Sage: Practical and Scalable ML-Driven Performance Debugging in Microservices
ASPLOS(2021)
Abstract
Cloud applications are increasingly shifting from large monolithic services to complex graphs of loosely-coupled microservices. Despite the advantages of modularity and elasticity microservices offer, they also complicate cluster management and performance debugging, as dependencies between tiers introduce backpressure and cascading QoS violations. Prior work on performance debugging for cloud services either relies on empirical techniques, or uses supervised learning to diagnose the root causes of performance issues, which requires significant application instrumentation, and is difficult to deploy in practice. We present Sage, a machine learning-driven root cause analysis system for interactive cloud microservices that focuses on practicality and scalability. Sage leverages unsupervised ML models to circumvent the overhead of trace labeling, captures the impact of dependencies between microservices to determine the root cause of unpredictable performance online, and applies corrective actions to recover a cloud service's QoS. In experiments on both dedicated local clusters and large clusters on Google Compute Engine we show that Sage consistently achieves over 93% accuracy in correctly identifying the root cause of QoS violations, and improves performance predictability.
MoreTranslated text
Key words
cloud computing,microservices,performance debugging,QoS,counterfactual,Bayesian network,variational autoencoder
AI Read Science
Must-Reading Tree
Example
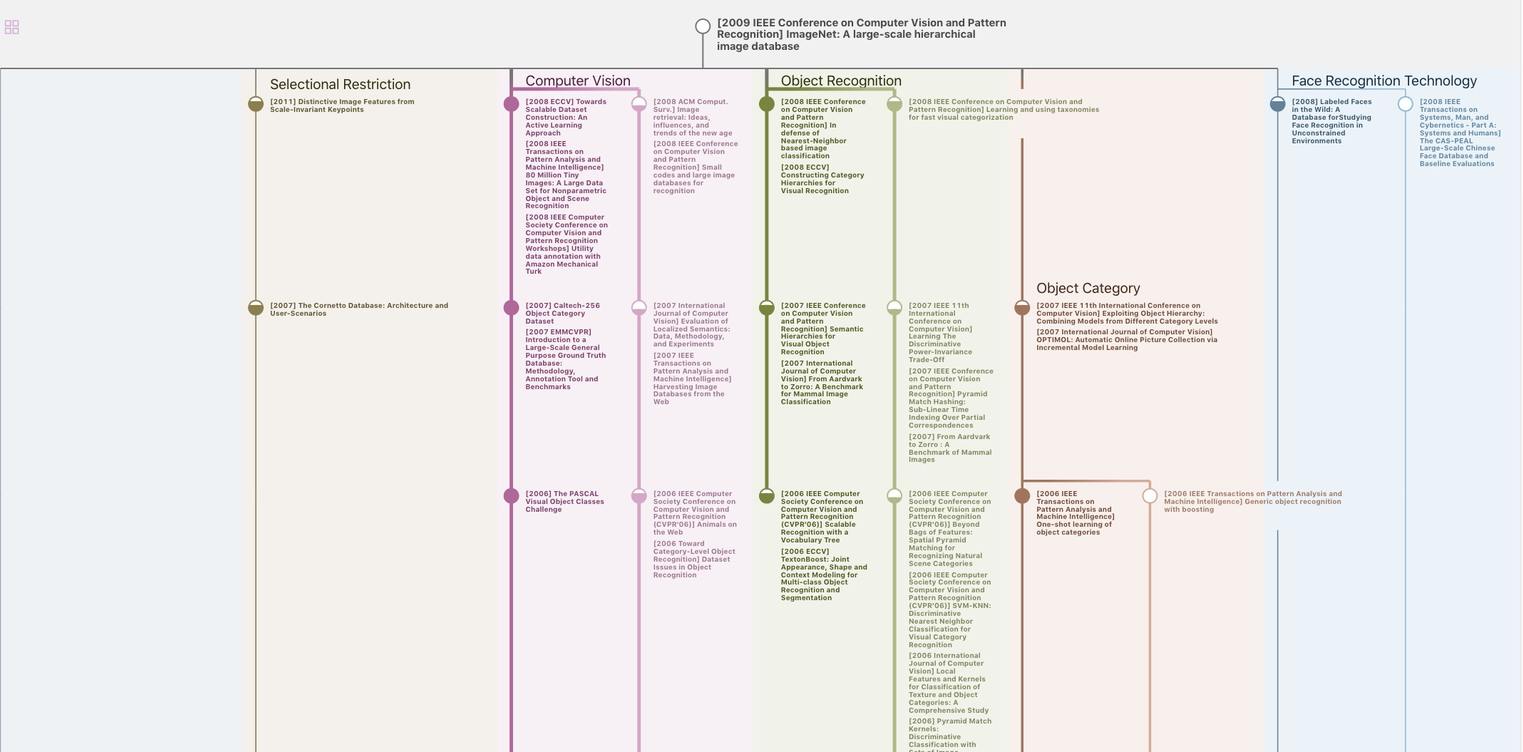
Generate MRT to find the research sequence of this paper
Chat Paper
Summary is being generated by the instructions you defined