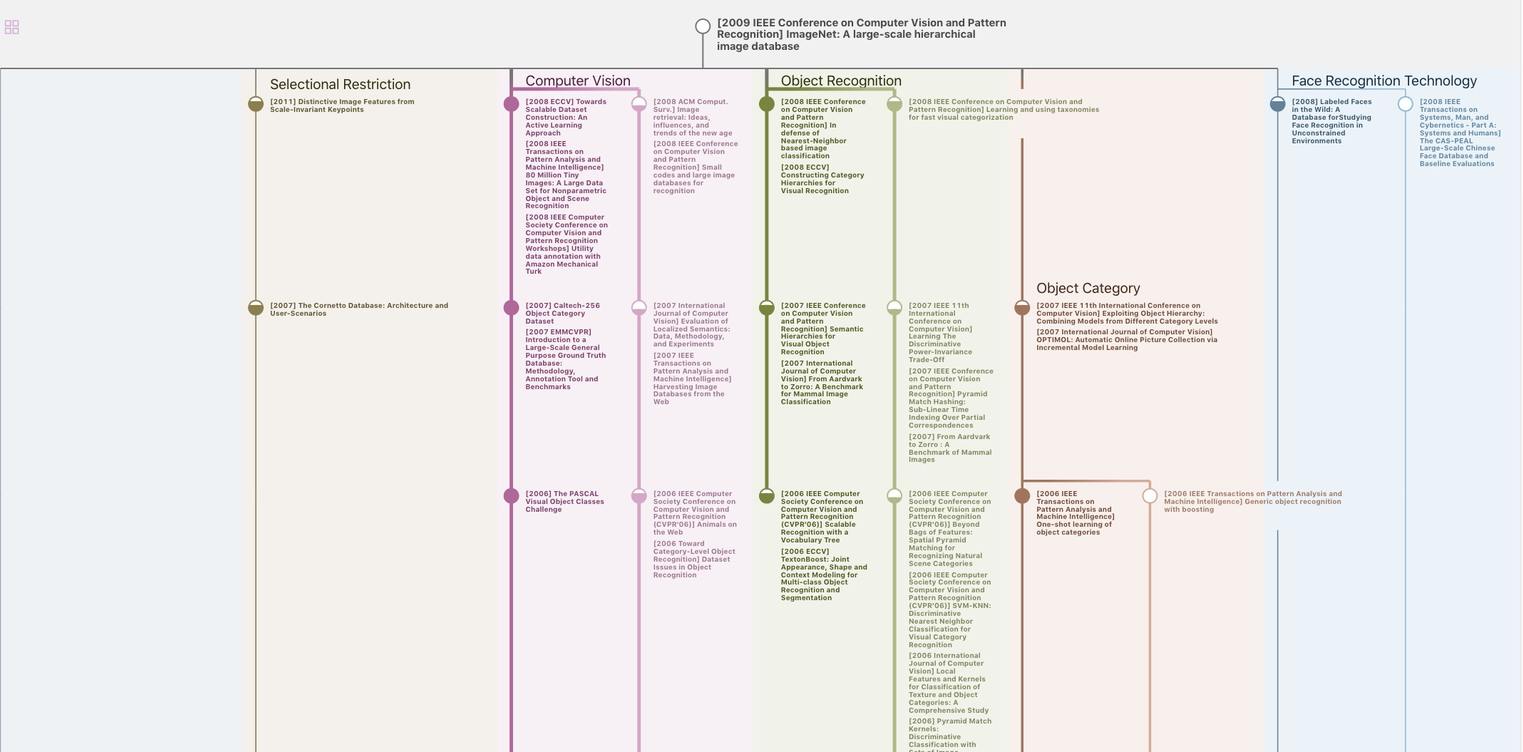
生成溯源树,研究论文发展脉络
Chat Paper
正在生成论文摘要