Histopathological characteristics and artificial intelligence for predicting tumor mutational burden-high colorectal cancer
JOURNAL OF GASTROENTEROLOGY(2021)
摘要
Background Tumor mutational burden-high (TMB-H), which is detected with gene panel testing, is a promising biomarker for immune checkpoint inhibitors (ICIs) in colorectal cancer (CRC). However, in clinical practice, not every patient is tested for TMB-H using gene panel testing. We aimed to identify the histopathological characteristics of TMB-H CRC for efficient selection of patients who should undergo gene panel testing. Moreover, we attempted to develop a convolutional neural network (CNN)-based algorithm to predict TMB-H CRC directly from hematoxylin and eosin (H&E) slides. Methods We used two CRC cohorts tested for TMB-H, and whole-slide H&E digital images were obtained from the cohorts. The Japanese CRC (JP-CRC) cohort ( N = 201) was evaluated to detect the histopathological characteristics of TMB-H using H&E slides. The JP-CRC cohort and The Cancer Genome Atlas (TCGA) CRC cohort ( N = 77) were used to develop a CNN-based TMB-H prediction model from the H&E digital images. Results Tumor-infiltrating lymphocytes (TILs) were significantly associated with TMB-H CRC ( P < 0.001). The area under the curve (AUC) for predicting TMB-H CRC was 0.910. We developed a CNN-based TMB-H prediction model. Validation tests were conducted 10 times using randomly selected slides, and the average AUC for predicting TMB-H slides was 0.934. Conclusions TILs, a histopathological characteristic detected with H&E slides, are associated with TMB-H CRC. Our CNN-based model has the potential to predict TMB-H CRC directly from H&E slides, thereby reducing the burden on pathologists. These approaches will provide clinicians with important information about the applications of ICIs at low cost.
更多查看译文
关键词
Gene panel testing,Immune checkpoint inhibitors,Convolutional neural networks,Digital pathology
AI 理解论文
溯源树
样例
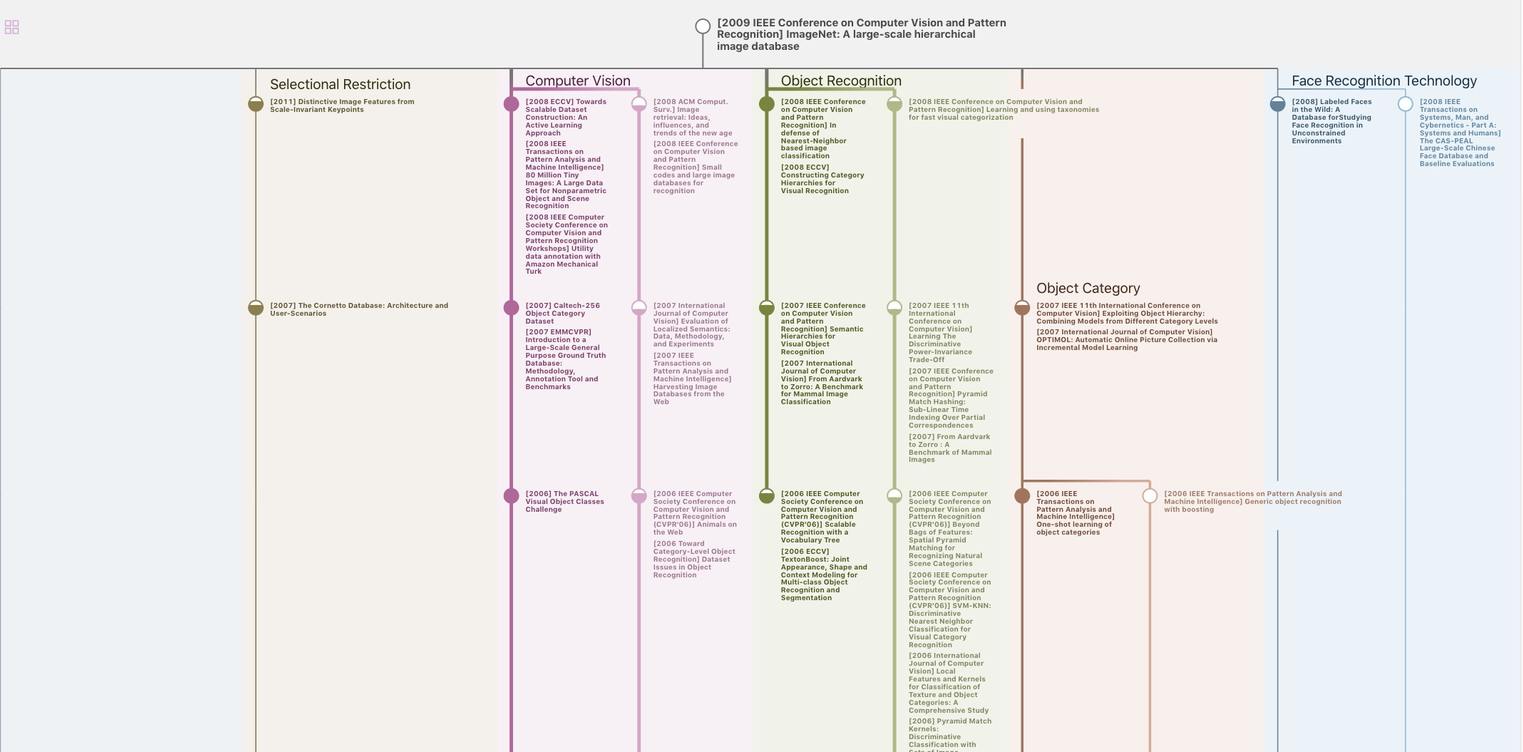
生成溯源树,研究论文发展脉络
Chat Paper
正在生成论文摘要