Scaling End-to-End Models for Large-Scale Multilingual ASR
2021 IEEE AUTOMATIC SPEECH RECOGNITION AND UNDERSTANDING WORKSHOP (ASRU)(2021)
摘要
Building ASR models across many languages is a challenging multi-task learning problem due to large variations and heavily unbalanced data. Existing work has shown positive transfer from high resource to low resource languages. However, degradations on high resource languages are commonly observed due to interference from the heterogeneous multilingual data and reduction in per-language capacity. We conduct a capacity study on a 15-language task, with the amount of data per language varying from 7.6K to 53.5K hours. We adopt GShard [1] to efficiently scale up to 10B parameters. Empirically, we find that (1) scaling the number of model parameters is an effective way to solve the capacity bottleneck - our 500M-param model already outperforms monolingual baselines and scaling it to 1B and 10B brought further quality gains; (2) larger models are not only more data efficient, but also more efficient in terms of training cost as measured in TPU days - the 1B-param model reaches the same accuracy at 34% of training time as the 500M-param model; (3) given a fixed capacity budget, adding depth works better than width and large encoders do better than large decoders; (4) with continuous training, they can be adapted to new languages and domains.
更多查看译文
关键词
large-scale, multilingual speech recognition
AI 理解论文
溯源树
样例
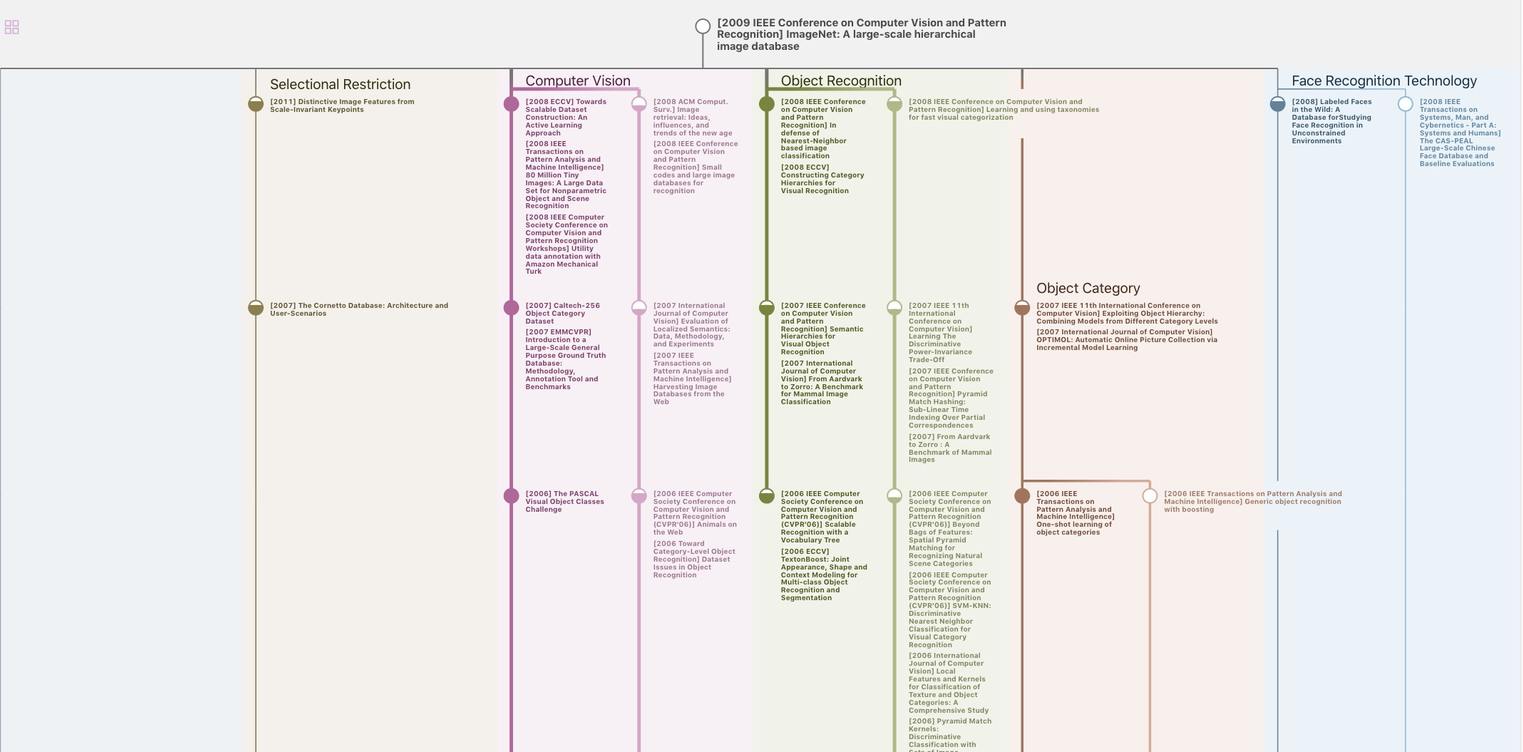
生成溯源树,研究论文发展脉络
Chat Paper
正在生成论文摘要