Collective Monte Carlo updates through tensor network renormalization
SciPost Physics(2023)
Abstract
We introduce a Metropolis-Hastings Markov chain for Boltzmann distributions of classical spin systems. It relies on approximate tensor network contractions to propose correlated collective updates at each step of the evolution. We present benchmark computations for a wide variety of instances of the two-dimensional Ising model, including ferromagnetic, antiferromagnetic, (fully) frustrated and Edwards-Anderson spin glass in-stances, and we show that, with modest computational effort, our Markov chain achieves sizeable acceptance rates, even in the vicinity of critical points. In each of the situations we have considered, the Markov chain compares well with other Monte Carlo schemes such as the Metropolis or Wolff's algorithm: equilibration times appear to be reduced by a factor that varies between 40 and 2000, depending on the model and the observable being monitored. We also present an extension to three spatial dimensions, and demonstrate that it exhibits fast equilibration for finite ferro-and antiferromagnetic instances. Additionally, and although it is originally designed for a square lattice of finite degrees of freedom with open boundary conditions, the proposed scheme can be used as such, or with slight modifications, to study triangular lattices, systems with continuous degrees of freedom, matrix models, a confined gas of hard spheres, or to deal with arbitrary boundary conditions.
MoreTranslated text
Key words
renormalization,collective monte carlo
AI Read Science
Must-Reading Tree
Example
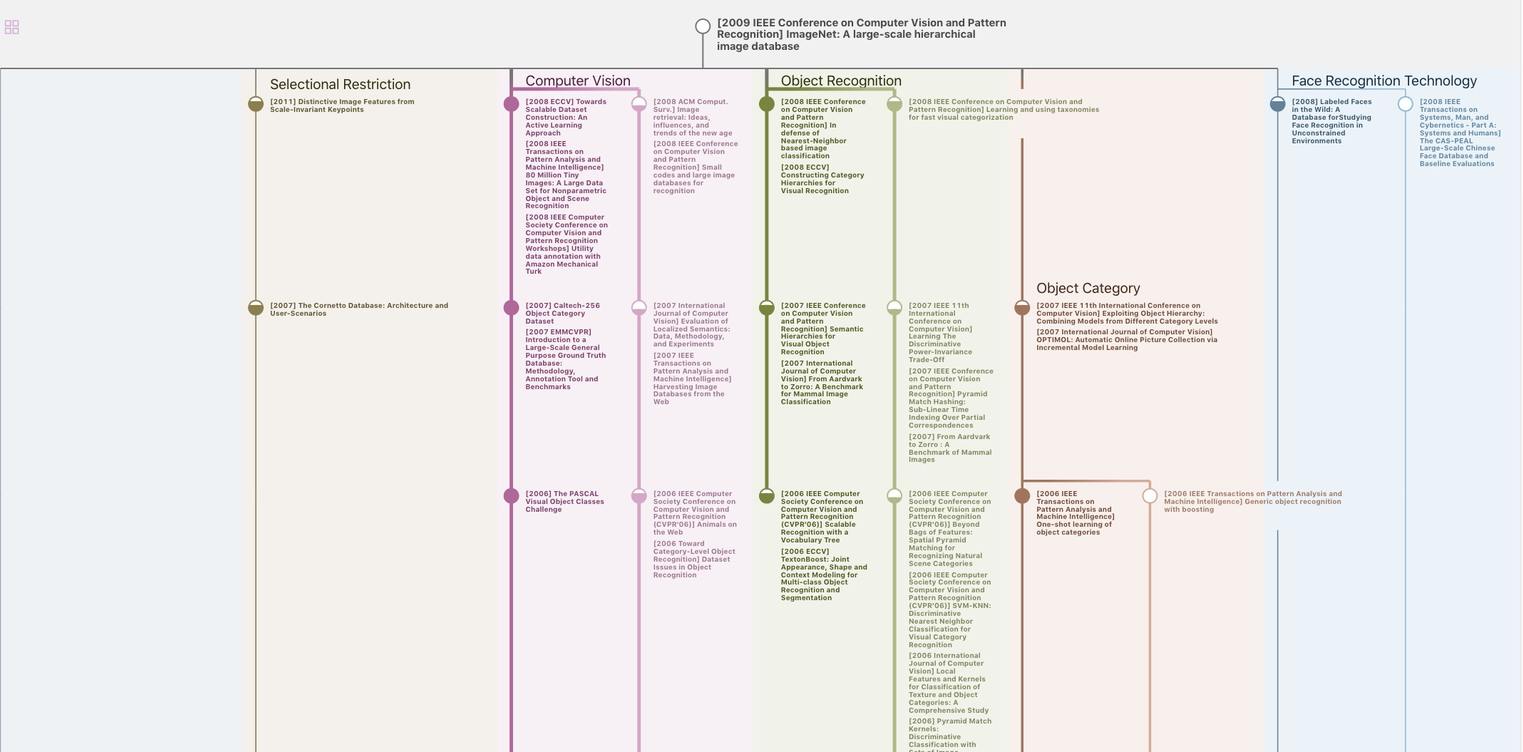
Generate MRT to find the research sequence of this paper
Chat Paper
Summary is being generated by the instructions you defined