Stochastic Block-ADMM for Training Deep Networks
arxiv(2021)
摘要
In this paper, we propose Stochastic Block-ADMM as an approach to train deep neural networks in batch and online settings. Our method works by splitting neural networks into an arbitrary number of blocks and utilizes auxiliary variables to connect these blocks while optimizing with stochastic gradient descent. This allows training deep networks with non-differentiable constraints where conventional backpropagation is not applicable. An application of this is supervised feature disentangling, where our proposed DeepFacto inserts a non-negative matrix factorization (NMF) layer into the network. Since backpropagation only needs to be performed within each block, our approach alleviates vanishing gradients and provides potentials for parallelization. We prove the convergence of our proposed method and justify its capabilities through experiments in supervised and weakly-supervised settings.
更多查看译文
关键词
training deep networks,stochastic,block-admm
AI 理解论文
溯源树
样例
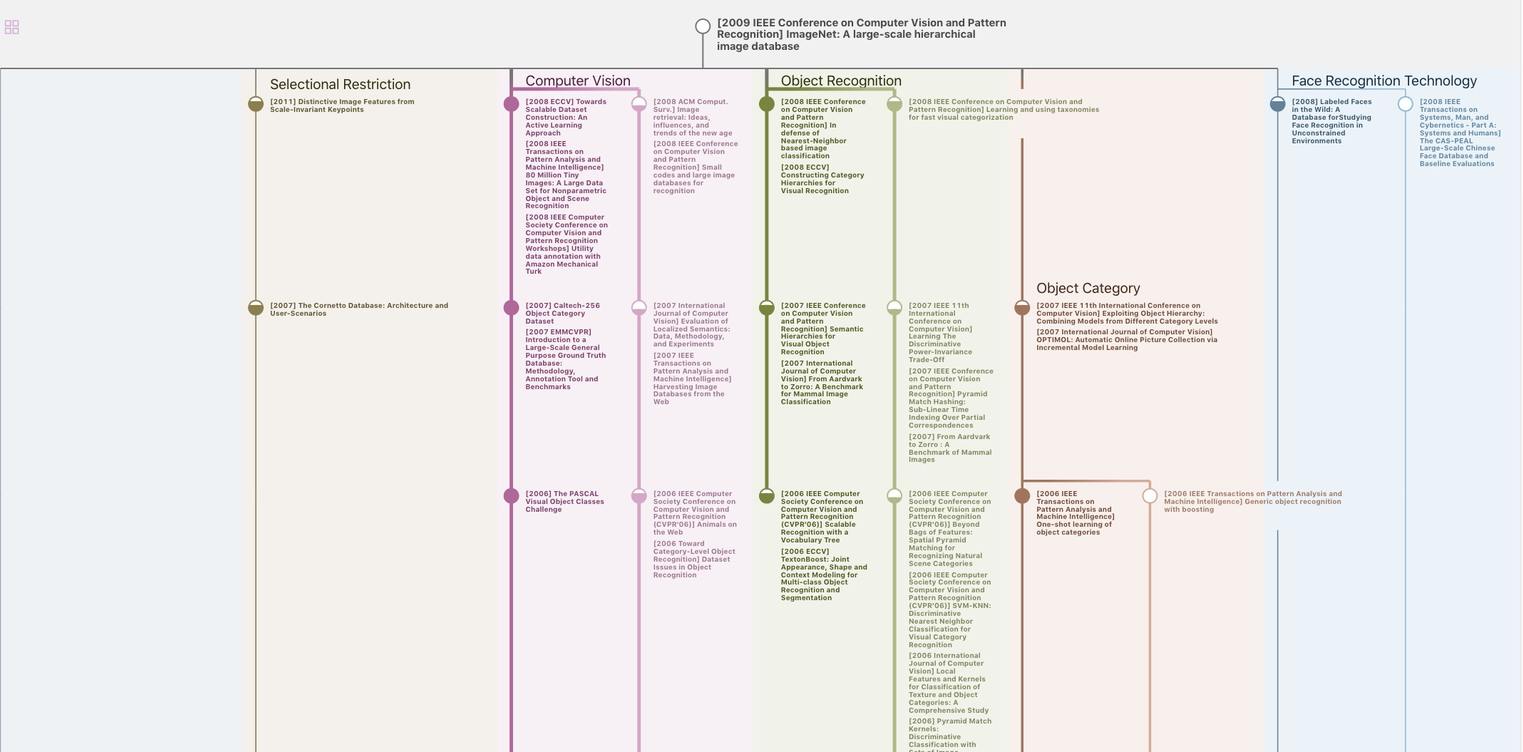
生成溯源树,研究论文发展脉络
Chat Paper
正在生成论文摘要