Discovering diverse athletic jumping strategies
ACM Transactions on Graphics(2021)
摘要
AbstractWe present a framework that enables the discovery of diverse and natural-looking motion strategies for athletic skills such as the high jump. The strategies are realized as control policies for physics-based characters. Given a task objective and an initial character configuration, the combination of physics simulation and deep reinforcement learning (DRL) provides a suitable starting point for automatic control policy training. To facilitate the learning of realistic human motions, we propose a Pose Variational Autoencoder (P-VAE) to constrain the actions to a subspace of natural poses. In contrast to motion imitation methods, a rich variety of novel strategies can naturally emerge by exploring initial character states through a sample-efficient Bayesian diversity search (BDS) algorithm. A second stage of optimization that encourages novel policies can further enrich the unique strategies discovered. Our method allows for the discovery of diverse and novel strategies for athletic jumping motions such as high jumps and obstacle jumps with no motion examples and less reward engineering than prior work.
更多查看译文
关键词
physics-based character animation, motion strategy, Bayesian optimization, control policy
AI 理解论文
溯源树
样例
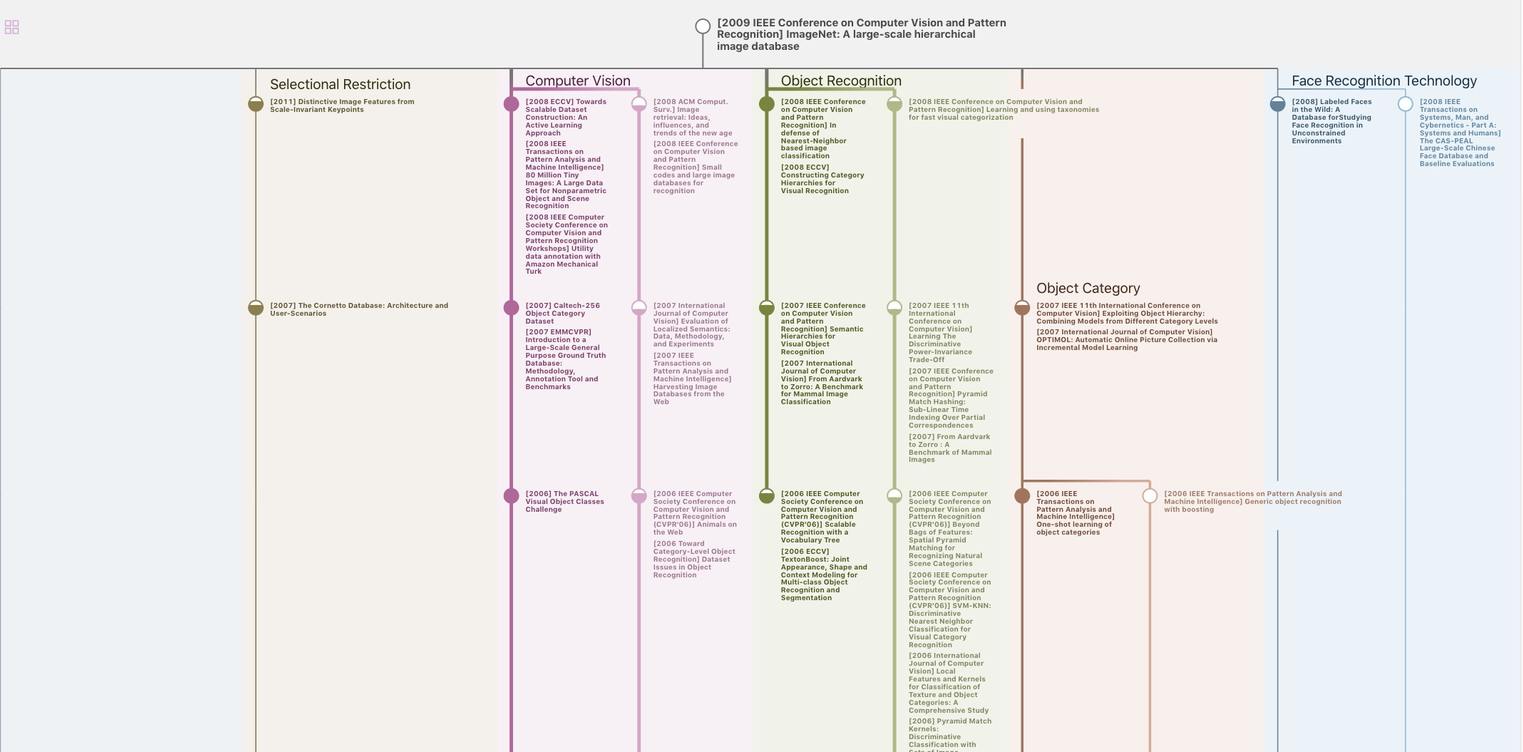
生成溯源树,研究论文发展脉络
Chat Paper
正在生成论文摘要