Design Of Nonlinear Autoregressive Exogenous Model Based Intelligence Computing For Efficient State Estimation Of Underwater Passive Target
ENTROPY(2021)
摘要
In this study, an intelligent computing paradigm built on a nonlinear autoregressive exogenous (NARX) feedback neural network model with the strength of deep learning is presented for accurate state estimation of an underwater passive target. In underwater scenarios, real-time motion parameters of passive objects are usually extracted with nonlinear filtering techniques. In filtering algorithms, nonlinear passive measurements are associated with linear kinetics of the target, governing by state space methodology. To improve tracking accuracy, effective feature estimation and minimizing position error of dynamic passive objects, the strength of NARX based supervised learning is exploited. Dynamic artificial neural networks, which contain tapped delay lines, are suitable for predicting the future state of the underwater passive object. Neural networks-based intelligence computing is effectively applied for estimating the real-time actual state of a passive moving object, which follows a semi-curved path. Performance analysis of NARX based neural networks is evaluated for six different scenarios of standard deviation of white Gaussian measurement noise by following bearings only tracking phenomena. Root mean square error between estimated and real position of the passive target in rectangular coordinates is computed for evaluating the worth of the proposed NARX feedback neural network scheme. The Monte Carlo simulations are conducted and the results certify the capability of the intelligence computing over conventional nonlinear filtering algorithms such as spherical radial cubature Kalman filter and unscented Kalman filter for given state estimation model.
更多查看译文
关键词
nonlinear autoregressive with exogenous input (NARX), state estimation, artificial neural network, measurement noise, nonlinear filtering, intelligent computing
AI 理解论文
溯源树
样例
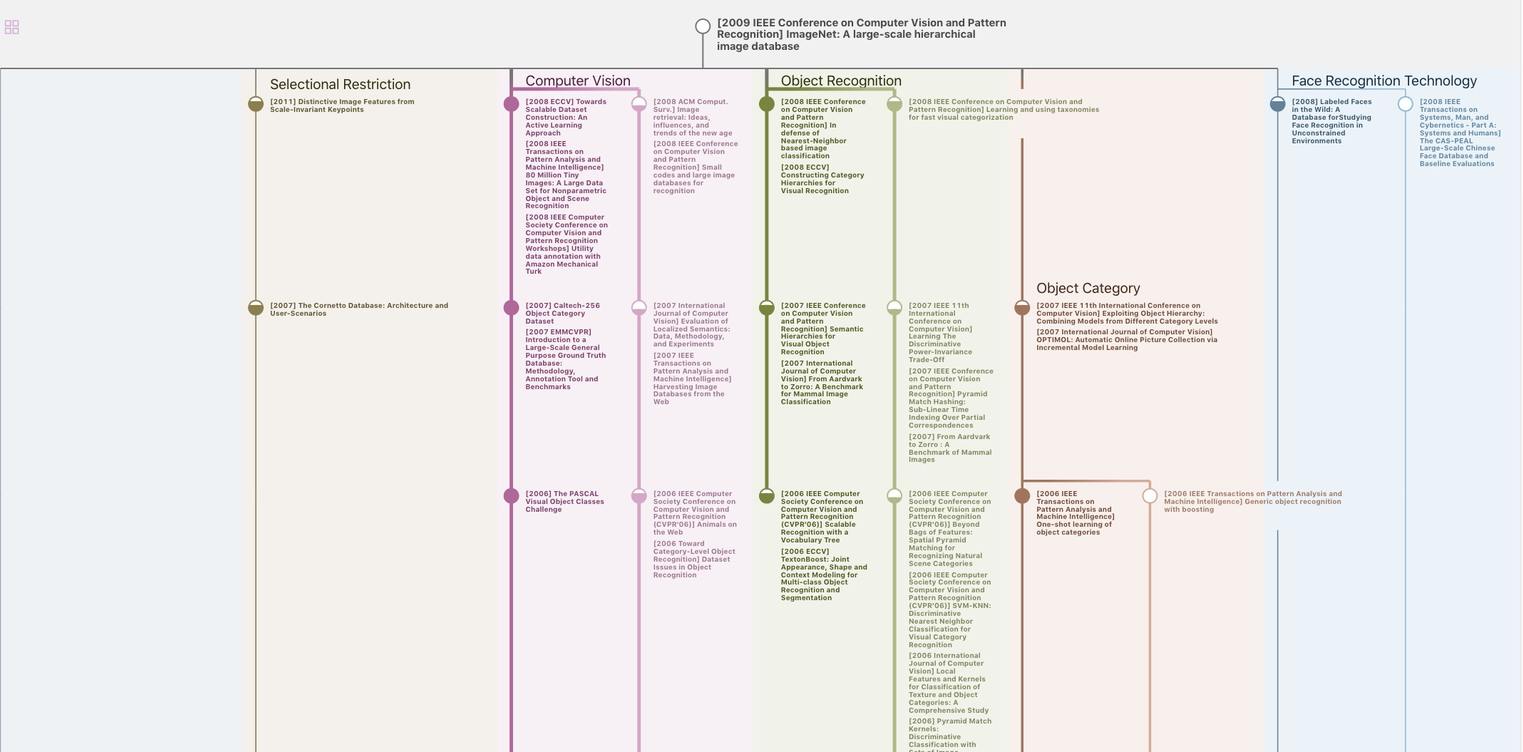
生成溯源树,研究论文发展脉络
Chat Paper
正在生成论文摘要