A Large-Scale Study on Unsupervised Spatiotemporal Representation Learning
2021 IEEE/CVF CONFERENCE ON COMPUTER VISION AND PATTERN RECOGNITION, CVPR 2021(2021)
摘要
We present a large-scale study on unsupervised spatiotemporal representation learning from videos. With a unified perspective on four recent image-based frameworks, we study a simple objective that can easily generalize all these methods to space-time. Our objective encourages temporally-persistent features in the same video, and in spite of its simplicity, it works surprisingly well across: (i) different unsupervised frameworks, (ii) pre-training datasets, (iii) downstream datasets, and (iv) backbone architectures. We draw a series of intriguing observations from this study, e.g., we discover that encouraging long-spanned persistency can be effective even if the timespan is 60 seconds. In addition to state-of-the-art results in multiple benchmarks, we report a few promising cases in which unsupervised pre-training can outperform its supervised counterpart.
更多查看译文
关键词
unsupervised spatiotemporal representation
AI 理解论文
溯源树
样例
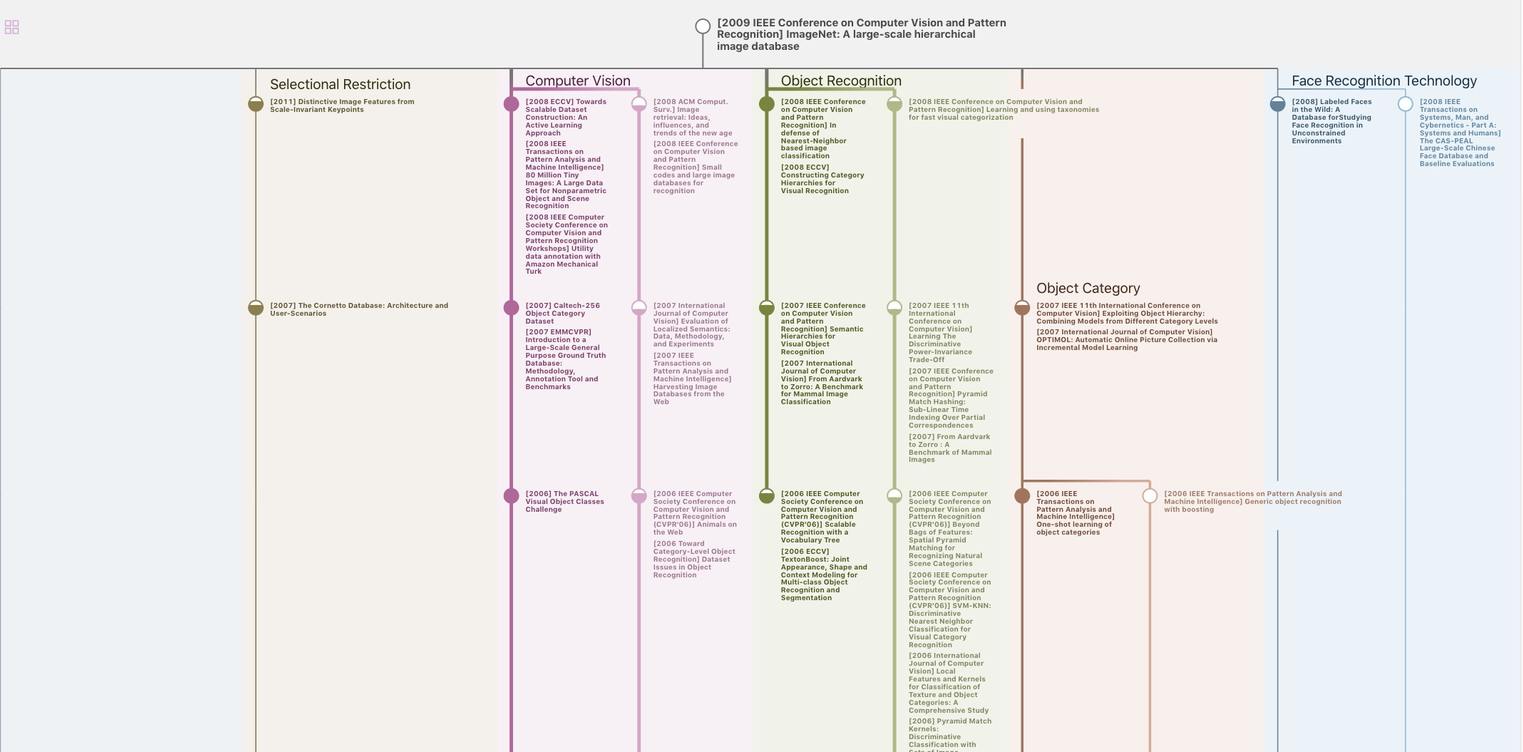
生成溯源树,研究论文发展脉络
Chat Paper
正在生成论文摘要