Monotonicity-based regularization for shape reconstruction in linear elasticity
Computational Mechanics(2022)
摘要
We deal with the shape reconstruction of inclusions in elastic bodies. For solving this inverse problem in practice, data fitting functionals are used. Those work better than the rigorous monotonicity methods from Eberle and Harrach (Inverse Probl 37(4):045006, 2021), but have no rigorously proven convergence theory. Therefore we show how the monotonicity methods can be converted into a regularization method for a data-fitting functional without losing the convergence properties of the monotonicity methods. This is a great advantage and a significant improvement over standard regularization techniques. In more detail, we introduce constraints on the minimization problem of the residual based on the monotonicity methods and prove the existence and uniqueness of a minimizer as well as the convergence of the method for noisy data. In addition, we compare numerical reconstructions of inclusions based on the monotonicity-based regularization with a standard approach (one-step linearization with Tikhonov-like regularization), which also shows the robustness of our method regarding noise in practice.
更多查看译文
关键词
Linear elasticity, Inverse problem, Shape reconstruction, One-step linearization method, Monotonicity-based regularization, 35R30, 65M32
AI 理解论文
溯源树
样例
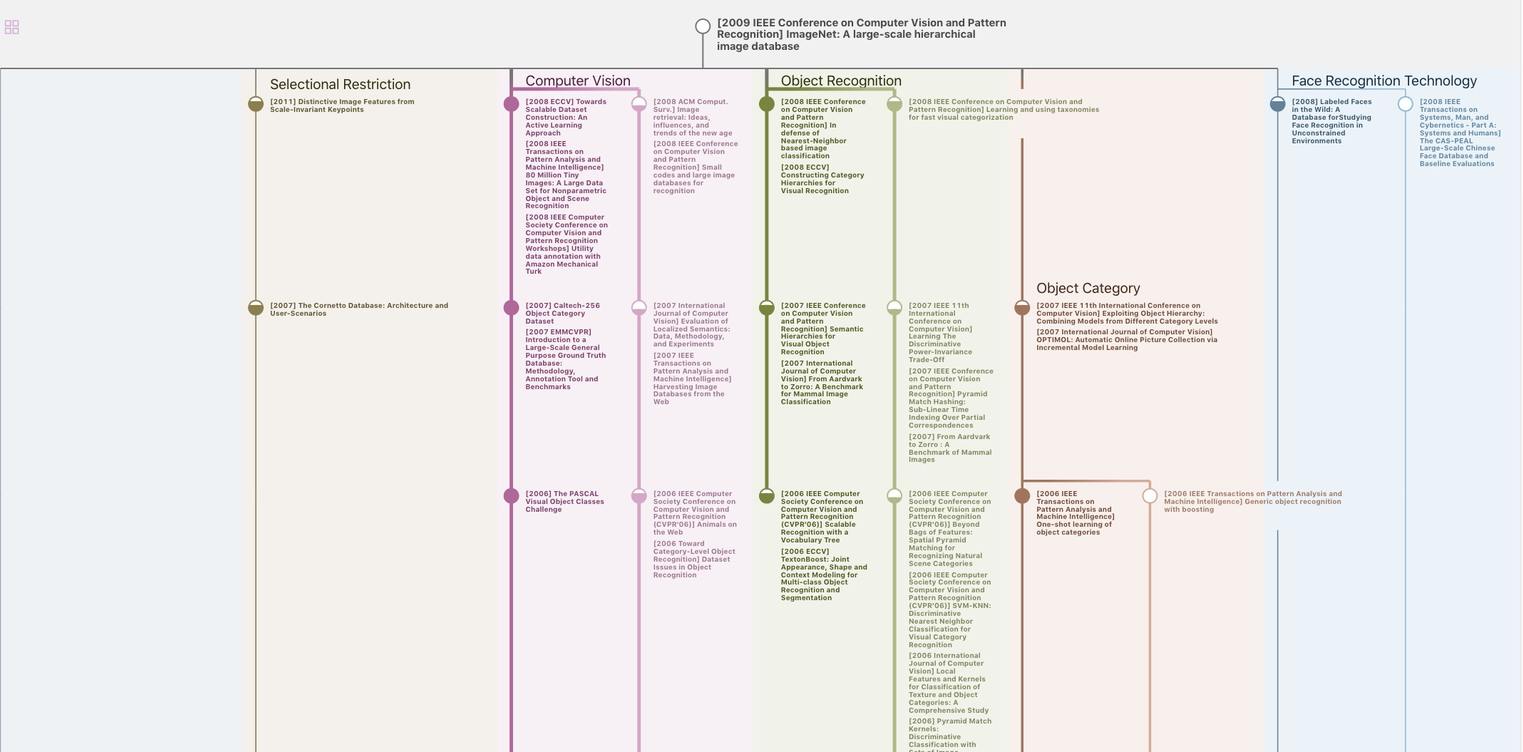
生成溯源树,研究论文发展脉络
Chat Paper
正在生成论文摘要