Effective Connectivity in Default Mode Network for Alcoholism Diagnosis
IEEE Transactions on Neural Systems and Rehabilitation Engineering(2021)
摘要
Alcohol Use Disorder (AUD) is a chronic relapsing brain disease characterized by excessive alcohol use, loss of control over alcohol intake, and negative emotional states under no alcohol consumption. The key factor in successful treatment of AUD is the accurate diagnosis for better medical and therapy management. Conventionally, for individuals to be diagnosed with AUD, certain criteria as outlined in the Diagnostic and Statistical Manual of Mental Disorders (DSM) should be met. However, this process is subjective in nature and could be misleading due to memory problems and dishonesty of some AUD patients. In this paper, an assessment scheme for objective diagnosis of AUD is proposed. For this purpose, EEG recording of 31 healthy controls and 31 AUD patients are used for the calculation of effective connectivity (EC) between the various regions of the brain Default Mode Network (DMN). The EC is estimated using partial directed coherence (PDC) which are then used as input to a 3D Convolutional Neural Network (CNN) for binary classification of AUD cases. Using 5-fold cross validation, the classification of AUD vs. HC effective connectivity matrices using the proposed 3D-CNN gives an accuracy of 87.85 +/- 4.64 %. For further validation, 32 and 30 subjects are randomly selected for training and testing, respectively, giving 100% correct classification of all the testing subjects.
更多查看译文
关键词
Electroencephalography,Alcoholic beverages,Feature extraction,Alcoholism,Coherence,Electrodes,Synchronization,3D Convolutional neural networks,alcohol use disorder,alcoholism,default mode network,brain effective connectivity,deep learning,multivariate autoregressive models,partial directed coherence
AI 理解论文
溯源树
样例
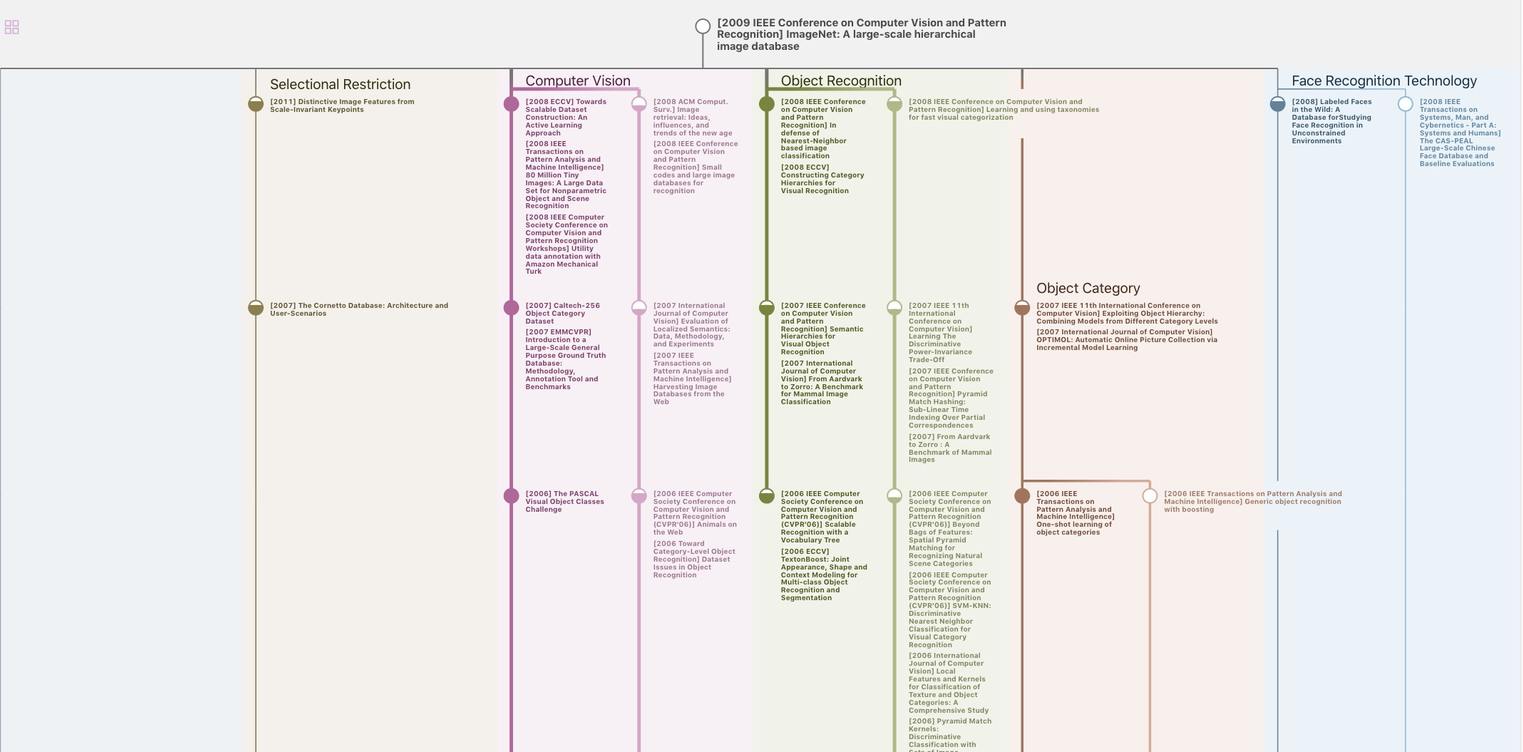
生成溯源树,研究论文发展脉络
Chat Paper
正在生成论文摘要