Classification Of Prostate Transitional Zone Cancer And Hyperplasia Using Deep Transfer Learning From Disease-Related Images
CUREUS(2021)
摘要
PurposeThe diagnosis of prostate transition zone cancer (PTZC) remains a clinical challenge due to their similarity to benign prostatic hyperplasia (BPH) on MRI. The Deep Convolutional Neural Networks (DCNNs) showed high efficacy in diagnosing PTZC on medical imaging but was limited by the small data size. A transfer learning (TL) method was combined with deep learning to overcome this challenge.Materials and methodsA retrospective investigation was conducted on 217 patients enrolled from our hospital database (208 patients) and The Cancer Imaging Archive (nine patients). Using T2-weighted images (T2WIs) and apparent diffusion coefficient (ADC) maps, DCNN models were trained and compared between different TL databases (ImageNet vs. disease-related images) and protocols (from scratch, fine-tuning, or transductive transferring).ResultsPTZC and BPH can be classified through traditional DCNN. The efficacy of TL from natural images was limited but improved by transferring knowledge from the disease-related images. Furthermore, transductive TL from disease-related images had comparable efficacy to the fine-tuning method. Limitations include retrospective design and a relatively small sample size.ConclusionDeep TL from disease-related images is a powerful tool for an automated PTZC diagnostic system. In developing regions where only conventional MR scans are available, the accurate diagnosis of PTZC can be achieved via transductive deep TL from disease-related images.
更多查看译文
关键词
deep learning, transfer learning, magnetic resonance imaging (mri), prostate cancer
AI 理解论文
溯源树
样例
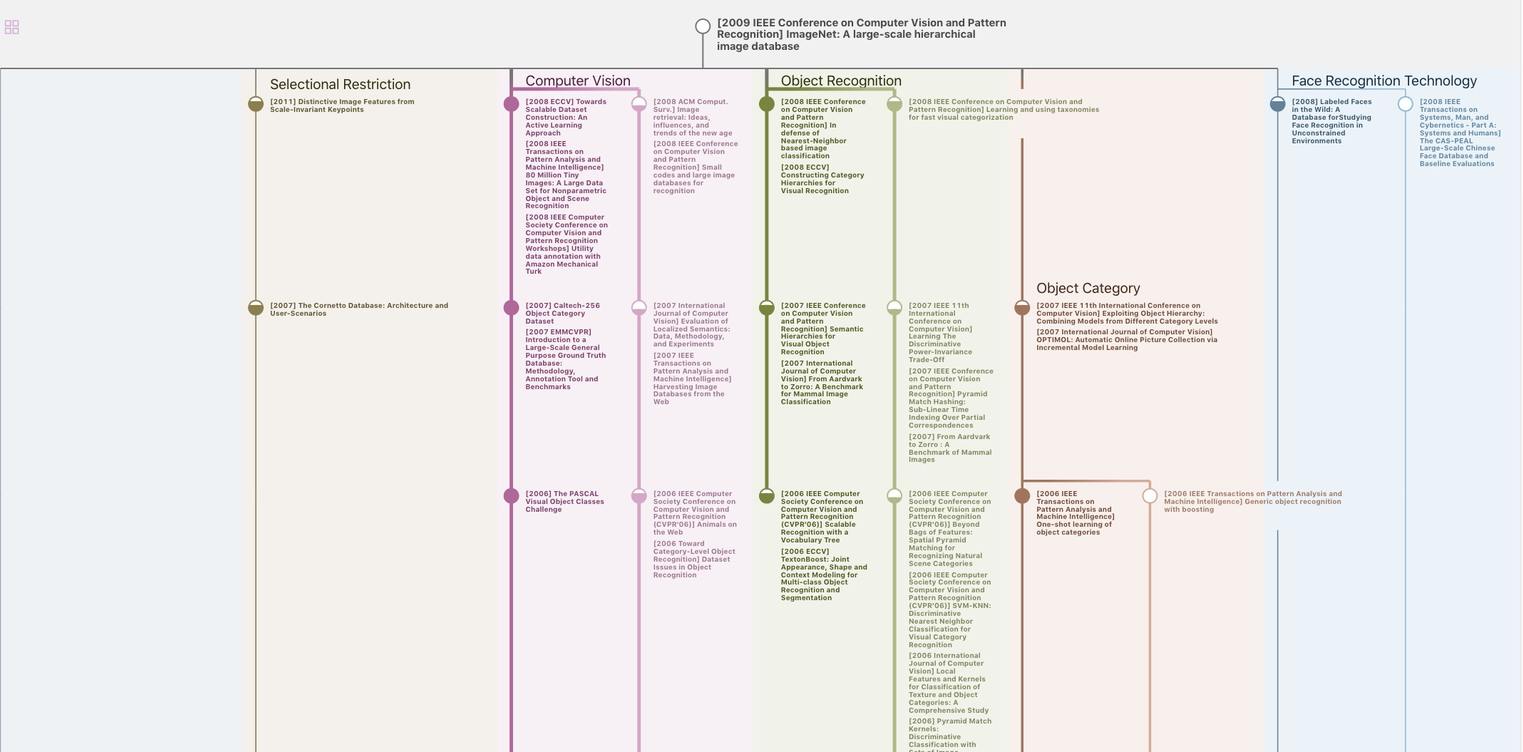
生成溯源树,研究论文发展脉络
Chat Paper
正在生成论文摘要