Surrogate Assisted Semi-supervised Inference for High Dimensional Risk Prediction
JOURNAL OF MACHINE LEARNING RESEARCH(2023)
摘要
Risk modeling with electronic health records (EHR) data is challenging due to no direct observations of the disease outcome and the high-dimensional predictors. In this paper, we develop a surrogate assisted semi-supervised learning approach, leveraging small labeled data with annotated outcomes and extensive unlabeled data of outcome surrogates and high-dimensional predictors. We propose to impute the unobserved outcomes by construct-ing a sparse imputation model with outcome surrogates and high-dimensional predictors. We further conduct a one-step bias correction to enable interval estimation for the risk prediction. Our inference procedure is valid even if both the imputation and risk pre-diction models are misspecified. Our novel way of ultilizing unlabelled data enables the high-dimensional statistical inference for the challenging setting with a dense risk predic-tion model. We present an extensive simulation study to demonstrate the superiority of our approach compared to existing supervised methods. We apply the method to genetic risk prediction of type-2 diabetes mellitus using an EHR biobank cohort.
更多查看译文
关键词
generalized linear models,high dimensional inference,model mis-specification,risk prediction,semi-supervised learning
AI 理解论文
溯源树
样例
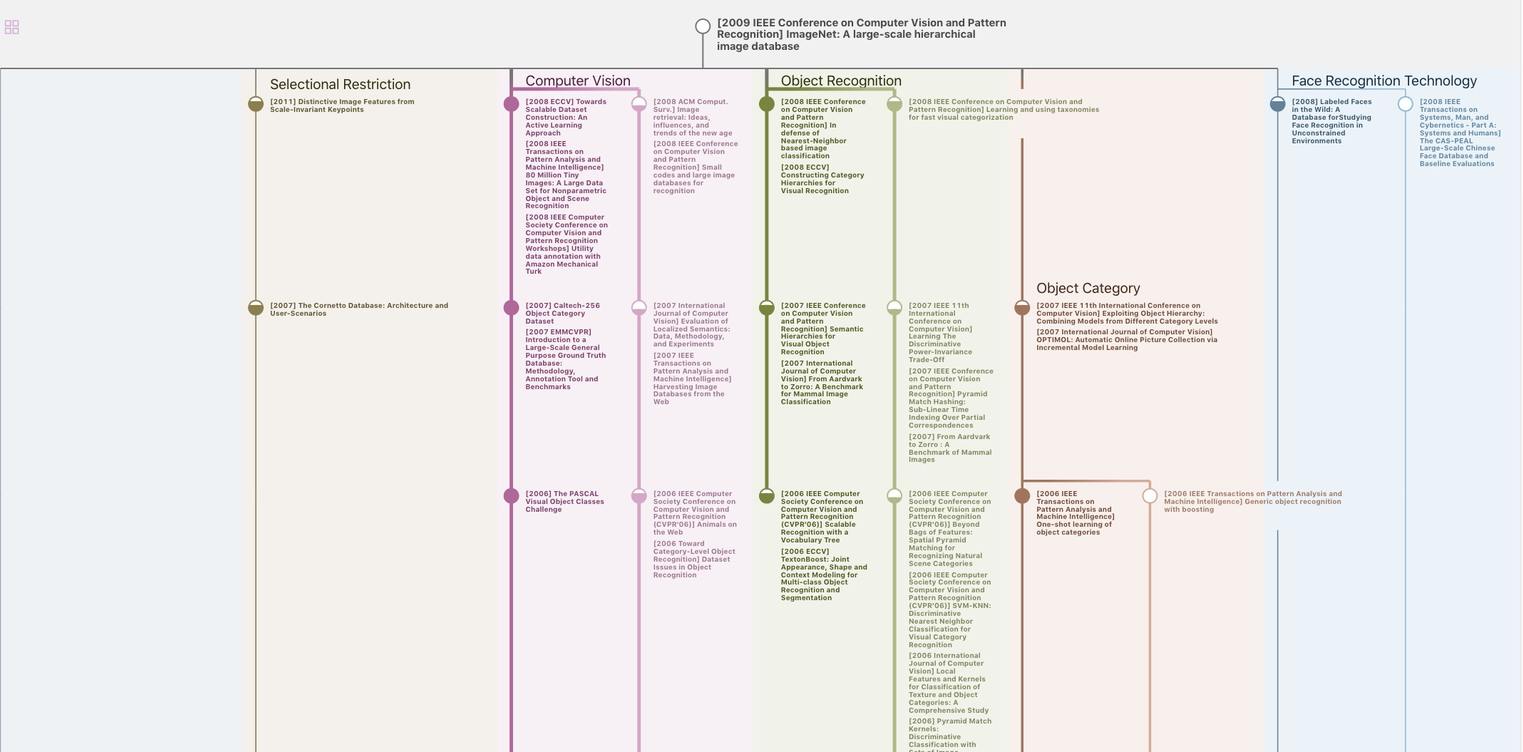
生成溯源树,研究论文发展脉络
Chat Paper
正在生成论文摘要