'Just because you are right, doesn't mean I am wrong' - Overcoming a bottleneck in development and evaluation of Open-Ended VQA tasks.
EACL(2021)
Abstract
GQA (CITATION) is a dataset for real-world visual reasoning and compositional question answering. We found that many answers predicted by the best vision-language models on the GQA dataset do not match the ground-truth answer but still are semantically meaningful and correct in the given context. In fact, this is the case with most existing visual question answering (VQA) datasets where they assume only one ground-truth answer for each question. We propose Alternative Answer Sets (AAS) of ground-truth answers to address this limitation, which is created automatically using off-the-shelf NLP tools. We introduce a semantic metric based on AAS and modify top VQA solvers to support multiple plausible answers for a question. We implement this approach on the GQA dataset and show the performance improvements.
MoreTranslated text
Key words
development,evaluation,open-ended
AI Read Science
Must-Reading Tree
Example
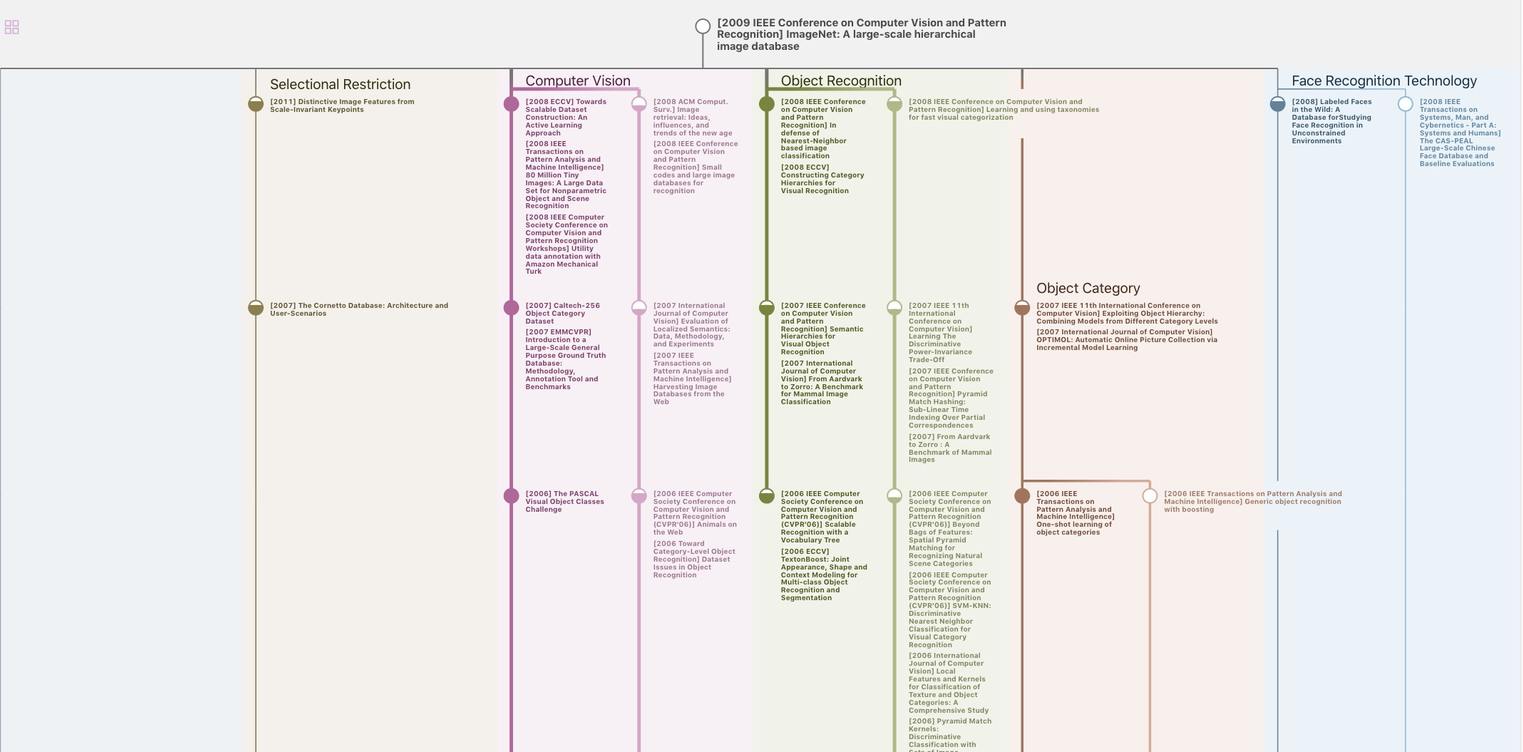
Generate MRT to find the research sequence of this paper
Chat Paper
Summary is being generated by the instructions you defined