Semi-On-Policy Training for Sample Efficient Multi-Agent Policy Gradients
CoRR(2021)
摘要
Policy gradient methods are an attractive approach to multi-agent reinforcement learning problems due to their convergence properties and robustness in partially observable scenarios. However, there is a significant performance gap between state-of-the-art policy gradient and value-based methods on the popular StarCraft Multi-Agent Challenge (SMAC) benchmark. In this paper, we introduce semi-on-policy (SOP) training as an effective and computationally efficient way to address the sample inefficiency of on-policy policy gradient methods. We enhance two state-of-the-art policy gradient algorithms with SOP training, demonstrating significant performance improvements. Furthermore, we show that our methods perform as well or better than state-of-the-art value-based methods on a variety of SMAC tasks.
更多查看译文
关键词
training,semi-on-policy,multi-agent
AI 理解论文
溯源树
样例
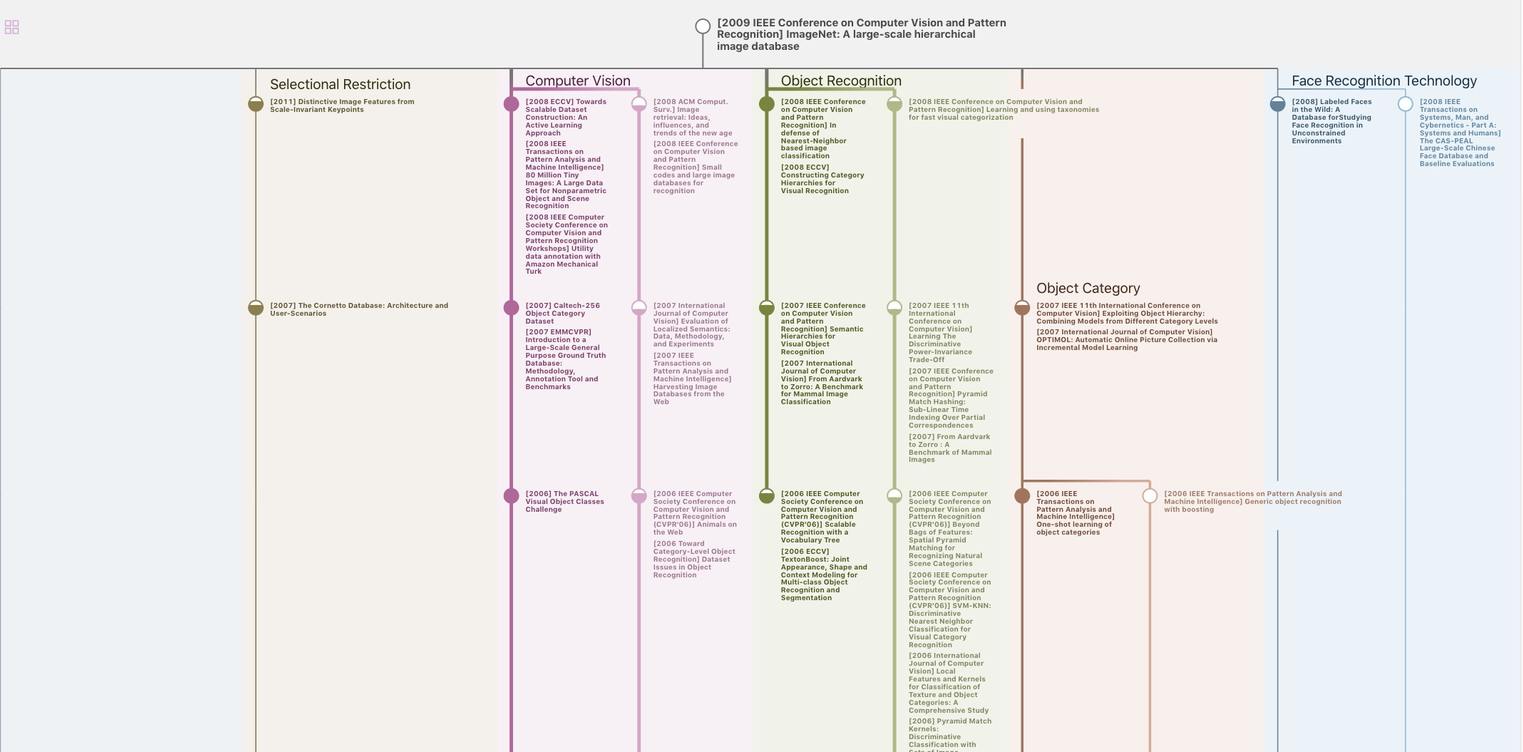
生成溯源树,研究论文发展脉络
Chat Paper
正在生成论文摘要