Analyzing the Forgetting Problem in Pretram-Finetuning of Open-domain Dialogue Response Models
EACL(2021)
摘要
In this work, we study how the finetuning stage in the pretrain-finetune framework changes the behavior of a pretrained neural language generator. We focus on the transformer encoder-decoder model for the open-domain dialogue response generation task. Our major finding is that after standard finetuning, the model forgets some of the important language generation skills acquired during large-scale pretraining. We demonstrate the forgetting phenomenon through a set of detailed behavior analysis from the perspectives of knowledge transfer, context sensitivity, and function space projection. As a preliminary attempt to alleviate the forgetting problem, we propose an intuitive finetuning strategy named mix-review. We find that mix-review effectively regularizes the finetuning process, and the forgetting problem is alleviated to some extent. Finally, we discuss interesting behavior of the resulting dialogue model and its implications.
更多查看译文
AI 理解论文
溯源树
样例
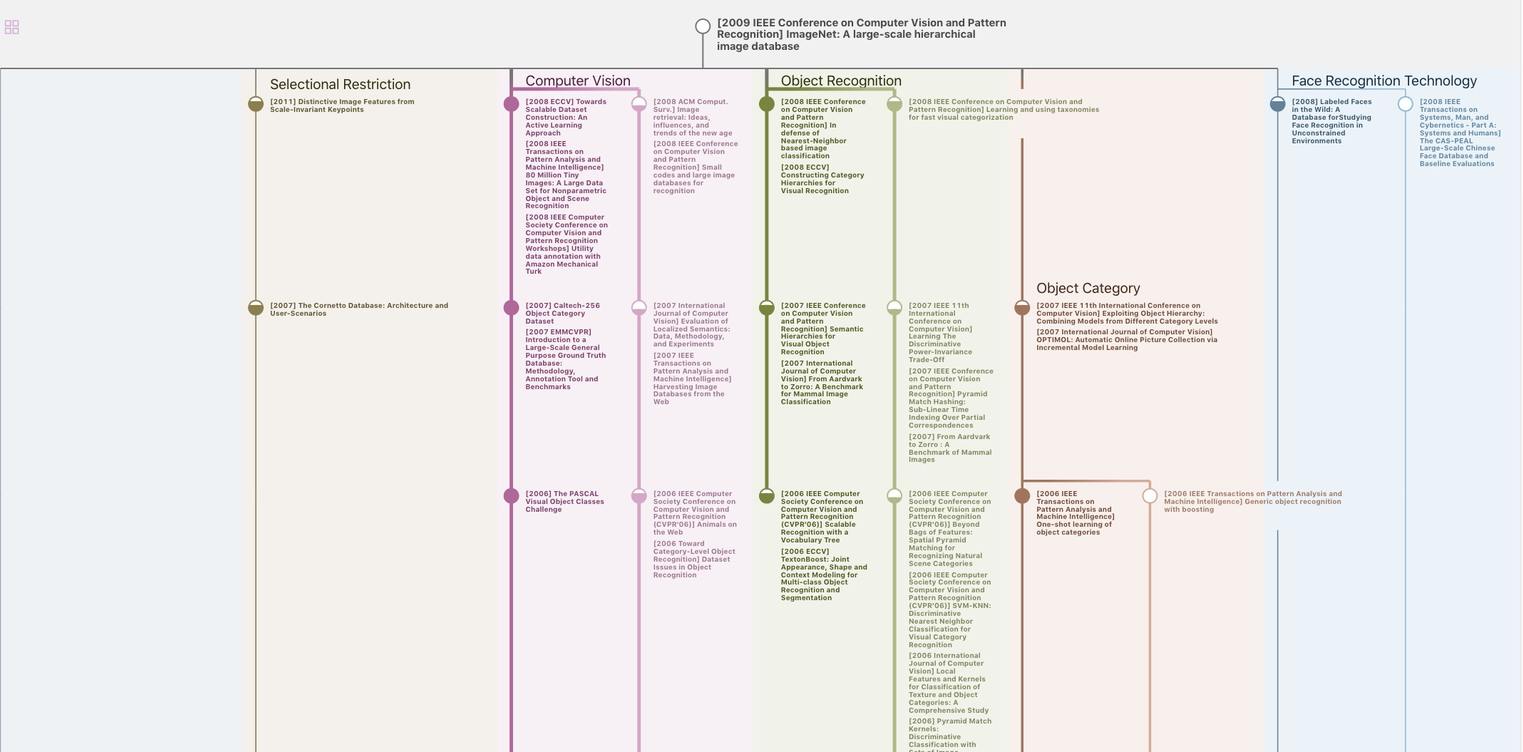
生成溯源树,研究论文发展脉络
Chat Paper
正在生成论文摘要