RubberBand: cloud-based hyperparameter tuning
EUROSYS(2021)
摘要
ABSTRACTHyperparameter tuning is essential to achieving state-of-the-art accuracy in machine learning (ML), but requires substantial compute resources to perform. Existing systems primarily focus on effectively allocating resources for a hyperparameter tuning job under fixed resource constraints. We show that the available parallelism in such jobs changes dynamically over the course of execution and, therefore, presents an opportunity to leverage the elasticity of the cloud. In particular, we address the problem of minimizing the financial cost of executing a hyperparameter tuning job, subject to a time constraint. We present RubberBand---the first framework for cost-efficient, elastic execution of hyperparameter tuning jobs in the cloud. RubberBand utilizes performance instrumentation and cloud pricing to model job completion time and cost prior to runtime, and generate a cost-efficient, elastic resource allocation plan. RubberBand is able to efficiently execute this plan and realize a cost reduction of up to 2x in comparison to static allocation baselines.
更多查看译文
关键词
Hyperparameter Optimization, Distributed Machine Learning
AI 理解论文
溯源树
样例
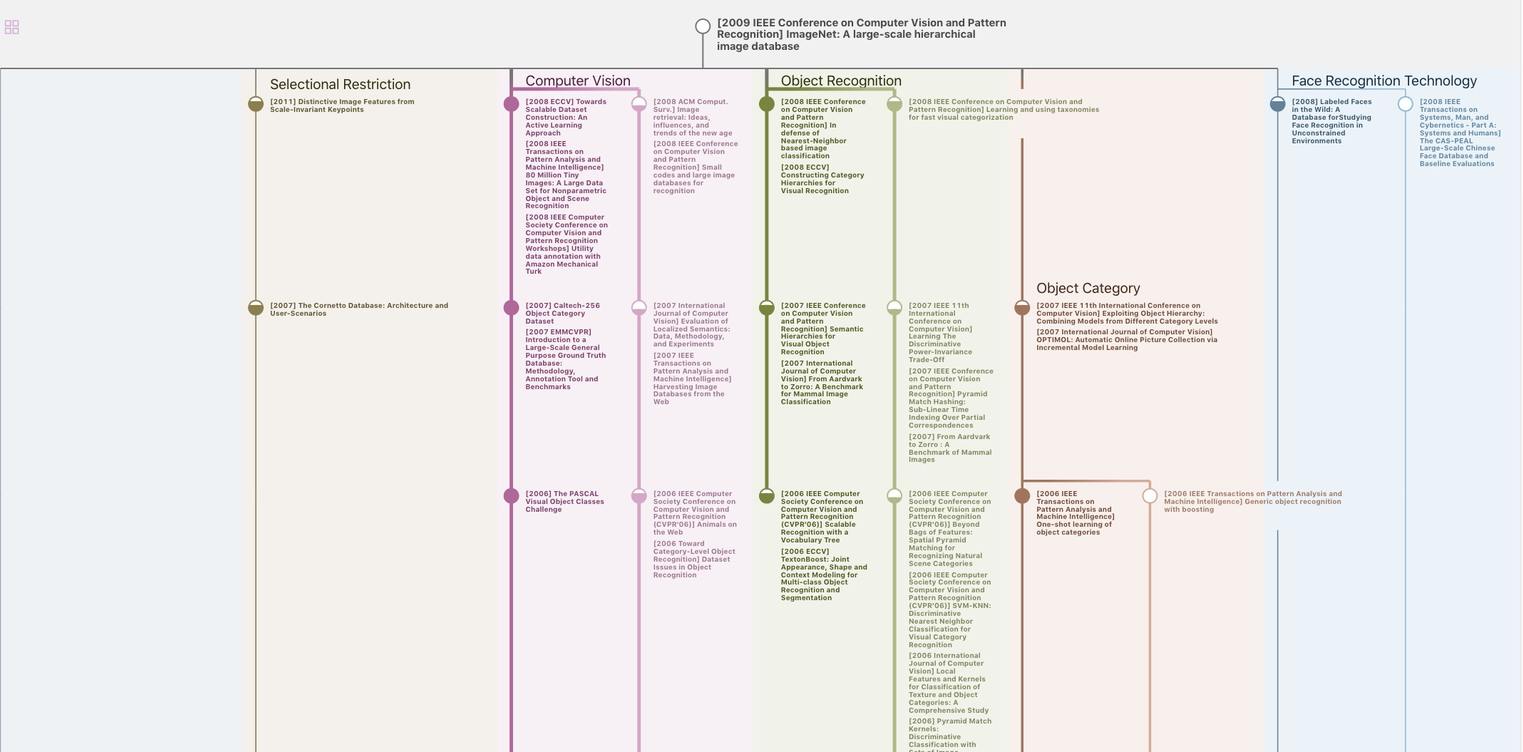
生成溯源树,研究论文发展脉络
Chat Paper
正在生成论文摘要