Single-Trial Kernel-Based Functional Connectivity For Enhanced Feature Extraction In Motor-Related Tasks
SENSORS(2021)
摘要
Motor learning is associated with functional brain plasticity, involving specific functional connectivity changes in the neural networks. However, the degree of learning new motor skills varies among individuals, which is mainly due to the between-subject variability in brain structure and function captured by electroencephalographic (EEG) recordings. Here, we propose a kernel-based functional connectivity measure to deal with inter/intra-subject variability in motor-related tasks. To this end, from spatio-temporal-frequency patterns, we extract the functional connectivity between EEG channels through their Gaussian kernel cross-spectral distribution. Further, we optimize the spectral combination weights within a sparse-based l2-norm feature selection framework matching the motor-related labels that perform the dimensionality reduction of the extracted connectivity features. From the validation results in three databases with motor imagery and motor execution tasks, we conclude that the single-trial Gaussian functional connectivity measure provides very competitive classifier performance values, being less affected by feature extraction parameters, like the sliding time window, and avoiding the use of prior linear spatial filtering. We also provide interpretability for the clustered functional connectivity patterns and hypothesize that the proposed kernel-based metric is promising for evaluating motor skills.
更多查看译文
关键词
functional connectivity, Gaussian kernel, motor imagery, motor execution
AI 理解论文
溯源树
样例
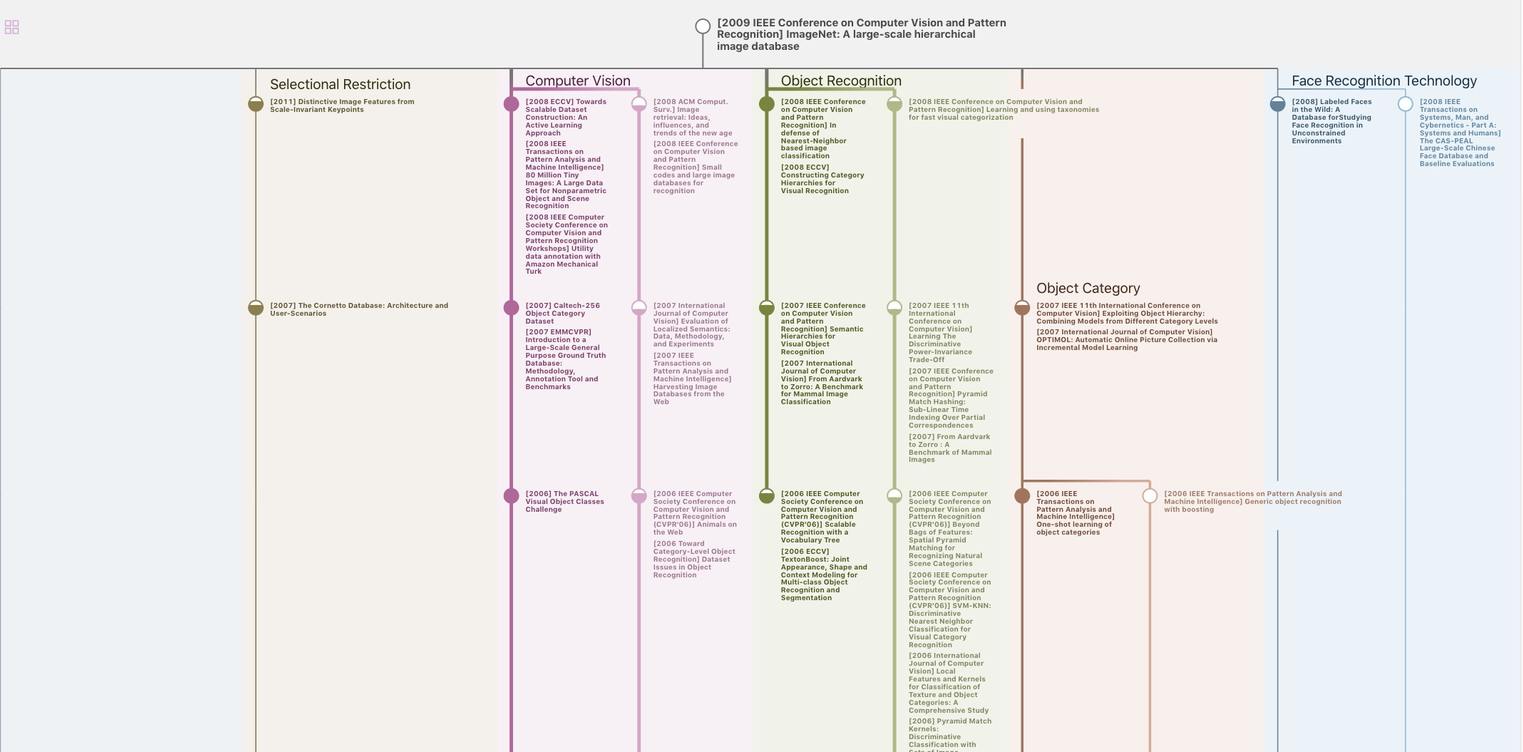
生成溯源树,研究论文发展脉络
Chat Paper
正在生成论文摘要