Target-Style-Aware Unsupervised Domain Adaptation For Object Detection
IEEE ROBOTICS AND AUTOMATION LETTERS(2021)
摘要
Vision modules running on mobility platforms, such as robots and cars, often face challenging situations such as a domain shift where the distributions of training (source) data and test (target) data are different. The domain shift is caused by several variation factors, such as style, camera viewpoint, object appearance, object size, backgrounds, and scene layout. In this work, we propose an object detection training framework for unsupervised domain-style adaptation. The proposed training framework transfers target-style information to source samples and simultaneously trains the detection network with these target-stylized source samples in an end-to-end manner. The detection network can learn the target domain from the target-stylized source samples. The style is extracted from object areas obtained by using pseudo-labels to reflect the style of the object areas more than that of the irrelevant backgrounds. We empirically verified that the proposed methods improve detection accuracy in diverse domain shift scenarios using the Cityscapes, FoggyCityscapes, Sim10k, BDD100k, PASCAL, and Watercolor datasets.
更多查看译文
关键词
Deep learning for visual perception, object detection, segmentation and categorization, transfer learning
AI 理解论文
溯源树
样例
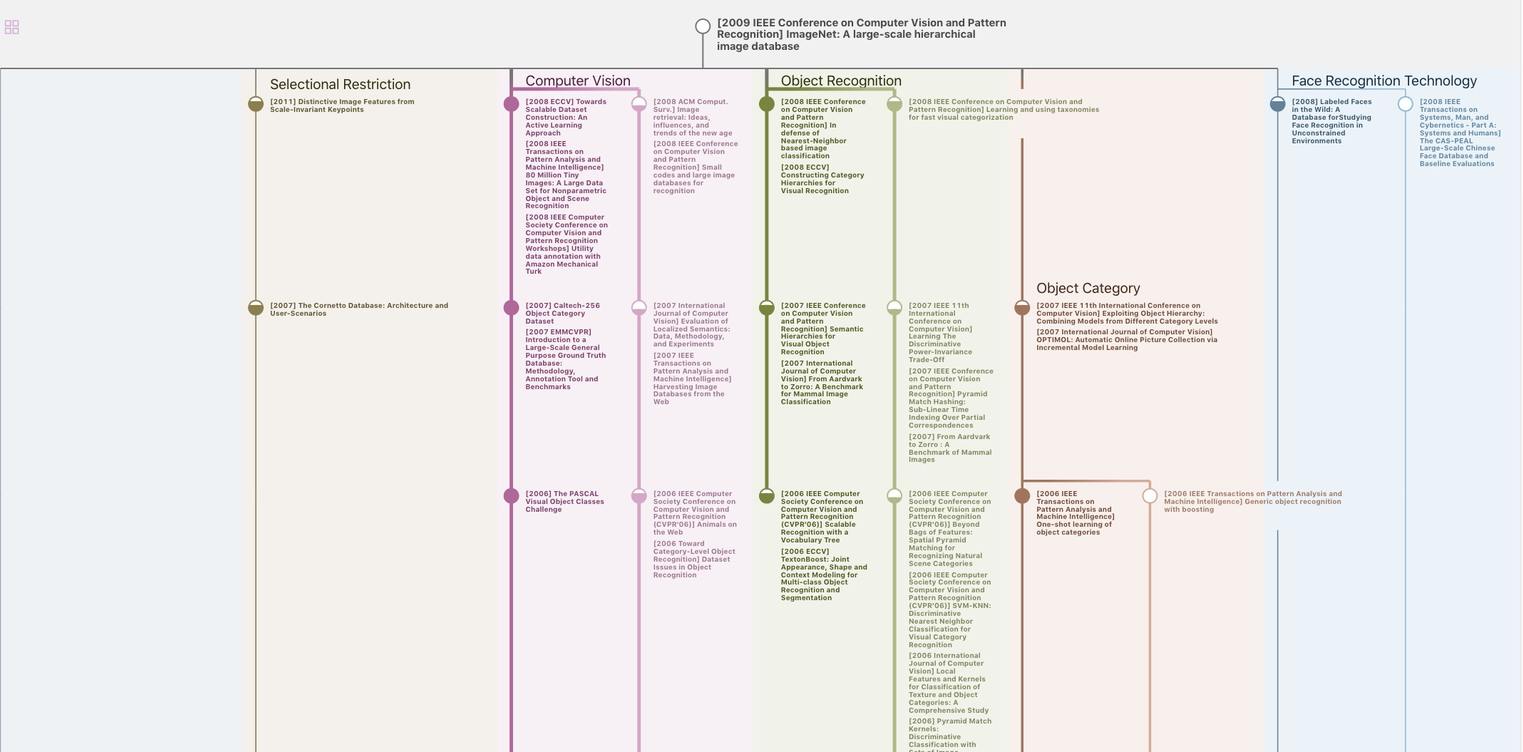
生成溯源树,研究论文发展脉络
Chat Paper
正在生成论文摘要