Machine Learning Versus Classical Electrocardiographic Criteria For Echocardiographic Left Ventricular Hypertrophy In A Pre-Participation Cohort
KARDIOLOGIA POLSKA(2021)
摘要
Background: Classical electrocardiographic (ECG) criteria for left ventricular hypertrophy (LVH) are well studied in older populations and patients with hypertension. Their utility in young pre-participation cohorts is unclear.Aims: We aimed to develop machine learning models for detection of echocardiogram-diagnosed LVH from ECG, and compare these models with classical criteria.Methods: Between November 2009 and December 2014, pre-participation screening ECG and subse- quent echocardiographic data was collected from 17310 males aged 16 to 23, who reported for medical screening prior to military conscription. A final diagnosis of LVH was made during echocardiography, defined by a left ventricular mass index >115 g/m(2). The continuous and threshold forms of classical ECG criteria (Sokolow-Lyon, Romhilt-Estes, Modified Cornell, Cornell Product, and Cornell) were compared against machine learning models (Logistic Regression, GLMNet, Random Forests, Gradient Boosting Machines) using receiver-operating characteristics curve analysis. We also compared the important variables identified by machine learning models with the input variables of classical criteria.Results: Prevalence of echocardiographic LVH in this population was 0.82% (143/17310). Classical ECG criteria had poor performance in predicting LVH. Machine learning methods achieved superior performance: Logistic Regression (area under the curve [AUC], 0.811; 95% confidence interval [CI], 0.738-0.884), GLMNet (AUC, 0.873; 95% CI, 0.817-0.929), Random Forest (AUC, 0.824; 95% CI, 0.749-0.898), Gradient Boosting Machines (AUC, 0.800; 95% CI, 0.738-0.862).Conclusions: Machine learning methods are superior to classical ECG criteria in diagnosing echocardiographic LVH in the context of pre-participation screening.
更多查看译文
关键词
biostatistics, electrocardiography, electronic medical records, myocardial disease
AI 理解论文
溯源树
样例
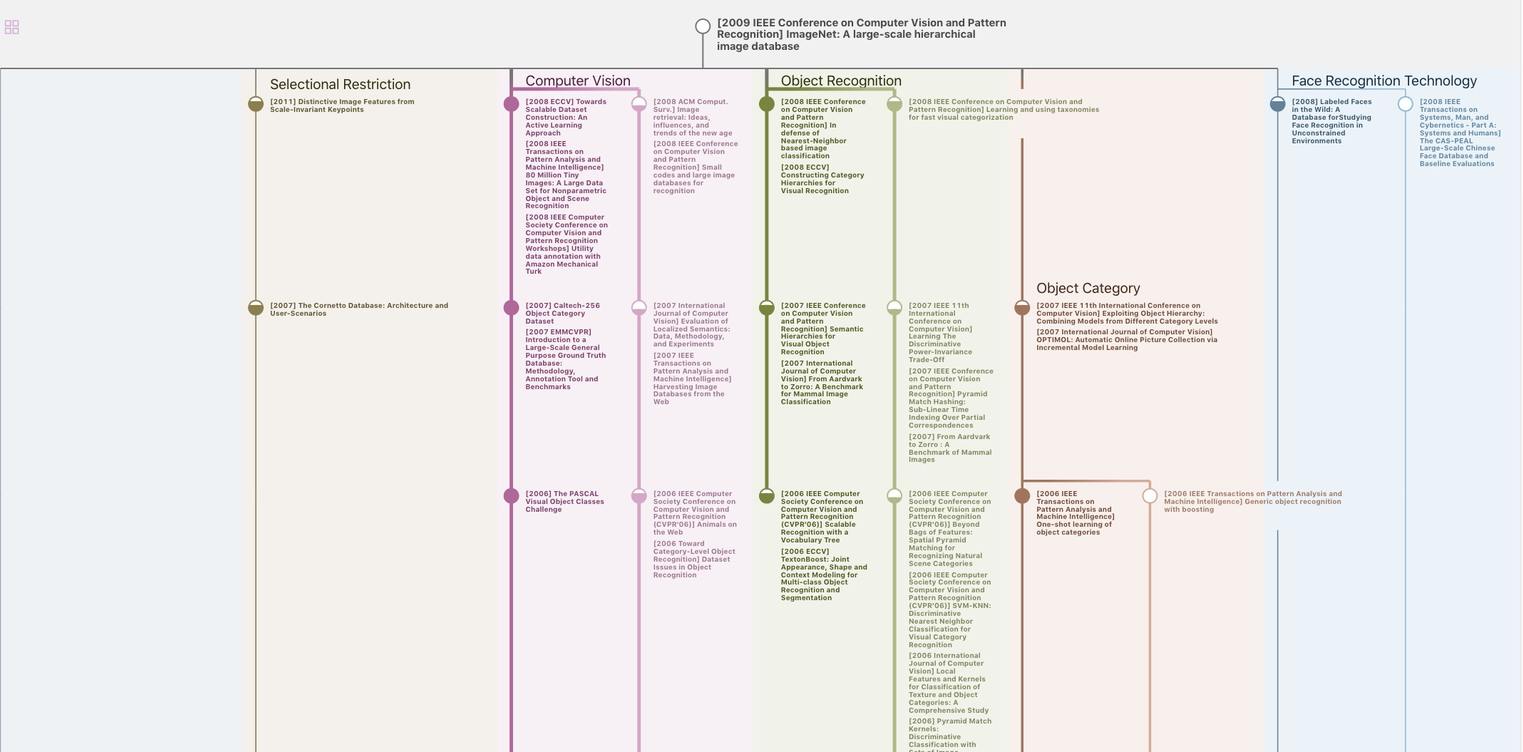
生成溯源树,研究论文发展脉络
Chat Paper
正在生成论文摘要