Weakly-Supervised Universal Lesion Segmentation with Regional Level Set Loss
MEDICAL IMAGE COMPUTING AND COMPUTER ASSISTED INTERVENTION - MICCAI 2021, PT II(2021)
Abstract
Accurately segmenting a variety of clinically significant lesions from whole body computed tomography (CT) scans is a critical task on precision oncology imaging, denoted as universal lesion segmentation (ULS). Manual annotation is the current clinical practice, being highly time-consuming and inconsistent on tumor's longitudinal assessment. Effectively training an automatic segmentation model is desirable but relies heavily on a large number of pixel-wise labelled data. Existing weakly-supervised segmentation approaches often struggle with regions nearby the lesion boundaries. In this paper, we present a novel weakly-supervised universal lesion segmentation method by building an attention enhanced model based on the High-Resolution Network (HRNet), named AHRNet, and propose a regional level set (RLS) loss for optimizing lesion boundary delineation. AHRNet provides advanced highresolution deep image features by involving a decoder, dual-attention and scale attention mechanisms, which are crucial to performing accurate lesion segmentation. RLS can optimize the model reliably and effectively in a weakly-supervised fashion, forcing the segmentation close to lesion boundary. Extensive experimental results demonstrate that our method achieves the best performance on the publicly large-scale DeepLesion dataset and a hold-out test set.
MoreTranslated text
Key words
universal lesion segmentation,weakly-supervised
AI Read Science
Must-Reading Tree
Example
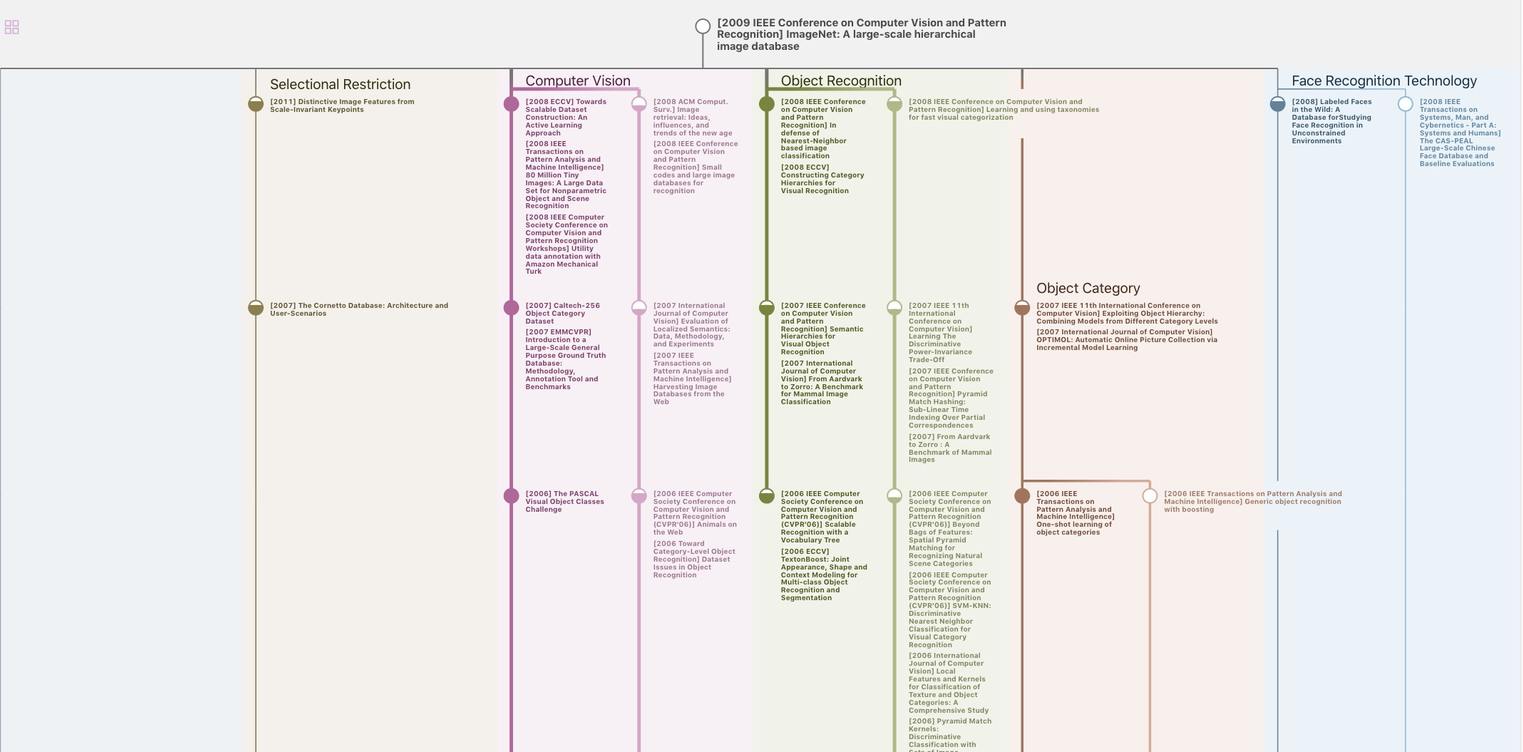
Generate MRT to find the research sequence of this paper
Chat Paper
Summary is being generated by the instructions you defined