Evidential Segmentation of 3D PET/CT Images
BELIEF FUNCTIONS: THEORY AND APPLICATIONS (BELIEF 2021)(2021)
摘要
Positron Emission Tomography (PET) and Computed Tomography (CT) are two modalities widely used in medical image analysis. Accurately detecting and segmenting lymphomas from these two imaging modalities are critical tasks for cancer staging and radiotherapy planning. However, this task is still challenging due to the complexity of PET/CT images, and the computation cost to process 3D data. In this paper, a segmentation method based on belief functions is proposed to segment lymphomas in 3D PET/CT images. The architecture is composed of a feature extraction module and an evidential segmentation (ES) module. The ES module outputs not only segmentation results (binary maps indicating the presence or absence of lymphoma in each voxel) but also uncertainty maps quantifying the classification uncertainty. The whole model is optimized by minimizing Dice and uncertainty loss functions to increase segmentation accuracy. The method was evaluated on a database of 173 patients with diffuse large b-cell lymphoma. Quantitative and qualitative results show that our method outperforms the state-of-the-art methods.
更多查看译文
关键词
Lymphoma segmentation,3D PET/CT,Belief functions,Dempster-Shafer theory,Uncertainty quantification,Deep learning
AI 理解论文
溯源树
样例
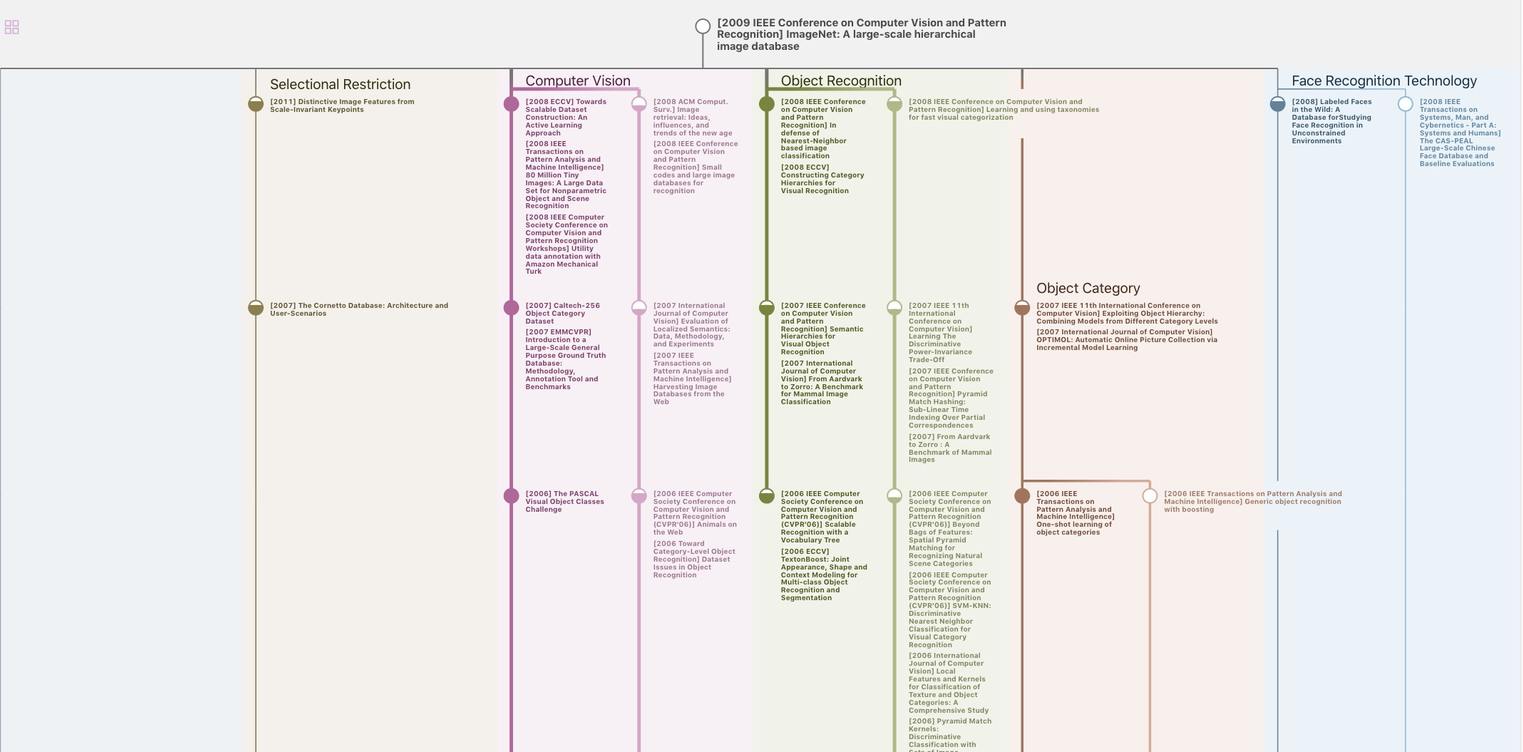
生成溯源树,研究论文发展脉络
Chat Paper
正在生成论文摘要