Optimising The Use Of Gene Expression Data To Predict Plant Metabolic Pathway Memberships
NEW PHYTOLOGIST(2021)
摘要
Plant metabolites from diverse pathways are important for plant survival, human nutrition and medicine. The pathway memberships of most plant enzyme genes are unknown. While co-expression is useful for assigning genes to pathways, expression correlation may exist only under specific spatiotemporal and conditional contexts.Utilising > 600 tomato (Solanum lycopersicum) expression data combinations, three strategies for predicting memberships in 85 pathways were explored.Optimal predictions for different pathways require distinct data combinations indicative of pathway functions. Naive prediction (i.e. identifying pathways with the most similarly expressed genes) is error prone. In 52 pathways, unsupervised learning performed better than supervised approaches, possibly due to limited training data availability. Using gene-to-pathway expression similarities led to prediction models that outperformed those based simply on expression levels. Using 36 experimental validated genes, the pathway-best model prediction accuracy is 58.3%, significantly better compared with that for predicting annotated genes without experimental evidence (37.0%) or random guess (1.2%), demonstrating the importance of data quality.Our study highlights the need to extensively explore expression-based features and prediction strategies to maximise the accuracy of metabolic pathway membership assignment. The prediction framework outlined here can be applied to other species and serves as a baseline model for future comparisons.
更多查看译文
关键词
gene expression, machine learning, metabolic pathway, prediction, tomato
AI 理解论文
溯源树
样例
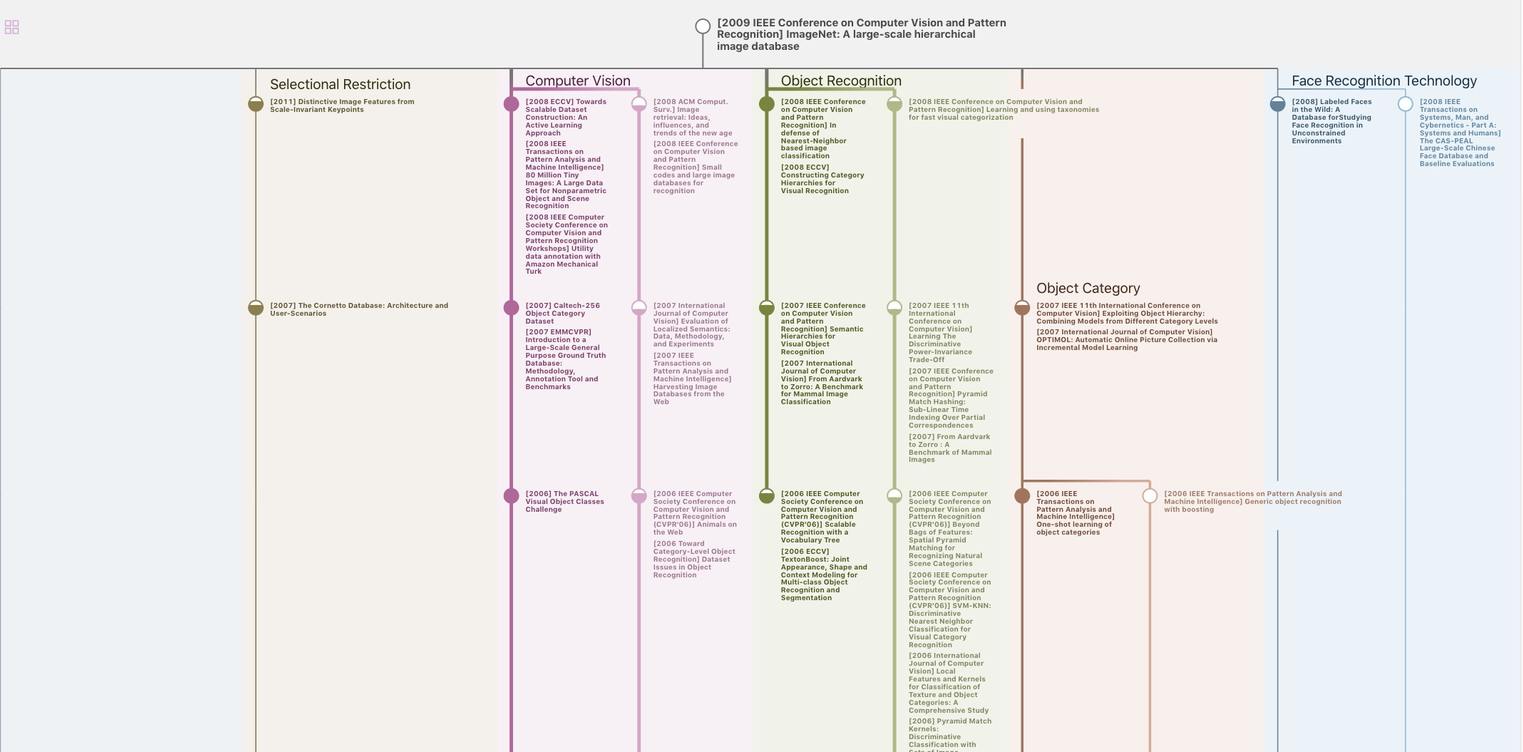
生成溯源树,研究论文发展脉络
Chat Paper
正在生成论文摘要